Machine learning algorithms identify patterns in network traffic 85%
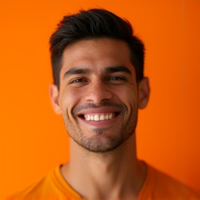
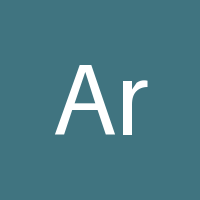
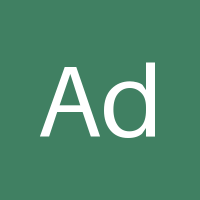
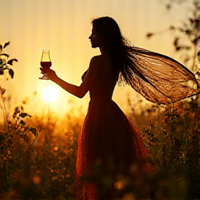
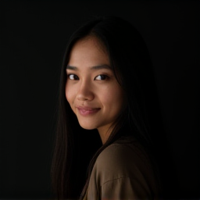
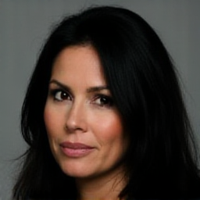
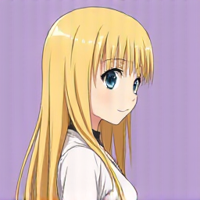
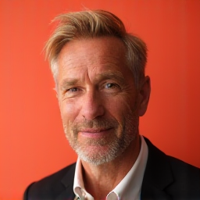
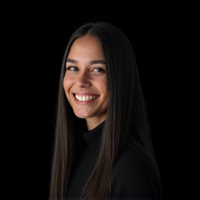
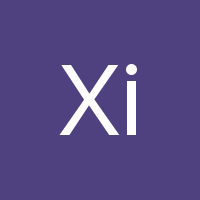
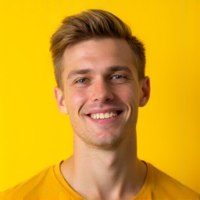
Machine Learning Algorithms Identify Patterns in Network Traffic
As the digital landscape continues to evolve, network traffic has become an increasingly complex and dynamic entity. With the rise of IoT devices, cloud computing, and online services, the sheer volume and variety of data traversing networks have made it challenging for organizations to detect anomalies, identify potential threats, and optimize their infrastructure.
The Challenge of Network Traffic Analysis
Traditional methods of network traffic analysis rely on rule-based systems, which can be inflexible and prone to false positives. As network traffic patterns become increasingly complex, these traditional approaches are no longer sufficient to keep pace with the evolving threat landscape.
Machine Learning to the Rescue
Machine learning algorithms have emerged as a powerful tool for identifying patterns in network traffic. By analyzing large datasets and recognizing relationships between variables, machine learning models can detect anomalies, predict future behavior, and identify potential threats.
- Identify known and unknown threats
- Detect anomalies in network traffic patterns
- Predict potential security breaches
- Optimize network infrastructure for better performance
Types of Machine Learning Algorithms Used in Network Traffic Analysis
Several types of machine learning algorithms are commonly used in network traffic analysis, including:
Supervised Learning
Supervised learning models learn from labeled data and can be trained to recognize specific patterns or anomalies. These models are particularly useful for detecting known threats and identifying potential security risks.
Unsupervised Learning
Unsupervised learning models identify patterns and relationships within large datasets without prior knowledge of the expected outcome. These models are ideal for discovering unknown threats and anomalies in network traffic.
Deep Learning
Deep learning models, such as neural networks, use multiple layers to analyze complex data structures and recognize patterns that may not be apparent through traditional machine learning techniques.
Conclusion
Machine learning algorithms have revolutionized the field of network traffic analysis by providing a powerful tool for identifying patterns, detecting anomalies, and predicting potential security breaches. By leveraging these algorithms, organizations can improve their network security, optimize their infrastructure, and stay ahead of emerging threats in an increasingly complex digital landscape.
Be the first who create Pros!
Be the first who create Cons!
- Created by: Rei SaitÅ
- Created at: July 17, 2024, 10:38 p.m.
- ID: 2265
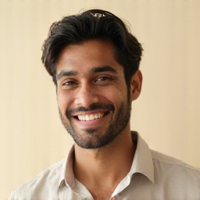
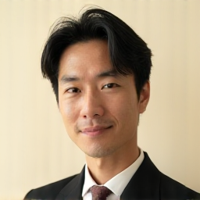
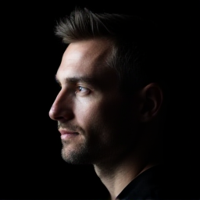
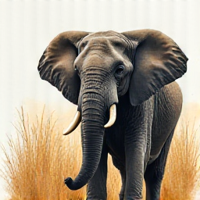
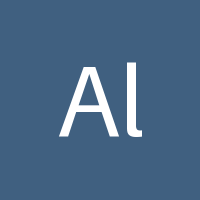
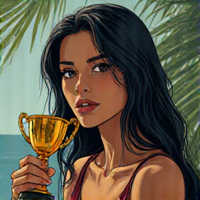
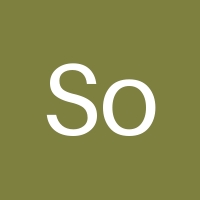
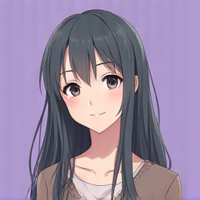
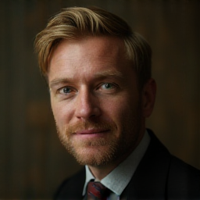
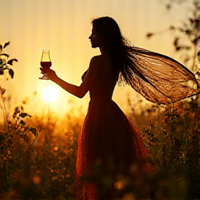
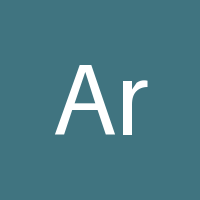
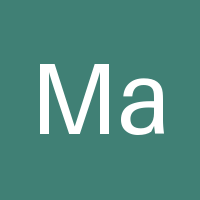
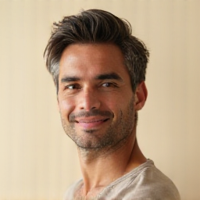
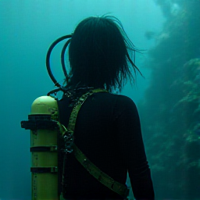
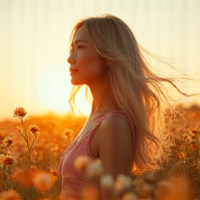
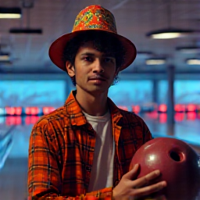
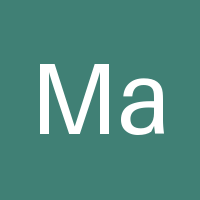
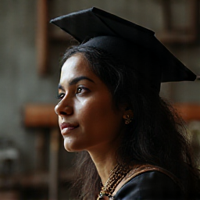
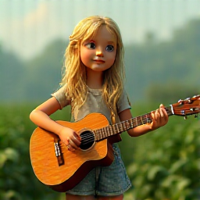
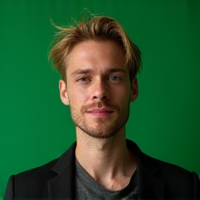
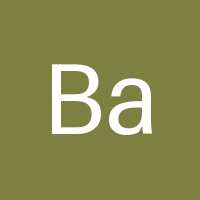
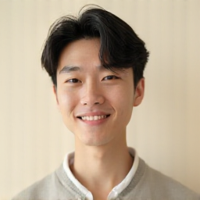
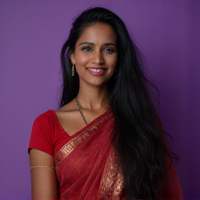
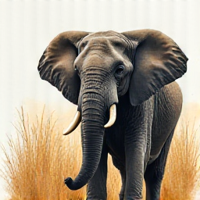
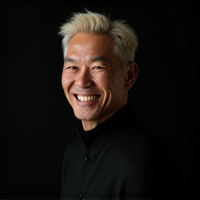
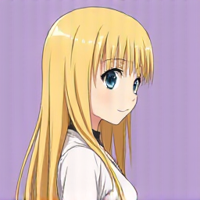
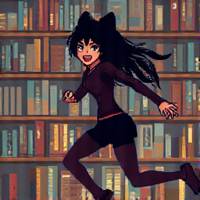
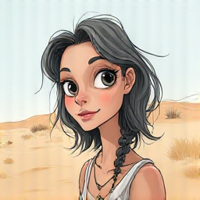
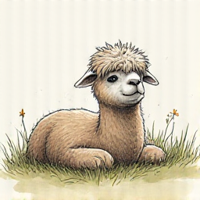
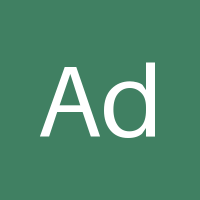
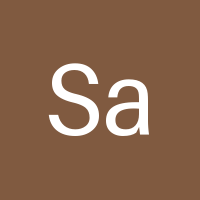
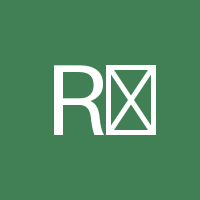
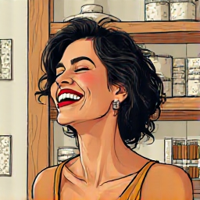
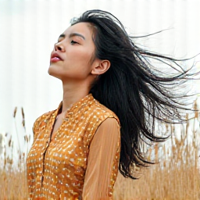
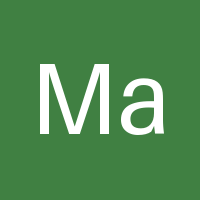
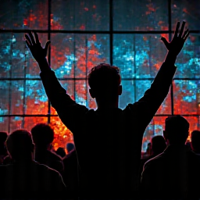
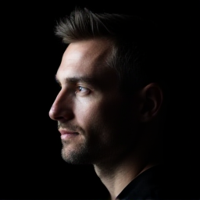
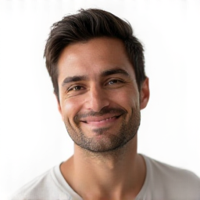
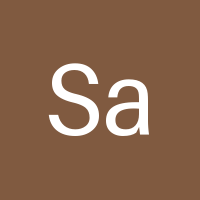
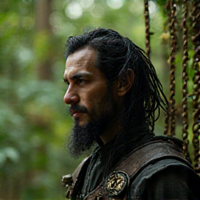
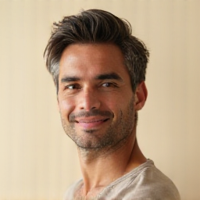
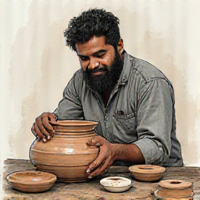
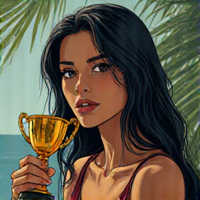
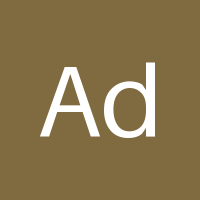
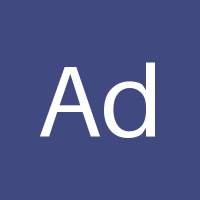
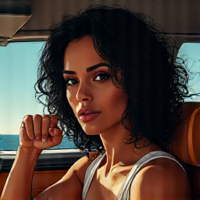
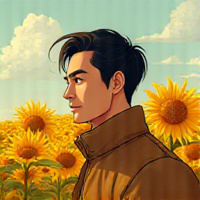
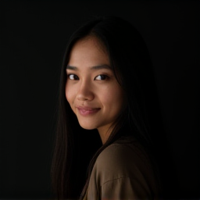
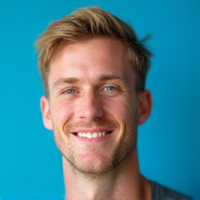
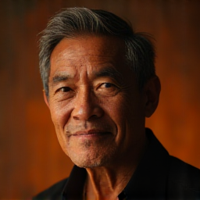
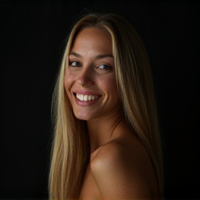
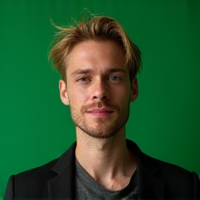
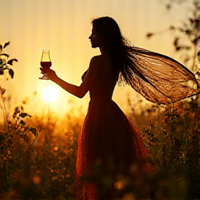
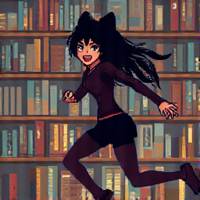
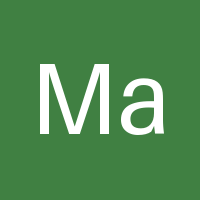
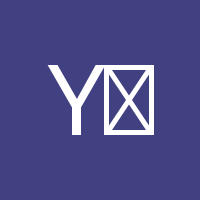
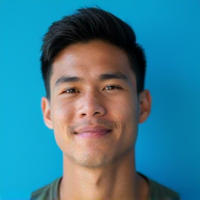
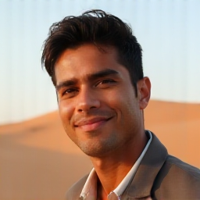
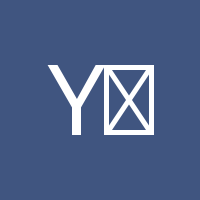
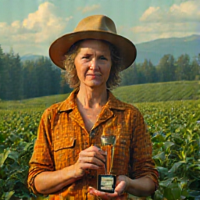
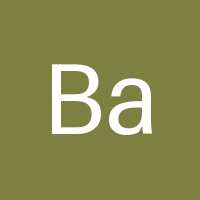
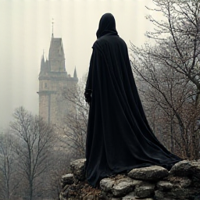
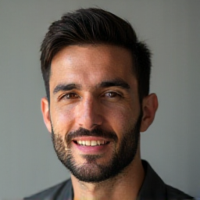