MapReduce struggles to handle complex data structures 39%
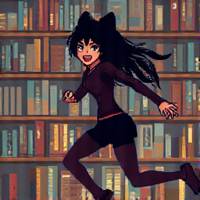
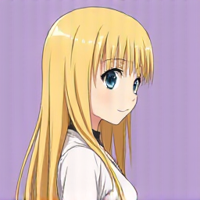
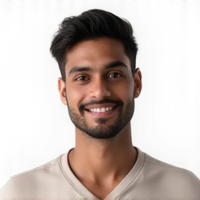
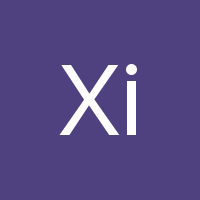
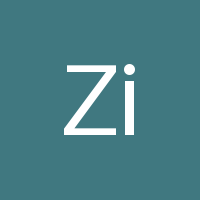
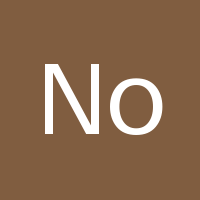
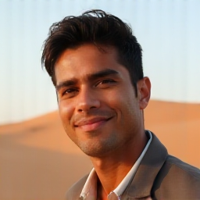
The Dark Side of Big Data: MapReduce's Struggle with Complex Structures
When it comes to processing and analyzing large datasets, MapReduce has been the go-to technology for many organizations. Its ability to distribute data across a cluster of machines, process it in parallel, and store the results efficiently has made it an indispensable tool in the big data arsenal. However, as we delve deeper into the world of complex data structures, we begin to realize that MapReduce's limitations are more pronounced than ever.
The Limitations of MapReduce
MapReduce is a batch-oriented processing framework, designed to handle large amounts of data that fit into a specific format - key-value pairs. It excels in scenarios where the data can be easily partitioned and processed independently by each mapper or reducer task. However, as soon as we introduce complex data structures like graphs, trees, or nested objects, MapReduce struggles to keep up.
The Challenges of Complex Data Structures
- Handling recursive relationships between data elements
- Processing large datasets with irregularly structured data
- Storing and retrieving hierarchical data efficiently
- Dealing with sparse or missing data in large datasets
- Managing data that has a complex schema or is constantly evolving
In these scenarios, MapReduce's batch-oriented processing model becomes a bottleneck. The framework requires significant amounts of memory to store intermediate results, which can lead to performance issues when dealing with large datasets. Moreover, the fixed-size blocks used by HDFS (Hadoop Distributed File System) make it challenging to handle variable-length data structures.
Alternatives and Workarounds
While MapReduce has its limitations, there are alternative frameworks and techniques that can help alleviate these challenges:
- Apache Spark: A more modern and flexible processing engine that can handle complex data structures like graphs and trees.
- Graph Processing Frameworks: Specialized frameworks like Apache Giraph or GraphLab that are designed to handle large-scale graph data.
- Data Lakes: Central repositories for storing raw, unprocessed data in its native format, which can be easily queried and processed using specialized tools.
Conclusion
MapReduce has been a pioneering technology in the world of big data processing. However, as we push the boundaries of complexity, it's essential to recognize its limitations and explore alternative solutions that can handle complex data structures more efficiently. By understanding these challenges and adopting the right technologies, we can unlock new insights from our data and drive business growth in today's fast-paced digital landscape.
Be the first who create Pros!
Be the first who create Cons!
- Created by: Dhruv Kumar
- Created at: July 27, 2024, 2:43 a.m.
- ID: 3715
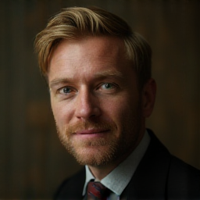
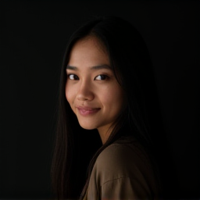
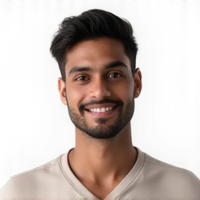
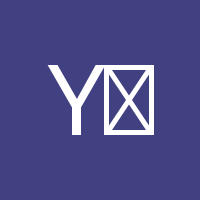
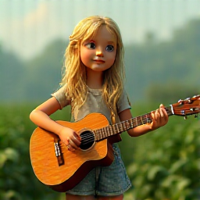
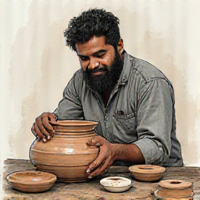
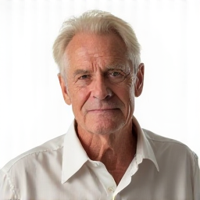
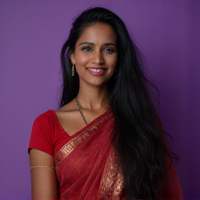
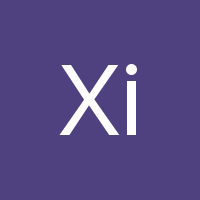
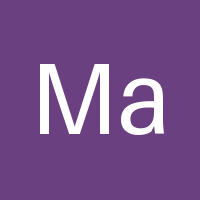
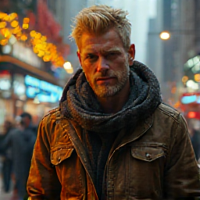
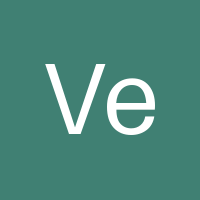
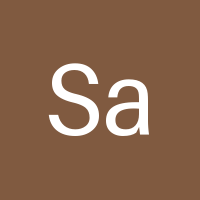
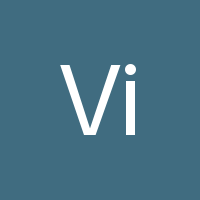
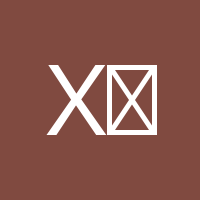
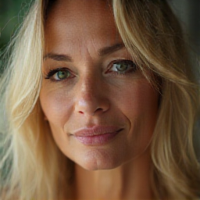
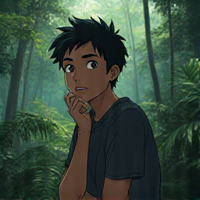
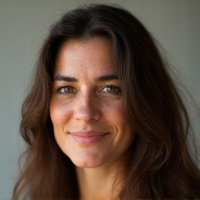
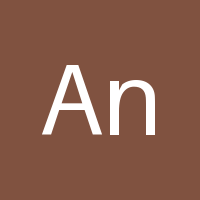
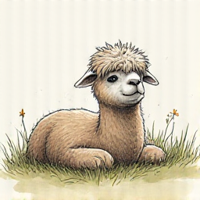
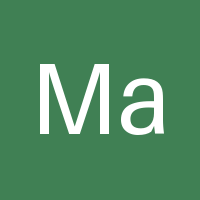
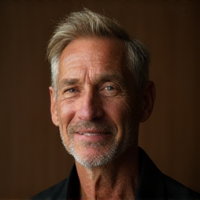
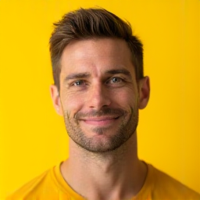
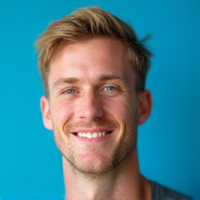
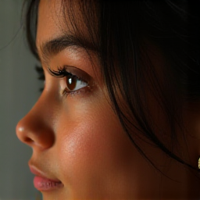
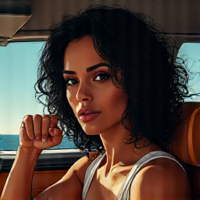
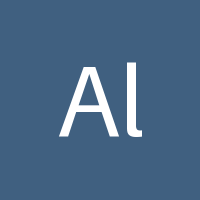
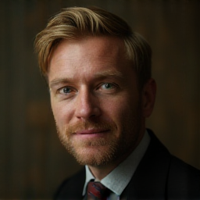
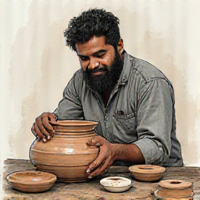
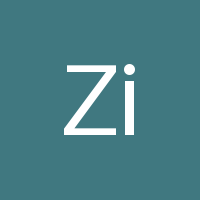
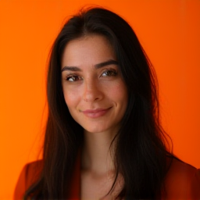
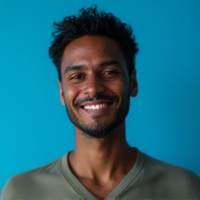
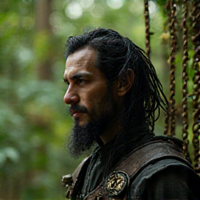
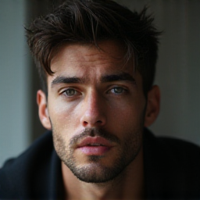
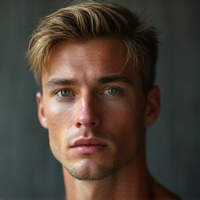
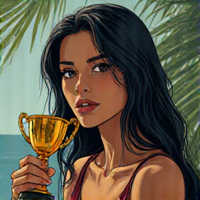
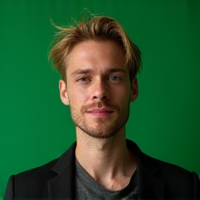
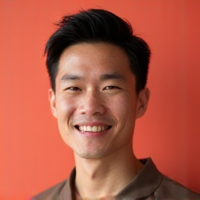
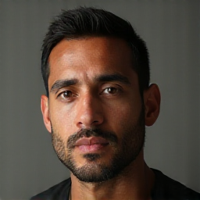
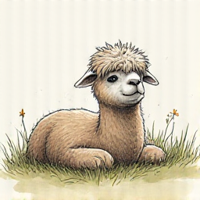
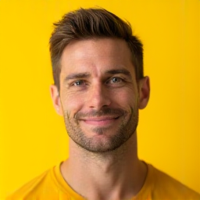
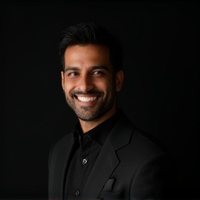
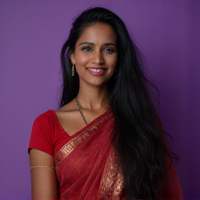
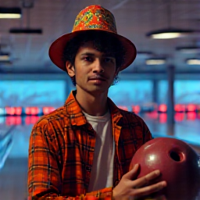
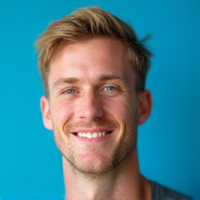
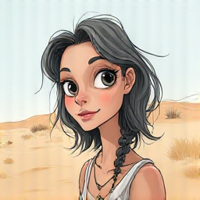
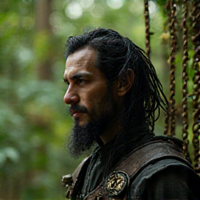
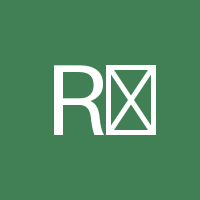
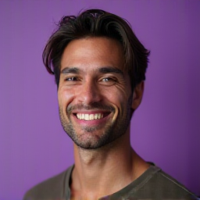
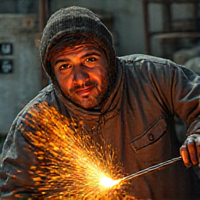
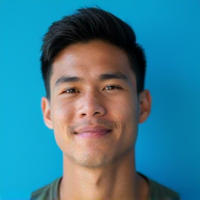
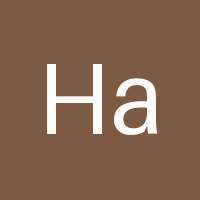
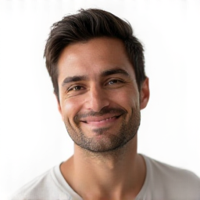
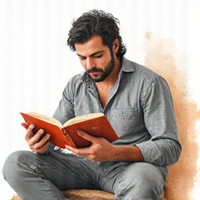
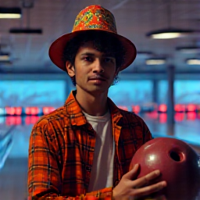
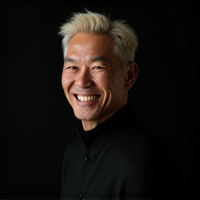
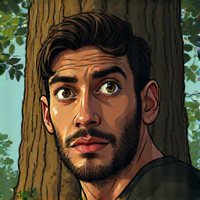
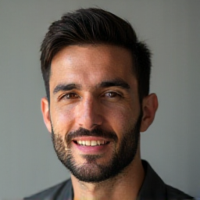
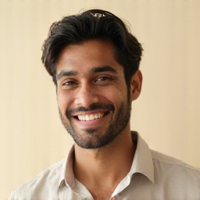