Manual data labeling is too time-consuming for big data 70%
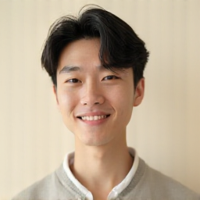
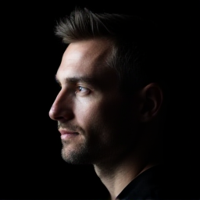
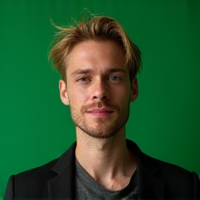
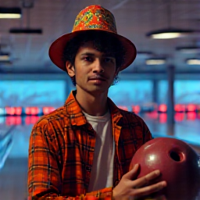
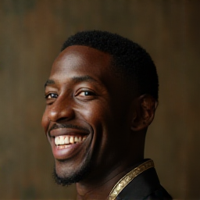
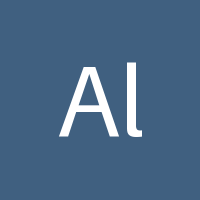
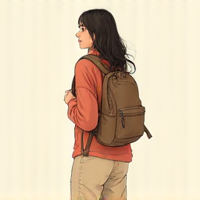
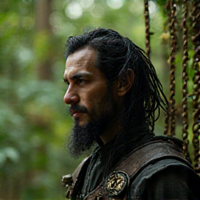
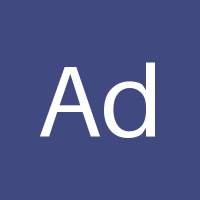
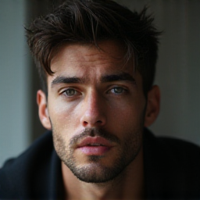
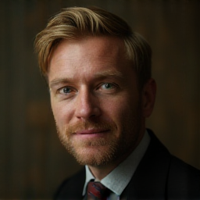
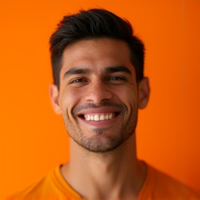
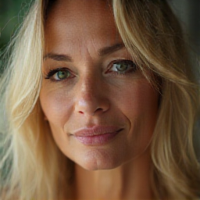
The Challenge of Big Data: Why Manual Labeling is No Longer Viable
In today's data-driven world, businesses are generating massive amounts of data every minute. This explosion in data has created new opportunities for organizations to gain insights and make informed decisions. However, with this increase in data comes the challenge of managing and labeling it. Manual data labeling, once a reliable method, is no longer sufficient for big data.
The Limitations of Manual Data Labeling
Manual data labeling involves human annotators assigning labels or tags to individual pieces of data. While effective for small datasets, this approach becomes impractical as dataset sizes grow. Here are some reasons why manual labeling falls short:
- Time-consuming: As dataset sizes increase, the time required to label each piece of data grows exponentially.
- Inefficient: Human annotators can only work on one task at a time, limiting the overall throughput and efficiency of the process.
- Expensive: With more human resources required for larger datasets, costs escalate quickly.
The Impact on Big Data
Big data is characterized by its massive size, complexity, and velocity. Manual labeling simply cannot keep up with these demands. As a result, organizations are facing significant delays in their analytics projects, which ultimately lead to missed business opportunities and revenue losses.
Automated Solutions: A Viable Alternative?
Fortunately, there are automated solutions available that can help alleviate the manual labeling burden. These tools use machine learning algorithms and natural language processing techniques to label data quickly and accurately. Some examples include:
- Active learning platforms that select the most informative samples for human annotation
- Transfer learning models that adapt to new datasets with minimal retraining
- Automated content moderation tools that detect and classify sensitive content
A New Era in Data Labeling
While automated solutions are not yet perfect, they offer a significant improvement over manual labeling. By leveraging these technologies, organizations can:
- Accelerate their analytics projects and get insights faster
- Reduce costs associated with human annotation
- Improve data quality and accuracy through more efficient sampling techniques
Conclusion
In conclusion, manual data labeling is no longer a viable solution for big data due to its time-consuming and inefficient nature. Automated solutions offer a promising alternative, enabling organizations to keep pace with the demands of big data. As technology continues to evolve, we can expect even more advanced tools that will further revolutionize the field of data labeling.
- Created by: Leon Kaczmarek
- Created at: July 27, 2024, 6:34 a.m.
- ID: 3859