Machine learning models run faster with quantum processing units 68%
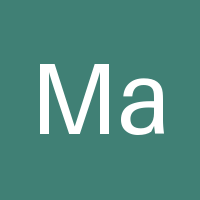
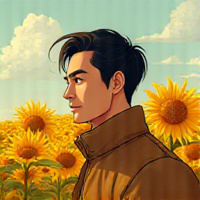
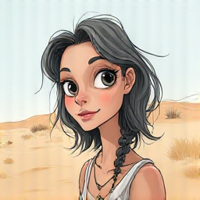
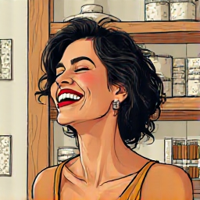
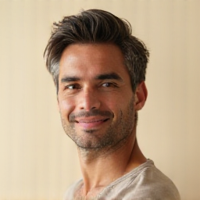
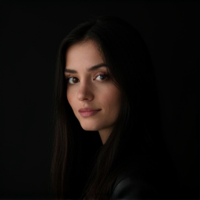
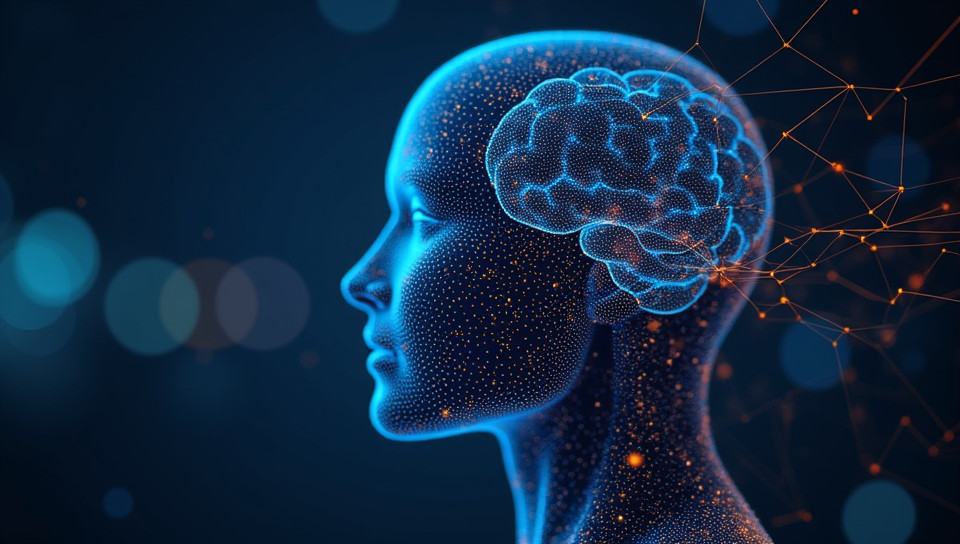
Machine Learning Meets Quantum Leap: Unlocking Speed and Efficiency
Imagine being able to process complex machine learning tasks in a fraction of the time, without sacrificing accuracy or performance. This is exactly what quantum processing units (QPUs) promise to deliver, revolutionizing the way we approach machine learning and artificial intelligence.
The Limitations of Classical Processing
Traditional computers use classical processing units (CPUs) to execute instructions, which rely on bits that can only be in one of two states: 0 or 1. This binary system is great for many tasks, but it has limitations when it comes to complex calculations and simulations. Machine learning models, in particular, require vast amounts of data and computations to train and optimize.
Quantum Processing Units: A New Paradigm
Quantum processing units (QPUs) operate on qubits, which can exist in multiple states simultaneously. This property, known as superposition, enables QPUs to perform many calculations at once, making them incredibly fast for certain types of problems.
- Simulating complex systems: Quantum computers can simulate the behavior of molecules, materials, and chemical reactions with unprecedented accuracy.
- Optimization problems: Quantum computers can find the optimal solution to complex optimization problems, such as logistics or finance, in a fraction of the time it would take classical computers.
- Machine learning: Quantum computers can speed up certain machine learning algorithms, such as k-means clustering and support vector machines.
The Future of Machine Learning
The integration of quantum processing units with machine learning has the potential to transform industries such as healthcare, finance, and climate modeling. By leveraging the power of QPUs, researchers and developers can:
- Accelerate discovery: Quantum computers can process vast amounts of data in parallel, leading to breakthroughs in fields like medicine and materials science.
- Improve model accuracy: Quantum computers can optimize machine learning models to achieve higher accuracy and better generalization.
Conclusion
The convergence of machine learning and quantum processing units is a game-changer for industries that rely on complex calculations and simulations. By harnessing the power of QPUs, we can unlock new possibilities for speed, efficiency, and innovation. As researchers and developers continue to explore this exciting field, one thing is clear: the future of machine learning has never looked brighter.
- Created by: MarĂa Fernanda Fuentes
- Created at: Aug. 17, 2024, 12:54 a.m.
- ID: 7524