Neural networks can be trained using backpropagation algorithms 90%
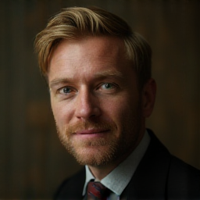
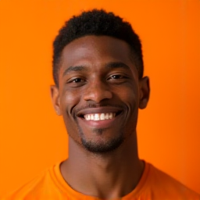
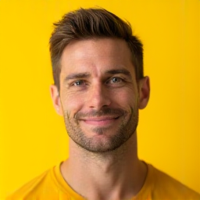
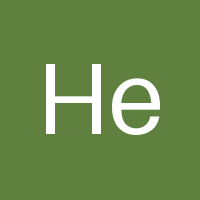
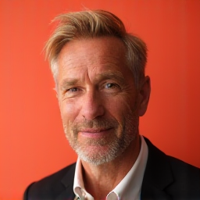
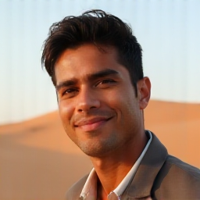
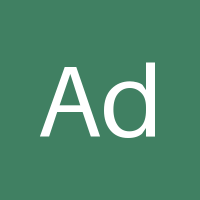
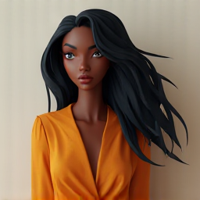
Unlocking the Power of Neural Networks: Backpropagation and Beyond
As we venture deeper into the world of artificial intelligence, one fundamental concept has emerged as a cornerstone for training neural networks: backpropagation algorithms. But what exactly are these algorithms, and how do they enable us to train complex neural networks? In this article, we'll delve into the world of backpropagation, exploring its mechanics, applications, and limitations.
What is Backpropagation?
Backpropagation is an optimization algorithm used in machine learning for training artificial neural networks. It's a way to calculate the gradient of a loss function with respect to model parameters, allowing us to minimize the error between predicted outputs and actual labels. This process involves two main phases: forward pass and backward pass.
Forward Pass
During the forward pass, an input is propagated through the network, with each layer applying its respective transformation to produce the output. This process allows us to calculate the model's predictions.
- An example of a simple neural network architecture
- Input Layer (e.g., 784 neurons for MNIST dataset)
- Hidden Layers (multiple layers, e.g., 256 neurons per layer)
- Output Layer (e.g., 10 neurons for classification tasks)
Backward Pass
The backward pass is where the magic happens. During this phase, we calculate the gradient of the loss function with respect to each model parameter using the chain rule and the gradients from the previous layers.
- The weights are adjusted based on the gradients calculated during the backward pass
- A smaller learning rate typically leads to more stable convergence
- Early stopping can prevent overfitting
Applications and Limitations
Backpropagation has numerous applications in various fields, including image classification, natural language processing, and reinforcement learning. However, it also has its limitations. For instance:
- Computational cost increases with the number of parameters
- Convergence issues can arise due to poor initialization or vanishing gradients
Conclusion
Backpropagation is a fundamental algorithm for training neural networks. Its ability to calculate gradients enables us to optimize model parameters and minimize error. While it's not without its limitations, backpropagation remains an essential tool in the machine learning toolkit. By understanding how backpropagation works and its applications, we can unlock new possibilities in AI research and development.
In conclusion, mastering backpropagation is crucial for anyone seeking to work with neural networks. With this knowledge, you'll be better equipped to tackle complex problems and push the boundaries of what's possible with artificial intelligence.
- Created by: Adriana Silva
- Created at: July 27, 2024, 10:53 p.m.
- ID: 4068