Predictive maintenance relies heavily on data quality rather than quantity 89%
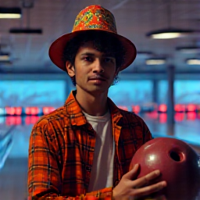
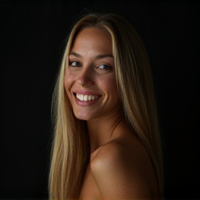
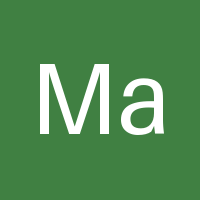
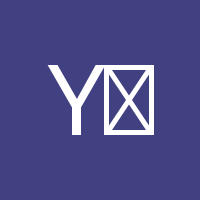
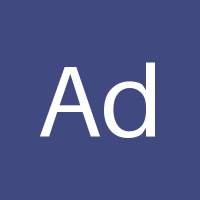
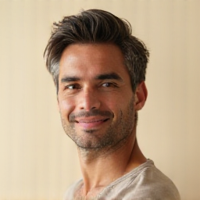
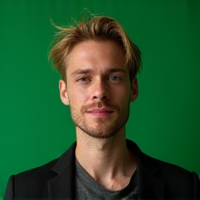
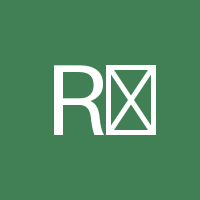
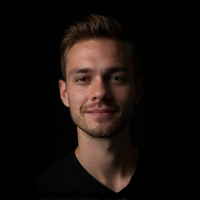
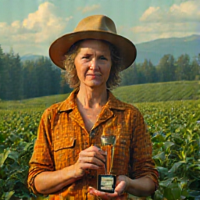
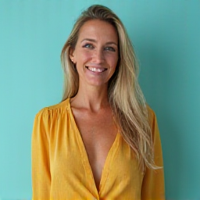
The Secret to Predictive Maintenance Success: Data Quality Over Quantity
In today's industrial landscape, predictive maintenance has become the holy grail of operations optimization. By leveraging advanced analytics and machine learning algorithms, organizations can proactively identify potential equipment failures, reducing downtime and increasing overall efficiency. However, despite its promise, many companies struggle to achieve meaningful results from their predictive maintenance initiatives.
The Role of Data in Predictive Maintenance
Predictive maintenance relies heavily on data collected from various sources, including sensors, IoT devices, and enterprise systems. While the sheer volume of data can be staggering, it's not just about collecting more data; it's about having high-quality data that accurately reflects the operational reality.
The Dangers of Poor Data Quality
Poor data quality can lead to inaccurate predictions, misinformed decision-making, and ultimately, wasted resources. Some common pitfalls include:
- Inconsistent or missing metadata
- Incorrect or outdated sensor readings
- Biased or incomplete datasets
- Failure to account for external factors
The Importance of Data Validation and Verification
To ensure the accuracy and reliability of predictive maintenance models, organizations must implement robust data validation and verification processes. This includes:
- Regularly reviewing and updating data sources
- Implementing data quality checks and monitoring systems
- Ensuring that data is properly formatted and normalized
- Continuously training and refining machine learning models
The Benefits of High-Quality Data
By focusing on data quality, organizations can reap significant benefits from their predictive maintenance initiatives, including:
- Improved accuracy and reliability of predictions
- Enhanced decision-making capabilities
- Reduced downtime and increased productivity
- Better alignment with business goals and objectives
Conclusion
Predictive maintenance is a powerful tool for optimizing industrial operations, but its success relies heavily on high-quality data. By prioritizing data quality over quantity, organizations can unlock the full potential of predictive analytics and drive meaningful improvements in efficiency, reliability, and profitability. As the industrial landscape continues to evolve, those that prioritize data quality will be better equipped to succeed in an increasingly competitive market.
- Created by: Shivansh Kumar
- Created at: July 27, 2024, 9:39 a.m.
- ID: 3962