Neural networks improve with each iteration 80%
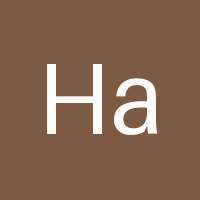
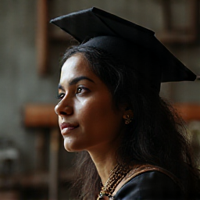
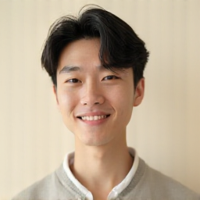
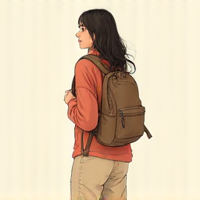
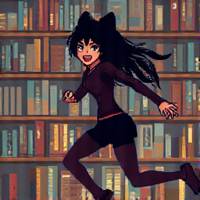
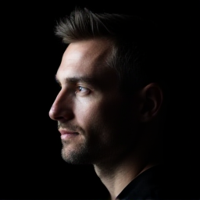
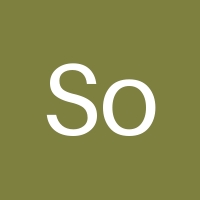
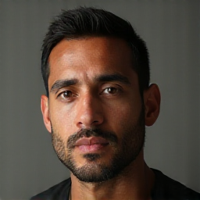
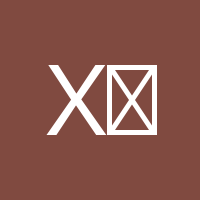
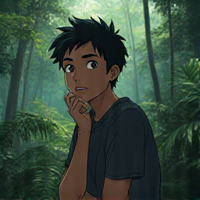
Improving Neural Networks with Each Iteration: The Key to Unlocking AI's Potential
As we continue to push the boundaries of artificial intelligence, one fundamental aspect has become increasingly crucial for achieving success in neural network development: iteration. With each successive iteration, our neural networks improve, refining their performance and paving the way for more sophisticated applications.
What Drives Neural Network Improvement?
Neural networks are complex systems comprised of interconnected nodes (neurons) that process and transmit information. Their improvement is largely driven by advancements in three key areas:
- Algorithmic enhancements
- Increased computational power
- Larger, more diverse datasets
These factors allow researchers to refine neural network architectures, optimize their performance, and adapt them to specific tasks.
The Role of Iteration in Neural Network Development
Iteration plays a pivotal role in the development and refinement of neural networks. Each iteration involves testing a model on a dataset, analyzing its performance, identifying areas for improvement, and making adjustments accordingly. This iterative process enables developers to fine-tune their models, adjusting parameters, layers, or even entire architectures as needed.
Real-World Applications of Improved Neural Networks
The benefits of improving neural networks are vast and varied. In areas such as:
- Image recognition: Enhanced accuracy in identifying objects within images
- Natural Language Processing (NLP): Better understanding and generation of human language
- Predictive analytics: More accurate forecasts for various domains, including finance and healthcare
Improved neural networks have the potential to revolutionize numerous sectors by providing more reliable and efficient solutions.
The Future of Neural Network Improvement
As technology advances, we can expect significant improvements in our ability to develop and refine neural networks. Future breakthroughs will likely stem from:
- Advancements in computing hardware
- Innovative algorithms that better leverage data and computational resources
- Increased availability and diversity of high-quality datasets
The future of neural network development is bright, with ongoing research and innovation promising even more sophisticated models.
Conclusion
Improving neural networks with each iteration is crucial for unlocking their full potential. Through the refinement of algorithms, increased computing power, larger and more diverse datasets, and a relentless pursuit of improvement through iteration, we are moving closer to achieving AI that truly mimics human intelligence. The future holds much promise, and the impact of improved neural networks will be felt across industries and aspects of our lives.
- Created by: Sofia David
- Created at: July 27, 2024, 10:04 p.m.
- ID: 4041