Natural language processing (NLP) is applied to text-based data 73%
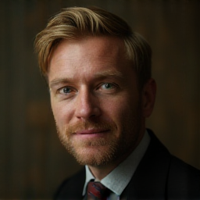
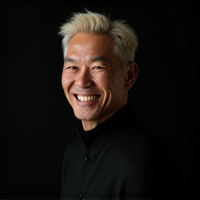
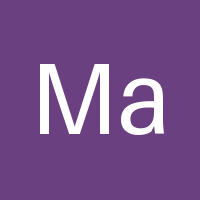
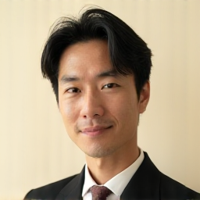
Unlocking the Power of Text-Based Data: The Rise of Natural Language Processing (NLP)
In today's digital age, text-based data is ubiquitous. From social media posts and online reviews to emails and chat logs, we are constantly generating and consuming vast amounts of text. However, this sea of words can be overwhelming, making it challenging for businesses and organizations to extract valuable insights from the noise. This is where Natural Language Processing (NLP) comes in – a field that has revolutionized the way we interact with text-based data.
What is NLP?
Natural Language Processing is a subfield of artificial intelligence (AI) that deals with the interaction between computers and humans in natural language. It involves using algorithms and statistical models to process, understand, and generate human language. NLP has numerous applications, including sentiment analysis, topic modeling, named entity recognition, and machine translation.
Text-Based Data: The Challenge
Text-based data presents several challenges that make it difficult for humans to analyze and extract insights from it. These include:
- Handling large volumes of unstructured text
- Identifying relevant information and patterns
- Mitigating the effects of language ambiguity and noise
- Ensuring consistency and accuracy in analysis results
The Role of NLP in Text-Based Data Analysis
NLP has emerged as a powerful tool to overcome these challenges. By applying NLP techniques, organizations can unlock valuable insights from text-based data, including:
- Sentiment analysis: understanding customer opinions and emotions
- Topic modeling: identifying underlying themes and patterns
- Named entity recognition: extracting specific entities such as names, locations, and organizations
- Text classification: categorizing text into predefined categories
Case Studies: Where NLP Has Made a Difference
NLP has been successfully applied in various industries, including:
- Customer service chatbots that use sentiment analysis to provide personalized support
- Social media monitoring tools that employ topic modeling to identify emerging trends and sentiments
- Healthcare organizations that leverage named entity recognition to extract relevant information from patient records
- Marketing teams that utilize text classification to categorize customer feedback and improve product development
Conclusion: Embracing the Power of NLP
Natural Language Processing has transformed the way we interact with text-based data, enabling us to extract valuable insights and make data-driven decisions. As organizations continue to generate vast amounts of text data, the need for effective NLP solutions will only grow. By embracing NLP, businesses can unlock new opportunities for growth, improvement, and innovation – making it an essential tool in today's competitive landscape.
- Created by: Yìzé Ko
- Created at: July 27, 2024, 6:11 a.m.
- ID: 3846