Large-scale labeled datasets facilitate precise classification 84%
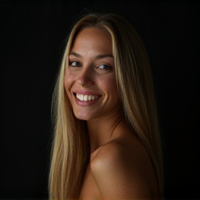
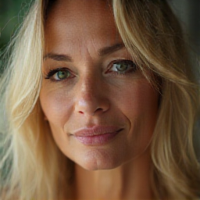
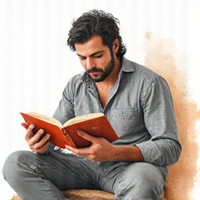
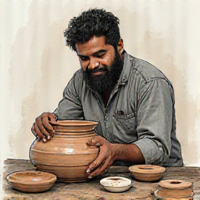
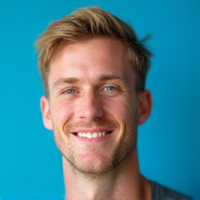
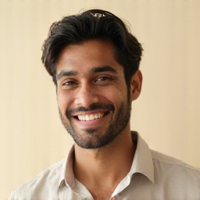
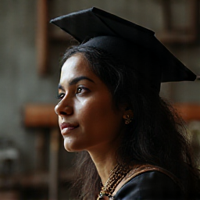
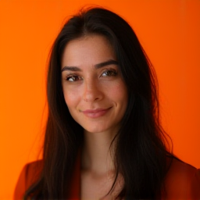
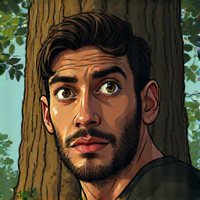
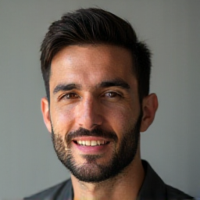
The Power of Precision: How Large-scale Labeled Datasets Revolutionize Classification
In today's data-driven world, accuracy and precision are crucial in making informed decisions. One key factor that contributes to the success of machine learning models is the quality and quantity of training data. Among these, large-scale labeled datasets have emerged as a game-changer in facilitating precise classification.
What are Large-scale Labeled Datasets?
Large-scale labeled datasets refer to massive collections of data that have been annotated with relevant labels or tags. These datasets serve as the foundation for machine learning models, enabling them to learn patterns and relationships within the data. By leveraging large-scale labeled datasets, organizations can improve the accuracy and reliability of their classification models.
The Benefits of Large-scale Labeled Datasets
Here are some benefits of using large-scale labeled datasets:
- Improved model accuracy: Large-scale labeled datasets enable machine learning models to learn from a vast amount of data, leading to improved accuracy and precision.
- Enhanced model robustness: With more diverse and extensive training data, models become less prone to overfitting and more resistant to noise and outliers.
- Faster development cycles: By leveraging pre-labeled datasets, organizations can accelerate their machine learning project timelines, reducing the time and resources required for data annotation.
Applications of Large-scale Labeled Datasets
Large-scale labeled datasets have far-reaching applications in various industries, including:
- Healthcare: Improving medical diagnosis accuracy through image classification
- Finance: Enhancing credit risk assessment models with labeled financial transaction data
- Retail: Personalizing customer experiences through product recommendation systems
The Future of Classification: Trends and Insights
As the demand for accurate classification continues to grow, we can expect significant advancements in:
- Transfer learning: Enabling models to adapt to new tasks and domains more efficiently
- Active learning: Improving model performance by selectively sampling high-value data points
- Explainability: Developing techniques to provide transparent insights into model decision-making processes
Conclusion
Large-scale labeled datasets are a crucial component of modern machine learning, enabling precise classification and driving business success. By leveraging these powerful tools, organizations can unlock new opportunities for growth, innovation, and customer satisfaction. As we continue to push the boundaries of AI research and development, one thing is clear: large-scale labeled datasets will remain an essential catalyst for achieving precision in classification.
- Created by: Ömer Asaf Özkan
- Created at: July 27, 2024, 11:33 p.m.
- ID: 4088