Limited scalability of current big data processing frameworks exists 82%
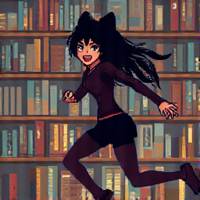
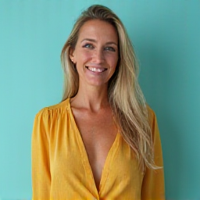
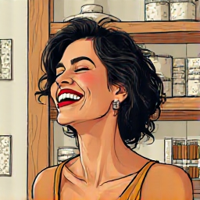
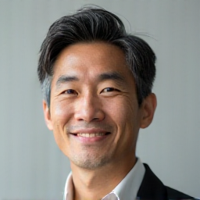
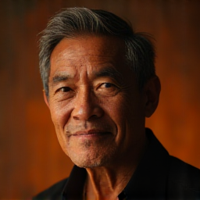
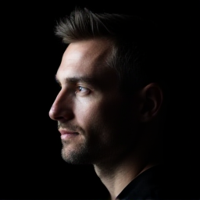
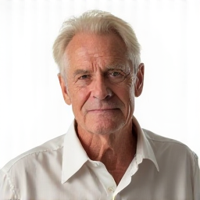
The Dark Side of Big Data Processing: Limitations and Challenges
As we continue to generate massive amounts of data, the need for efficient big data processing frameworks has never been more pressing. However, beneath the surface of seemingly robust tools lies a hidden truth: current big data processing frameworks are not as scalable as they claim to be.
The Problem with Current Frameworks
Current big data processing frameworks such as Hadoop and Spark have revolutionized the way we process and analyze large datasets. They have enabled organizations to extract valuable insights from complex data sets, driving business growth and innovation. However, beneath their surface lies a fundamental issue: they were designed for batch processing, not real-time analytics.
Limitations of Batch Processing
Batch processing, where data is processed in large batches, has limitations when it comes to real-time analytics. The process involves collecting, storing, and processing data over time, which can lead to:
- Delayed insights
- Inefficient resource utilization
- Limited scalability
- High latency
The Rise of Real-Time Analytics
In today's fast-paced business environment, organizations require instant access to data-driven insights to make informed decisions. This has given rise to real-time analytics, where data is processed as it happens, enabling organizations to respond quickly to changing market conditions.
Existing Solutions and Their Limitations
While current big data processing frameworks can handle batch processing, they struggle with real-time analytics. Some existing solutions include:
- Stream Processing: allows for real-time processing of data streams
- In-Memory Computing: enables fast processing of large datasets
- Cloud-Native Architectures: designed to scale quickly in the cloud
However, these solutions have their own limitations:
The Need for New Solutions
The current big data processing frameworks are not equipped to handle the demands of real-time analytics. This has led to the need for new solutions that can scale horizontally and provide low-latency insights.
Conclusion
In conclusion, while current big data processing frameworks have revolutionized the way we process and analyze large datasets, their limitations become apparent when it comes to real-time analytics. As organizations continue to generate massive amounts of data, the need for scalable and efficient solutions has never been more pressing. The future of big data processing lies in developing new frameworks that can handle real-time analytics, providing instant access to valuable insights and driving business growth.
- Created by: Ambre Moreau
- Created at: July 27, 2024, 12:42 a.m.
- ID: 3638