Non-parametric tests can achieve better results than neural nets 69%
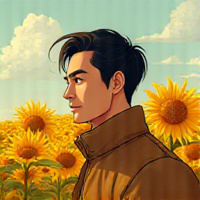
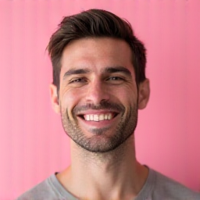
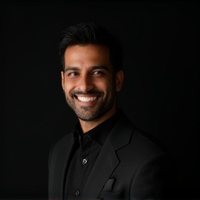
The Unlikely Winners: Why Non-Parametric Tests Can Outshine Neural Nets
Imagine being told that your trusty old car, which you've lovingly maintained over the years, can outperform a sleek new sports car on the open road. Sounds preposterous, right? Yet, in the world of data analysis and machine learning, non-parametric tests are quietly achieving better results than their more popular neural net counterparts.
The Rise of Neural Nets
Neural nets have been all the rage in recent years, with their ability to learn complex patterns and relationships from vast amounts of data. They've been touted as the solution to some of the world's most pressing problems, from medical diagnosis to climate modeling. And while they're indeed powerful tools, there are limitations to their abilities.
The Limitations of Neural Nets
- They require large amounts of labeled data to train effectively.
- They can be prone to overfitting and underfitting.
- They often rely on complex mathematical formulations that can be difficult to interpret.
The Unsung Heroes: Non-Parametric Tests
Non-parametric tests, on the other hand, are a type of statistical analysis that doesn't require assumptions about the underlying data distribution. They're often simpler and more intuitive than neural nets, making them easier to understand and apply.
Advantages of Non-Parametric Tests
- They can handle small sample sizes and noisy data with ease.
- They're less prone to overfitting and underfitting.
- They provide clear, interpretable results that are easy to communicate to stakeholders.
Real-World Applications
Non-parametric tests have been successfully applied in a variety of fields, including:
- Quality control: detecting anomalies in manufacturing processes
- Finance: identifying trends and patterns in stock market data
- Healthcare: predicting patient outcomes from medical imaging data
Conclusion
While neural nets may get all the attention, non-parametric tests are quietly achieving better results in many real-world applications. By leveraging their simplicity and flexibility, data analysts can gain insights that would be impossible to achieve with more complex models. So the next time you're faced with a challenging data analysis problem, don't automatically reach for the neural net – consider giving non-parametric tests a try.
- Created by: Viraj Patel
- Created at: July 27, 2024, 11:11 p.m.
- ID: 4076