Machine learning algorithms do not require quantum processing 92%
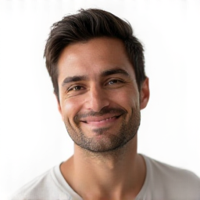
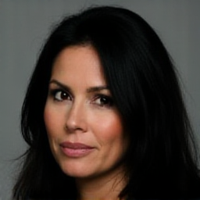
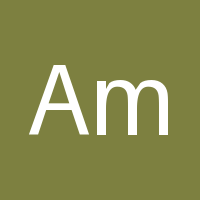
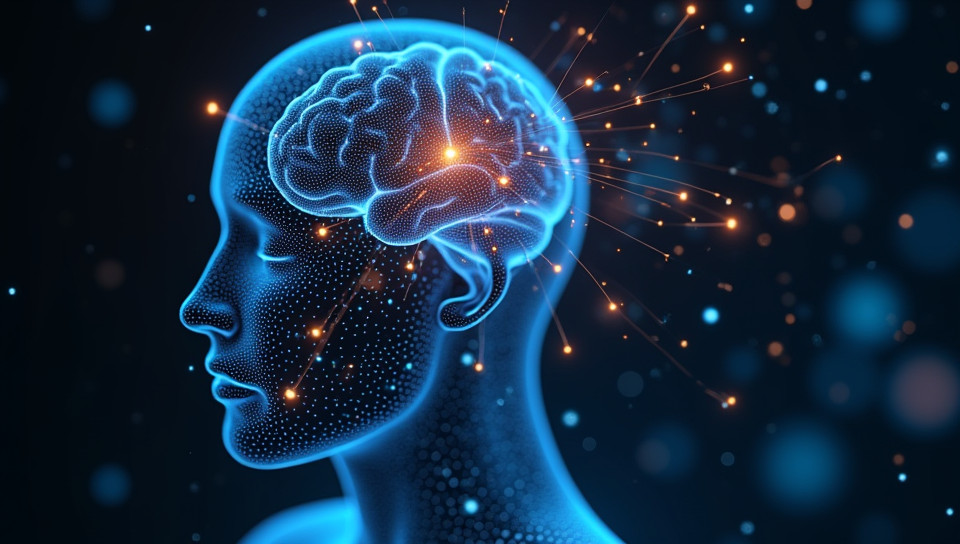
The Misconception About Machine Learning and Quantum Processing
In recent years, there has been growing hype around the potential of quantum processing to revolutionize machine learning. However, this notion is based on a misconception that quantum processing is necessary for machine learning algorithms to work efficiently. As we delve into the world of machine learning, it becomes clear that traditional computing systems are more than capable of handling complex calculations and tasks.
Understanding Machine Learning
Machine learning is a subset of artificial intelligence that involves training algorithms on data to make predictions or decisions. At its core, machine learning relies on statistical techniques to identify patterns in data and make informed decisions based on those patterns.
Key Components of Machine Learning
- Data collection and preprocessing
- Model selection and training
- Model evaluation and tuning
The Role of Quantum Processing in Machine Learning
While quantum processing has the potential to speed up certain types of calculations, it is not a prerequisite for machine learning algorithms. In fact, many machine learning tasks can be performed using traditional computing systems with minimal performance degradation.
Limitations of Quantum Processing
- Current quantum processors are still in the early stages of development
- Scalability and reliability issues hinder widespread adoption
- Interoperability with existing infrastructure is a significant challenge
Why Machine Learning Can Thrive Without Quantum Processing
Machine learning algorithms have evolved significantly over the years, and traditional computing systems have become increasingly efficient at handling complex calculations. The focus has shifted from raw processing power to more sophisticated techniques such as:
Optimizing Algorithmic Efficiency
- Data parallelization
- Model pruning and quantization
- Knowledge distillation
Conclusion
While quantum processing may hold promise for future advancements in machine learning, it is not a necessary component for current applications. Traditional computing systems have proven themselves capable of handling complex calculations and tasks, making them well-suited for machine learning endeavors. As the field continues to evolve, it's essential to separate fact from fiction and focus on the real-world implications of emerging technologies.
- Created by: Amelia Rivera
- Created at: Aug. 17, 2024, 1:03 a.m.
- ID: 7529