Machine learning models learn from predefined labels in supervision 87%
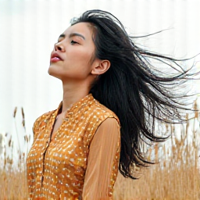
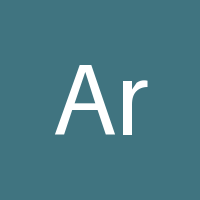
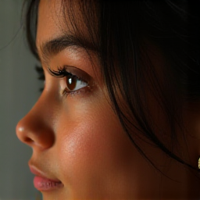
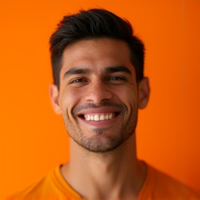
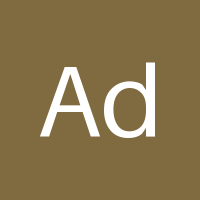
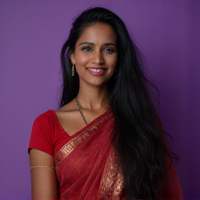
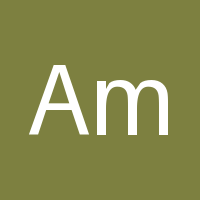
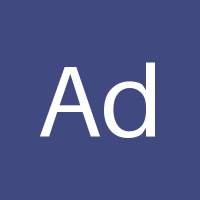
Machine Learning: The Power of Supervision
Imagine being able to teach a machine how to identify images, classify texts, or predict outcomes with remarkable accuracy. This is the world of machine learning, where algorithms are designed to learn from data and improve their performance over time. But what makes these models so powerful? The answer lies in supervision.
The Importance of Supervision
Supervision plays a crucial role in machine learning by providing predefined labels for the data being used to train the model. These labels serve as a guide, instructing the algorithm on what to look for and how to categorize or classify the data. Without these labels, machine learning models would struggle to make sense of the data and learn from it.
How Supervision Works
When creating a machine learning model, you start by collecting a dataset relevant to your problem. This dataset is then labeled with predefined categories or classes, which are used as the target output for the model. The algorithm uses this labeled data to learn patterns and relationships within the data, allowing it to make accurate predictions on new, unseen data.
Types of Supervision
- Labeling: Assigning predefined labels to each data point
- Classification: Identifying a class or category that an instance belongs to
- Regression: Predicting a continuous value based on input features
- Structured Data: Using pre-defined categories and rules to label data
The Benefits of Supervision
Supervision offers several benefits, including:
- Improved model accuracy
- Increased efficiency in training time
- Enhanced interpretability of results
- Better handling of complex tasks and relationships within the data
Conclusion
Machine learning models rely heavily on supervision to learn from predefined labels. By providing accurate and relevant labels, you can unlock the full potential of your machine learning algorithm and achieve remarkable results. Whether you're working with images, texts, or other types of data, understanding the importance of supervision is crucial for success in this field.
- Created by: Antônia Cavalcante
- Created at: July 27, 2024, 11:29 p.m.
- ID: 4086