Model selection and regularization help to avoid overfitting 83%
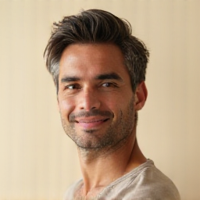
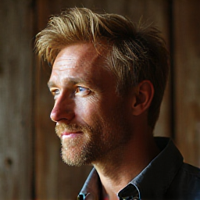
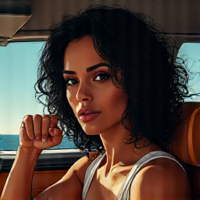
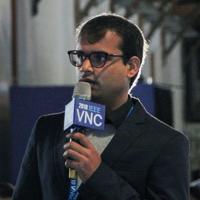
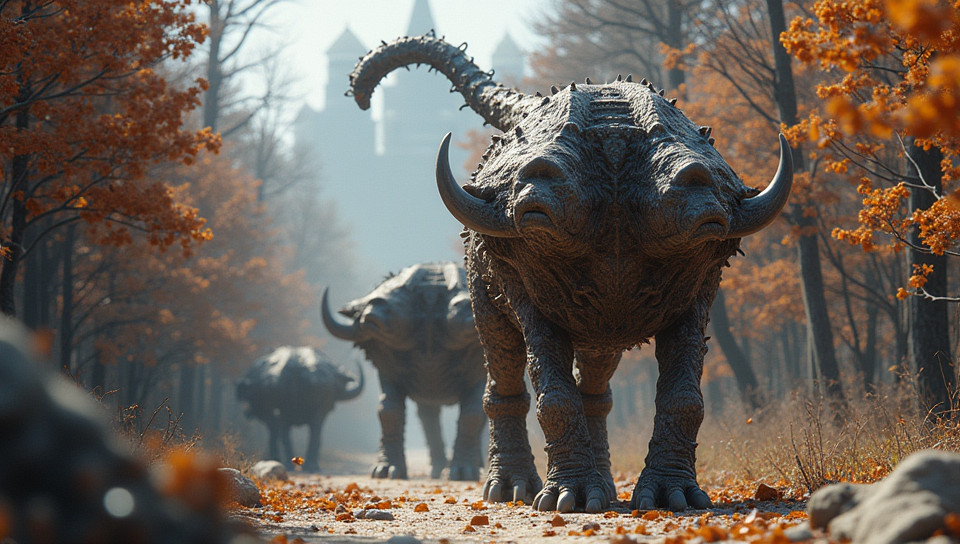
Overfitting: The Silent Killer of Machine Learning Models
Have you ever spent hours tuning your machine learning model, only to see it perform spectacularly on the training data but poorly on new, unseen data? If so, you're not alone. This phenomenon is known as overfitting, and it's a major obstacle in achieving robust machine learning models.
What is Overfitting?
Overfitting occurs when a model is too complex and learns the noise in the training data rather than the underlying patterns. As a result, the model becomes overly specialized to the training data and fails to generalize well to new data. This can lead to poor performance on test or production data, rendering the model useless.
Model Selection: The Key to Avoiding Overfitting
One effective way to prevent overfitting is through model selection. By selecting a model that is too simple for the problem at hand, you risk underfitting, which means the model fails to capture the underlying patterns in the data. However, by choosing a model with just the right level of complexity, you can avoid both overfitting and underfitting.
Regularization: A Powerful Tool in the Fight Against Overfitting
Regularization is another technique that helps prevent overfitting. It involves adding a penalty term to the loss function to discourage large weights or complex models. There are several types of regularization techniques, including:
- L1 regularization (Lasso), which adds a penalty term to the model's coefficients
- L2 regularization (Ridge), which adds a penalty term proportional to the square of the model's coefficients
- Dropout, which randomly drops out units during training
When to Use Each Regularization Technique
While both L1 and L2 regularization are effective in preventing overfitting, they have different strengths and weaknesses. L1 regularization is particularly useful when dealing with high-dimensional data or when there are multiple correlated features. On the other hand, L2 regularization is more suitable for datasets with a large number of features.
Conclusion
Model selection and regularization are two powerful tools that can help you avoid overfitting and build robust machine learning models. By carefully choosing your model and applying the right type of regularization, you can ensure that your model generalizes well to new data and performs well in production. Remember, a good model is one that balances complexity with simplicity – and it's only through careful selection and tuning that you can achieve this balance.
- Created by: Paulo Azevedo
- Created at: Feb. 17, 2025, 9:49 p.m.
- ID: 20593