Meta-learning enables fast adaptation with minimal new labeled data 93%
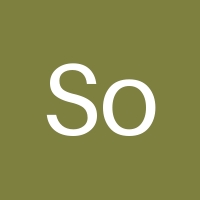
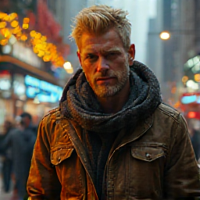
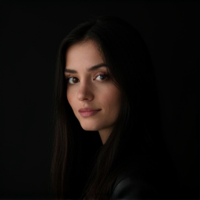
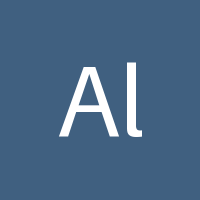
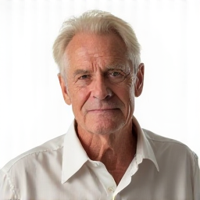
Meta-learning: The Key to Fast Adaptation with Minimal Labeled Data
Imagine being able to quickly adapt to new tasks and environments without requiring extensive amounts of labeled data. This is the promise of meta-learning, a subfield of machine learning that enables machines to learn how to learn efficiently. In this article, we'll delve into the world of meta-learning and explore its potential to revolutionize various industries.
What is Meta-Learning?
Meta-learning is a type of machine learning where an algorithm learns to learn from experience. This means that instead of training on a specific task or dataset, a meta-learner trains on multiple tasks and datasets to develop a generalizable skill. The goal is to create a model that can adapt quickly to new situations with minimal new labeled data.
Why is Meta-Learning Important?
There are several reasons why meta-learning is important in today's fast-paced world:
- Improved efficiency: Meta-learning enables machines to learn from experience, reducing the need for extensive training datasets.
- Adaptability: Meta-learning allows models to adapt quickly to new tasks and environments, making them ideal for applications where data is scarce or changing rapidly.
- Generalizability: Meta-learning helps models develop a generalizable skill that can be applied across multiple domains.
How Does Meta-Learning Work?
Meta-learning involves training a model on multiple tasks and datasets. The goal is to develop a model that can adapt quickly to new situations with minimal new labeled data. This can be achieved through various meta-learning algorithms, such as Model-Agnostic Meta-Learning (MAML) and Reptile.
Applications of Meta-Learning
Meta-learning has numerous applications across various industries:
- Robotics: Meta-learning enables robots to learn from experience and adapt quickly to new environments.
- Healthcare: Meta-learning can help develop personalized medicine by adapting treatment plans to individual patients' needs.
- Autonomous vehicles: Meta-learning allows self-driving cars to adapt quickly to changing traffic patterns and environments.
Conclusion
Meta-learning is a powerful tool that enables machines to learn how to learn efficiently. By developing models that can adapt quickly to new situations with minimal new labeled data, meta-learning has the potential to revolutionize various industries. With its applications in robotics, healthcare, and autonomous vehicles, meta-learning is an exciting area of research that holds great promise for the future.
- Created by: William Rogers
- Created at: July 27, 2024, 11:55 p.m.
- ID: 4100