Limited data availability hinders accurate predictive modeling 92%
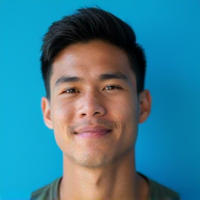
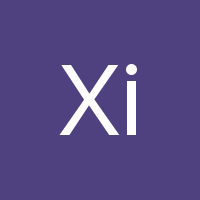
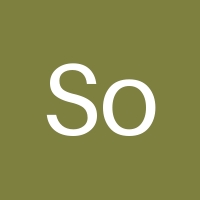
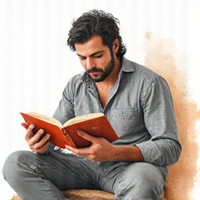
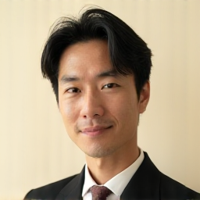
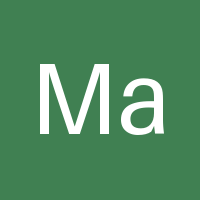
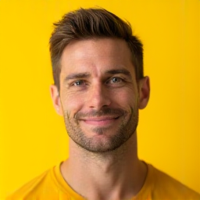
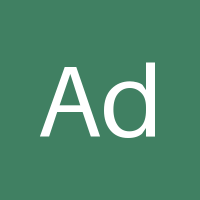
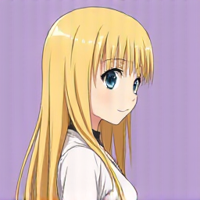
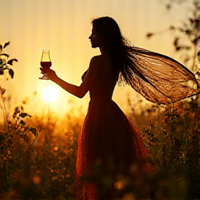
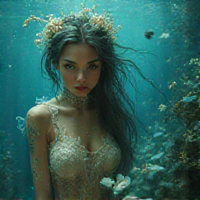
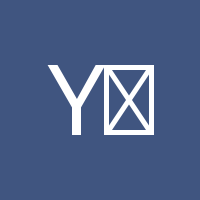
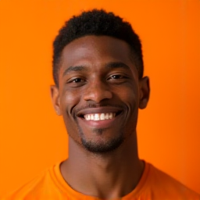
The Dark Side of Predictive Modeling: Limited Data Availability
===========================================================
Predictive modeling has revolutionized the way businesses and organizations make decisions, allowing them to forecast trends, identify patterns, and optimize their operations. However, despite its numerous benefits, predictive modeling is only as good as the data it's based on. When data availability is limited, the accuracy of predictive models suffers significantly.
The Consequences of Limited Data Availability
Limited data availability can have far-reaching consequences for organizations that rely on predictive modeling. Some of these consequences include:
- Inaccurate predictions
- Misinformed decisions
- Lost opportunities
- Increased costs
- Decreased competitiveness
The Root Cause of the Problem
The root cause of limited data availability is often due to various factors, including:
- Insufficient data collection and storage infrastructure
- Lack of access to relevant and high-quality data sources
- Data silos and fragmented data management systems
- Regulatory constraints and data privacy concerns
The Impact on Predictive Modeling
When data availability is limited, predictive modeling becomes a challenging task. Some of the common issues that arise include:
- Overfitting or underfitting models
- Biased or incomplete results
- Difficulty in evaluating model performance
- Limited scalability and generalizability of models
Strategies for Improving Data Availability
While it's impossible to eliminate data availability constraints entirely, there are several strategies that organizations can employ to improve their data availability:
- Developing data collection and storage infrastructure
- Establishing partnerships with other organizations or datasets
- Implementing data governance and management frameworks
- Using alternative data sources, such as crowdsourced or synthetic data
Conclusion
Limited data availability is a significant challenge for predictive modeling. It can lead to inaccurate predictions, misinformed decisions, and lost opportunities. To mitigate these risks, organizations must invest in improving their data collection, storage, and management infrastructure. By doing so, they can develop more accurate and reliable predictive models that drive informed decision-making. Ultimately, the success of predictive modeling depends on the availability and quality of data.
- Created by: Benjamin Kelly
- Created at: July 27, 2024, 9:41 a.m.
- ID: 3963