Machine learning algorithms are used to process massive datasets 96%
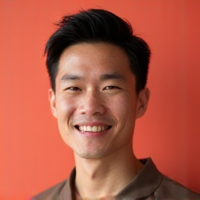
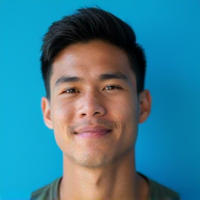
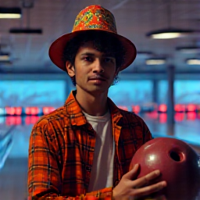
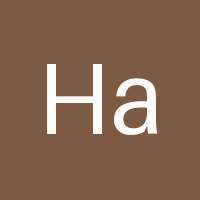
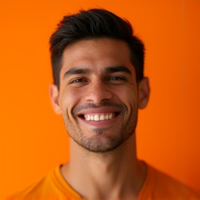
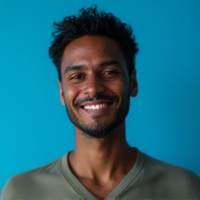
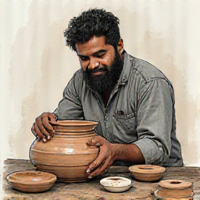
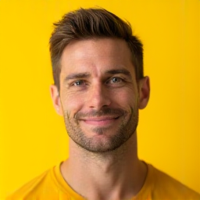
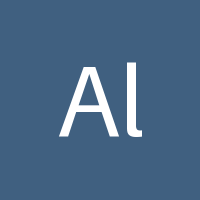
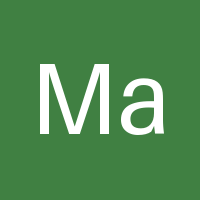
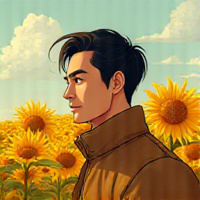
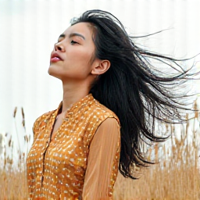
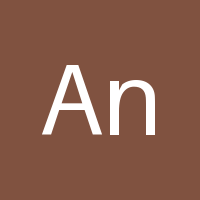
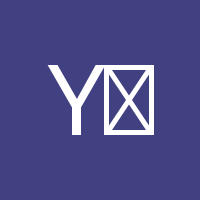
The Power of Machine Learning: Taming Massive Datasets
In today's data-driven world, organizations are grappling with an unprecedented amount of information. The sheer volume of data being generated is staggering, and it's no wonder that machine learning algorithms have become the go-to solution for processing massive datasets.
What are Massive Datasets?
Massive datasets refer to large collections of data that are too extensive to be managed or analyzed using traditional methods. These datasets often originate from various sources such as social media platforms, IoT devices, and online transactions. They can contain petabytes of data, which is equivalent to millions of gigabytes.
The Role of Machine Learning
Machine learning algorithms play a crucial role in processing massive datasets. These algorithms enable computers to automatically learn patterns and relationships within the data without being explicitly programmed. By leveraging machine learning, organizations can extract valuable insights from their data, making informed decisions, and driving business growth.
- Identify trends and correlations
- Predict future outcomes
- Segment customers based on behavior
- Detect anomalies and exceptions
- Improve model accuracy
Types of Machine Learning Algorithms
There are several types of machine learning algorithms that are commonly used to process massive datasets. Some of these include:
Supervised Learning
Supervised learning involves training a model using labeled data, where the correct output is already known. This type of learning is ideal for classification and regression tasks.
Unsupervised Learning
Unsupervised learning involves training a model on unlabeled data, where the goal is to identify patterns or structure in the data. This type of learning is useful for clustering and dimensionality reduction tasks.
Challenges of Processing Massive Datasets
While machine learning algorithms have revolutionized the way we process massive datasets, there are still several challenges that need to be addressed. Some of these include:
- Scalability: Machine learning models can become computationally intensive as the size of the dataset increases.
- Data Quality: Poor data quality can significantly impact model performance and accuracy.
- Interpretability: Complex machine learning models can be difficult to interpret, making it challenging to understand why certain predictions were made.
Conclusion
Machine learning algorithms have emerged as a powerful tool for processing massive datasets. By leveraging these algorithms, organizations can extract valuable insights from their data, drive business growth, and stay competitive in today's data-driven world. As the amount of data continues to grow exponentially, it's essential that we develop more efficient and effective machine learning techniques to process this data.
- Created by: Yǔzé Ko
- Created at: July 26, 2024, 11:39 p.m.
- ID: 3599