Predictive models are not built without prior knowledge 77%
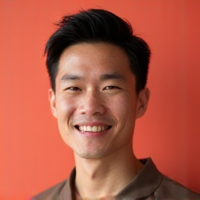
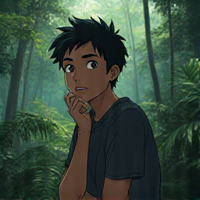
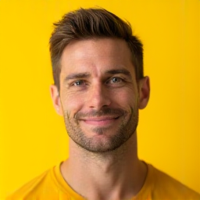
The Hidden Truth About Predictive Models
As data scientists, we're often tasked with building predictive models that can forecast future outcomes with uncanny accuracy. We pour over datasets, experiment with different algorithms, and fine-tune our models until they're humming along smoothly. But have you ever stopped to think about what makes a predictive model truly effective? The answer lies not in the algorithm itself, but in the prior knowledge we bring to the table.
The Problem of Overfitting
When building predictive models, it's easy to fall into the trap of overfitting. This occurs when our model is too closely tailored to the specific dataset we're working with, rather than learning generalizable patterns that apply across different contexts. But how do we avoid this pitfall?
The Role of Prior Knowledge in Predictive Modeling
Prior knowledge refers to any information we bring to the table about the problem domain, such as:
- Domain expertise
- Business understanding
- Data quality and limitations
- Relevant literature and research findings
- Previous experience working with similar datasets or models
This prior knowledge serves as a foundation for our predictive model, guiding us in our feature engineering decisions, algorithm selection, and hyperparameter tuning. By incorporating these insights into our modeling process, we can build more robust, reliable, and generalizable models that truly excel.
The Benefits of Prior Knowledge
So why is prior knowledge so crucial to building effective predictive models? For one thing, it allows us to:
- Avoid the pitfalls of overfitting by recognizing patterns that generalize across different datasets
- Make informed decisions about feature engineering and algorithm selection
- Identify potential biases in our data or modeling process
- Communicate more effectively with stakeholders about the limitations and assumptions underlying our model
Conclusion
Predictive models are not built without prior knowledge. In fact, it's this knowledge that sets us up for success, enabling us to build models that truly deliver on their promise. By recognizing the importance of prior knowledge in predictive modeling, we can take a more holistic approach to building these models, one that balances technical expertise with business acumen and domain insight. Only then can we unlock the full potential of our predictive models, driving real-world impact and value for our organizations.
- Created by: Mùchén Chu
- Created at: July 28, 2024, 12:24 a.m.
- ID: 4115