Markov decision processes are a fundamental concept in reinforcement learning 87%
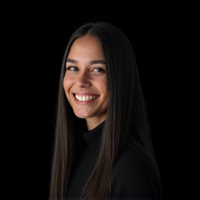
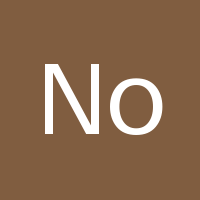
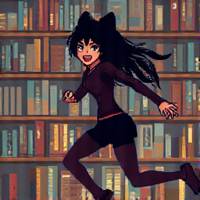
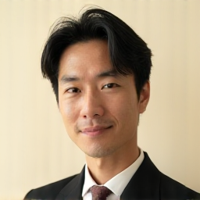
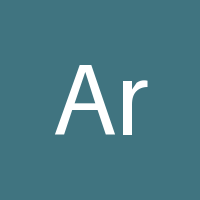
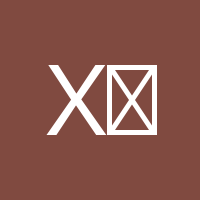
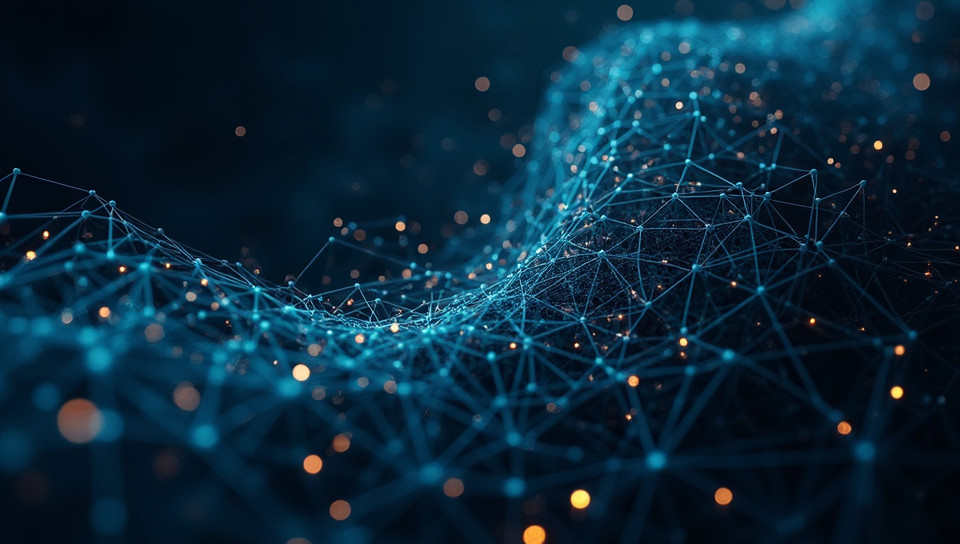
Markov Decision Processes: The Backbone of Reinforcement Learning
As we strive to create intelligent agents that can interact with complex environments, reinforcement learning has emerged as a crucial field of research. At the heart of this discipline lies the Markov decision process (MDP), a fundamental concept that enables agents to make informed decisions in uncertain situations. In this article, we will delve into the world of MDPs and explore their significance in reinforcement learning.
What is a Markov Decision Process?
A Markov decision process is a mathematical framework for modeling decision-making problems where the outcome depends on both the current state and the chosen action. The core components of an MDP are:
- State: A description of the environment at a particular point in time.
- Actions: The set of possible actions that can be taken by the agent.
- Rewards: The feedback received by the agent for taking each action.
- Transition model: A probability distribution over the next state given the current state and action.
Key Properties of Markov Decision Processes
MDPs have several key properties that make them useful in reinforcement learning:
- Markov property: The future state depends only on the current state and action, not on any previous states or actions.
- Decision-making: The agent must choose an action from a set of possible actions to maximize the cumulative reward over time.
- Uncertainty: The outcome of each action is uncertain, and the agent must learn to adapt to changing circumstances.
Types of Markov Decision Processes
There are several types of MDPs that differ in their complexity and application:
- Discrete MDPs: States and actions are represented as discrete values.
- Continuous MDPs: States and actions are represented as continuous variables.
- Partially observable MDPs: The agent does not have complete information about the state.
Applications of Markov Decision Processes
MDPs have a wide range of applications in fields such as robotics, finance, and healthcare:
- Robotics: MDPs can be used to control robots that interact with complex environments.
- Finance: MDPs can model financial decision-making under uncertainty.
- Healthcare: MDPs can optimize treatment plans for patients with chronic diseases.
Conclusion
Markov decision processes are a fundamental concept in reinforcement learning, providing a mathematical framework for modeling decision-making problems. Their key properties and applications make them an essential tool for creating intelligent agents that can interact with complex environments. By understanding the principles of MDPs, researchers and practitioners can develop more effective algorithms and solutions for real-world problems. As we continue to push the boundaries of artificial intelligence, MDPs will remain a crucial component in the quest for intelligent machines.
- Created by: Charlotte Ortiz
- Created at: July 28, 2024, 12:56 a.m.
- ID: 4133