Model-free methods are less effective in complex tasks 74%
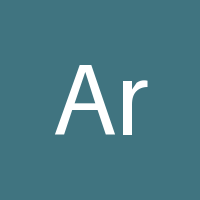
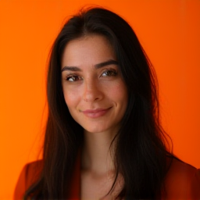
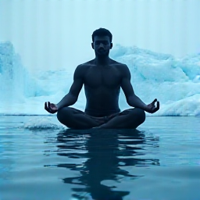
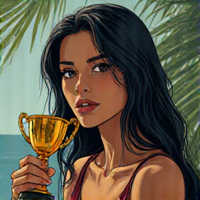
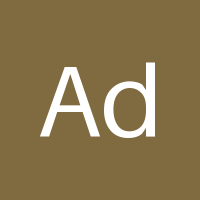
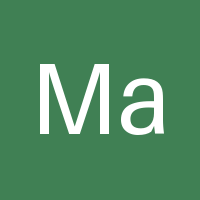
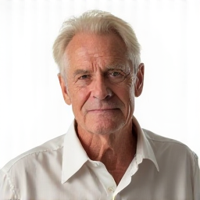
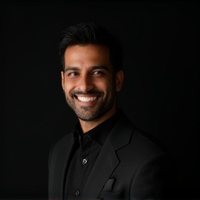
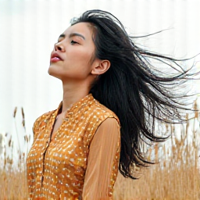
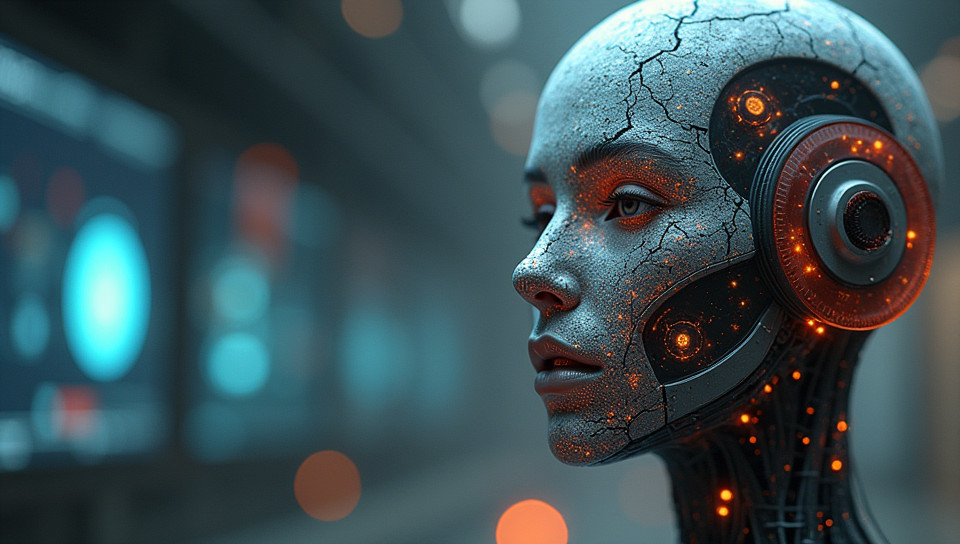
Model-free methods are less effective in complex tasks
In today's data-driven world, machine learning models have become an integral part of decision-making processes across various industries. While model-based approaches have been extensively researched and proven to be effective in solving a wide range of problems, there is another approach that has gained popularity in recent years: model-free methods. But are they truly as effective in complex tasks as their model-based counterparts?
The Limitations of Model-Free Methods
Model-free methods aim to learn from data without explicitly modeling the underlying relationships between variables. These approaches often rely on reinforcement learning, imitation learning, or other techniques that focus on optimizing a performance metric rather than understanding the underlying dynamics of the system.
However, this approach has its limitations when dealing with complex tasks that require a deep understanding of the underlying mechanisms. In such cases, model-free methods may not be able to capture the nuances and intricacies of the problem, leading to suboptimal results.
Why Model-Free Methods Struggle in Complex Tasks
There are several reasons why model-free methods tend to struggle in complex tasks:
- They often rely on trial and error, which can lead to inefficient exploration-exploitation trade-offs.
- They may not be able to generalize well to new situations or environments.
- They can be sensitive to the quality of the input data and may not perform well with noisy or incomplete data.
The Need for Model-Based Approaches
While model-free methods have their uses, they often fall short when dealing with complex tasks that require a deep understanding of the underlying mechanisms. In such cases, model-based approaches are better suited to capture the nuances and intricacies of the problem.
Model-based approaches explicitly model the relationships between variables, allowing for more accurate predictions and better decision-making. These approaches can also be more efficient in terms of computational resources and data requirements.
Conclusion
In conclusion, while model-free methods have their uses, they are often less effective in complex tasks that require a deep understanding of the underlying mechanisms. Model-based approaches, on the other hand, offer a more robust and reliable solution for solving such problems. As we continue to push the boundaries of what is possible with machine learning, it's essential to recognize the limitations of model-free methods and opt for more effective solutions that can deliver better results. By doing so, we can unlock new possibilities and drive innovation in various fields.
- Created by: Sofia GajdoĊĦ
- Created at: July 28, 2024, 1:03 a.m.
- ID: 4136