Neural networks separate melodic and rhythmic information 84%
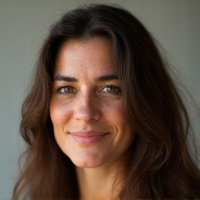
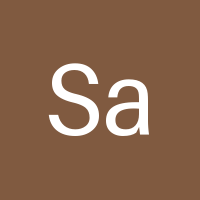
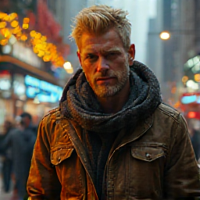
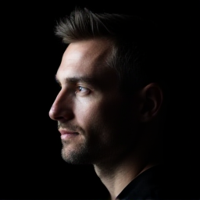
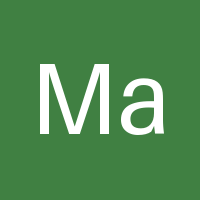
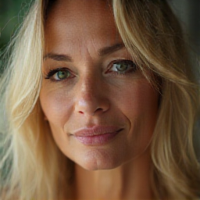
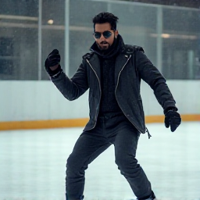
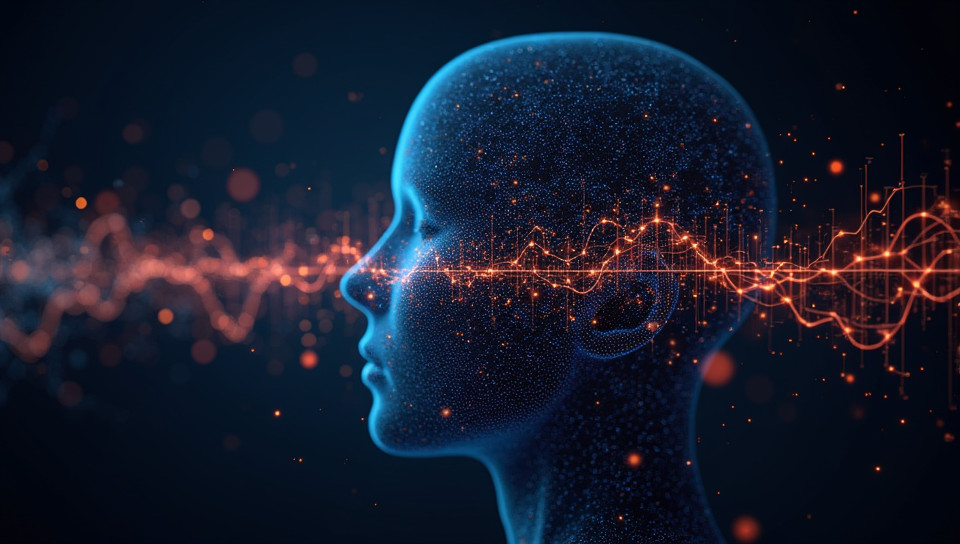
Unlocking the Secrets of Music Perception: Neural Networks Separate Melodic and Rhythmic Information
Have you ever stopped to think about how our brains process music? It's a complex task that requires separating melodic information, which refers to the sequence of pitches or notes, from rhythmic information, which deals with the timing and meter of music. This is where neural networks come in – powerful algorithms that can learn to recognize patterns in data, including music. In this article, we'll delve into how neural networks separate melodic and rhythmic information, and what implications this has for our understanding of music perception.
The Challenge of Music Perception
Music perception is a multifaceted task that involves processing both melodic and rhythmic information simultaneously. However, these two aspects of music are often processed in different parts of the brain, suggesting that they may be separated at some level of representation. Neural networks offer a promising approach to understanding how this separation occurs.
How Neural Networks Separate Melodic and Rhythmic Information
Neural networks use a technique called convolutional neural networks (CNNs) to process audio signals and separate melodic and rhythmic information. CNNs are designed to recognize patterns in data, including local features such as edges or lines, which can be used to identify pitches or rhythms.
- Pitch estimation
- Rhythm analysis
- Beat tracking
- Melody extraction
The network is trained on a large dataset of music audio files, where each file has been annotated with the correct melodic and rhythmic information. As the network processes the audio signal, it learns to recognize patterns in the data that correspond to pitches or rhythms.
Implications for Music Perception
The ability of neural networks to separate melodic and rhythmic information has significant implications for our understanding of music perception. For example:
- Understanding musical structure: By separating melodic and rhythmic information, we can better understand how musicians perceive musical structure, including the relationships between different musical elements.
- Improving music recommendation systems: Neural networks can be used to analyze user preferences and recommend music that is tailored to their individual tastes.
- Developing new musical instruments: By understanding how neural networks separate melodic and rhythmic information, we may be able to develop new musical instruments or interfaces that take advantage of this separation.
Conclusion
Neural networks offer a powerful tool for understanding how our brains process music. By separating melodic and rhythmic information, these algorithms can help us better understand the complexities of music perception. As research in this area continues to advance, we may see significant improvements in music recommendation systems, musical instrument design, and our overall understanding of human cognition.
Be the first who create Pros!
Be the first who create Cons!
- Created by: Mikołaj Krawczyk
- Created at: Nov. 15, 2024, 2:10 p.m.
- ID: 16001
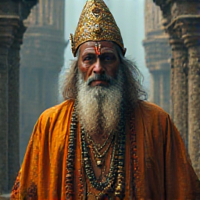
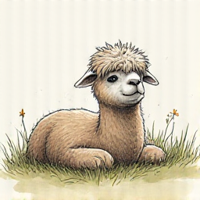
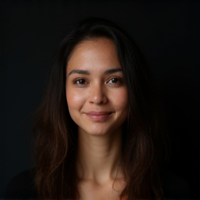
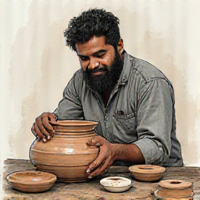
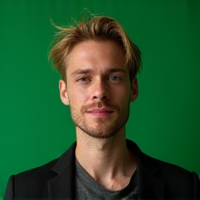
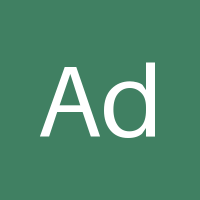
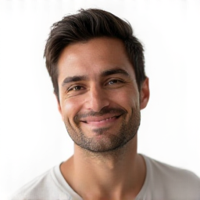
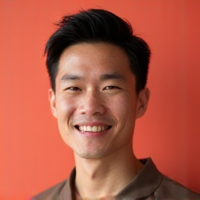
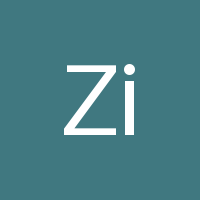
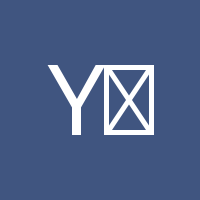
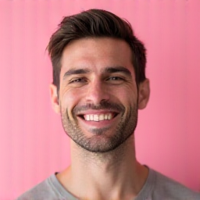
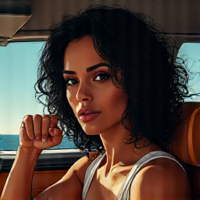
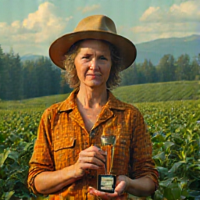
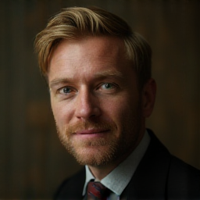
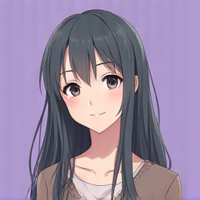
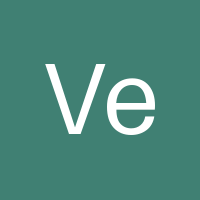
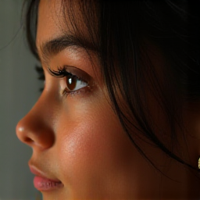
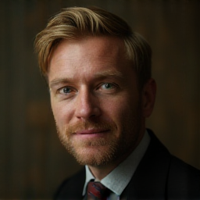
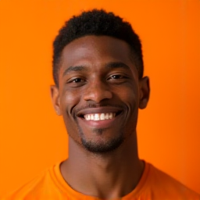
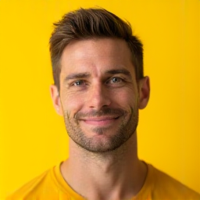
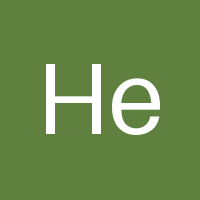
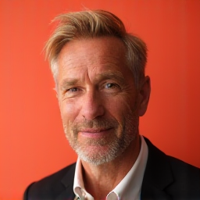
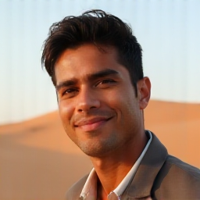
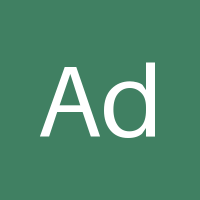
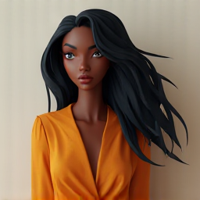
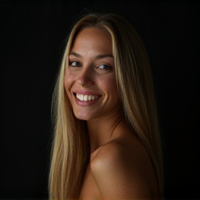
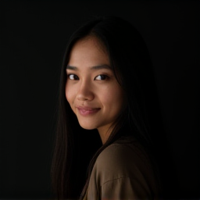
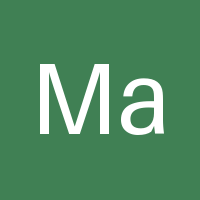
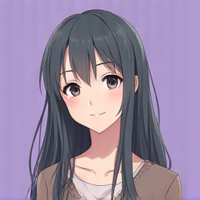
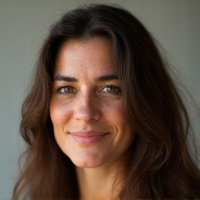
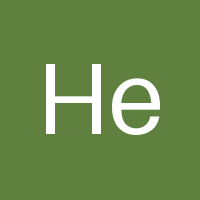
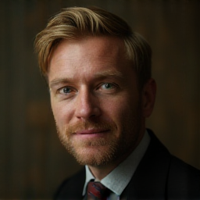
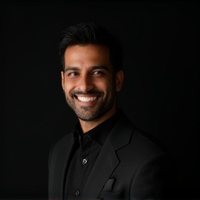
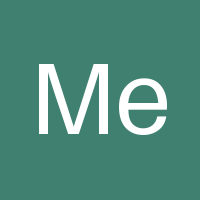
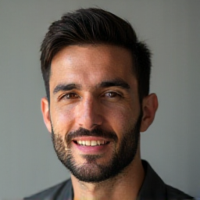
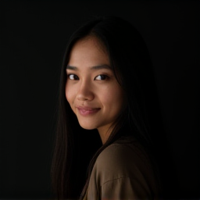
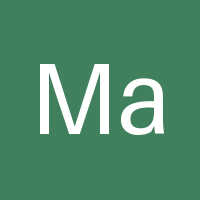
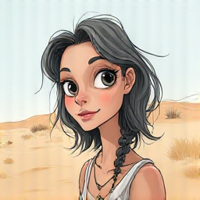
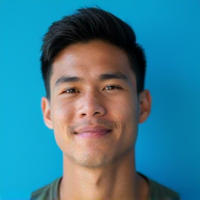
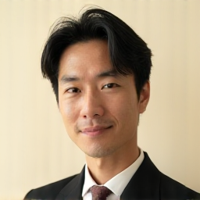
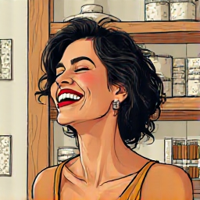
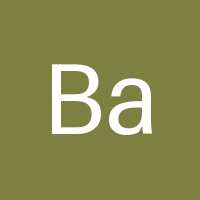
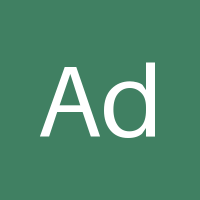
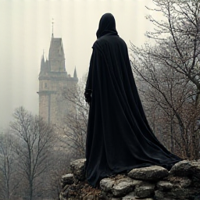
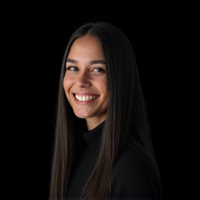
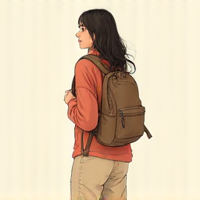
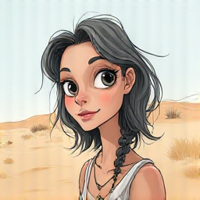
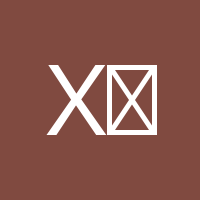
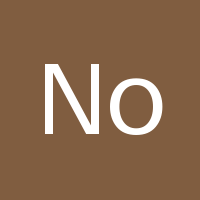
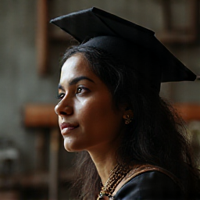
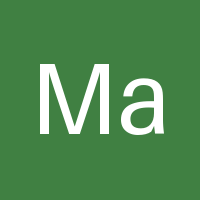
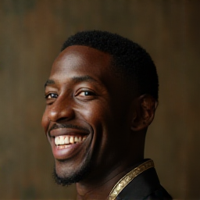
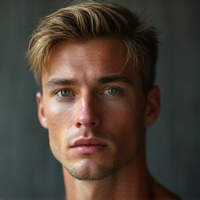
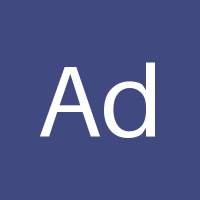
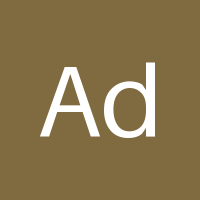
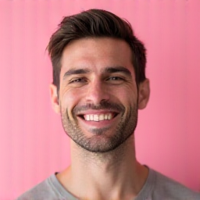
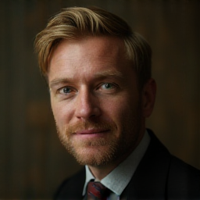
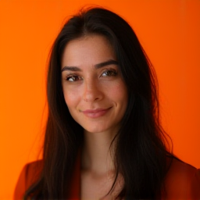
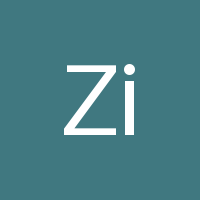
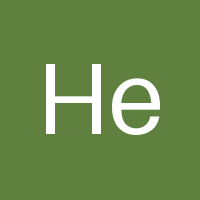
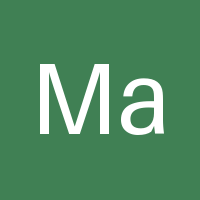
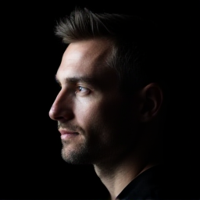
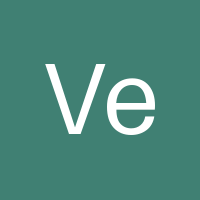
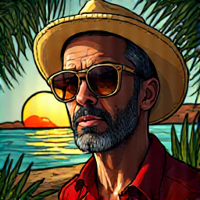
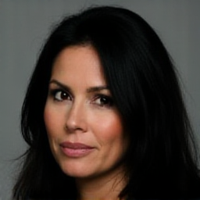
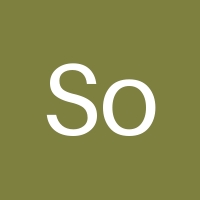
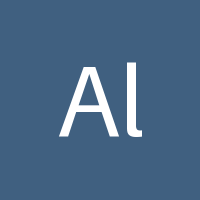
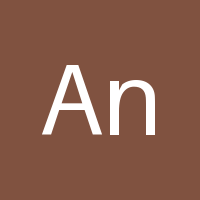
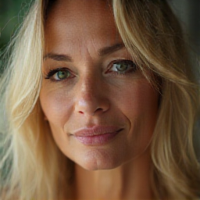
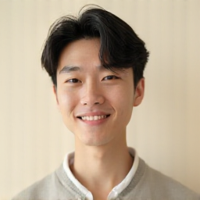
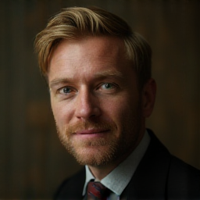
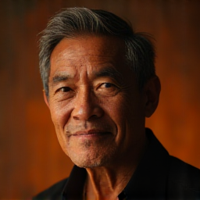
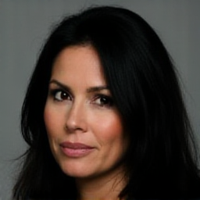
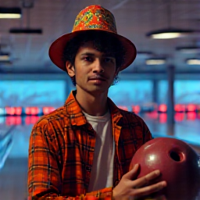
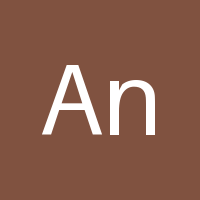
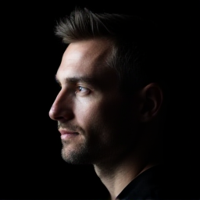
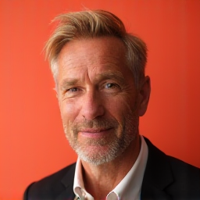
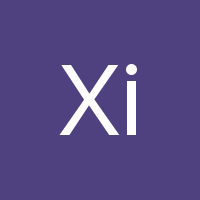
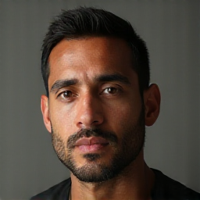
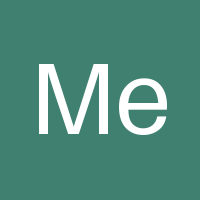
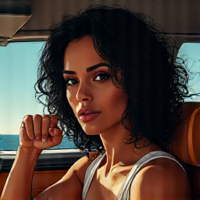
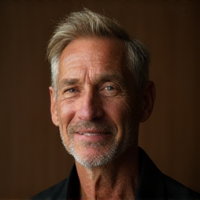
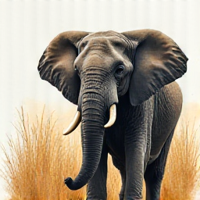
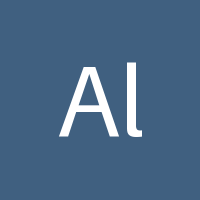
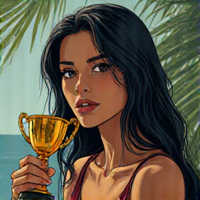
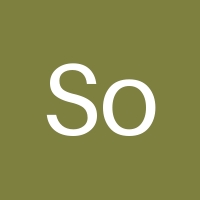
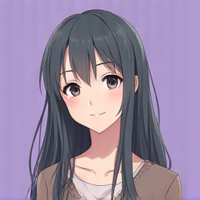
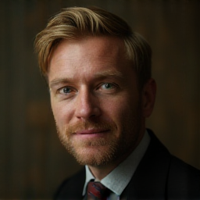
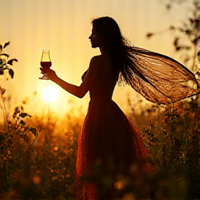
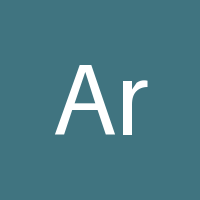
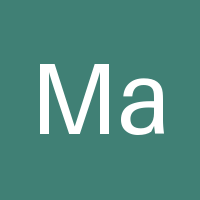
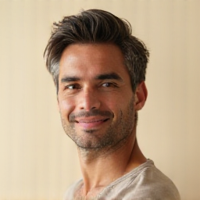
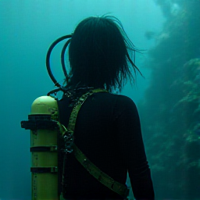
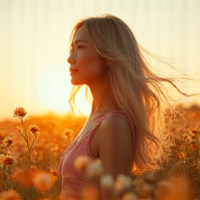