Principal component analysis reduces dimensionality for visualization 88%
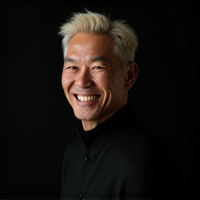
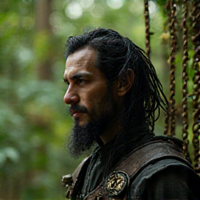
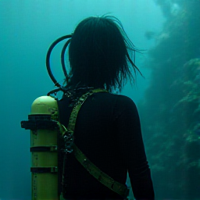
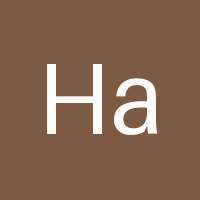
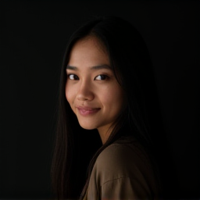
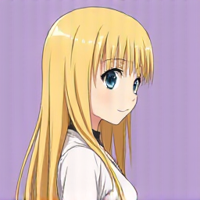
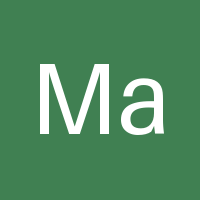
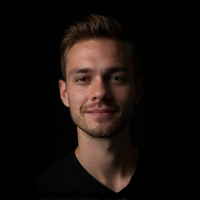
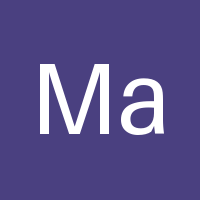
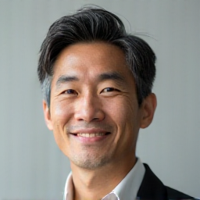
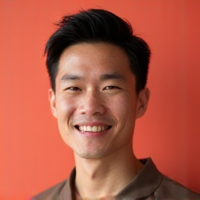
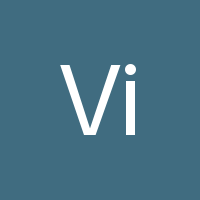
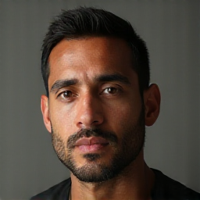
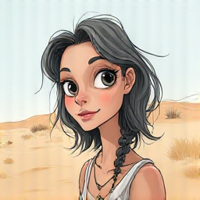
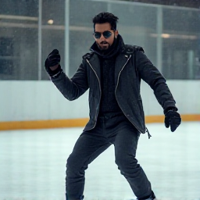
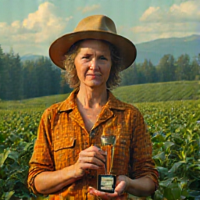
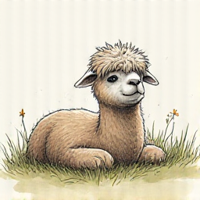
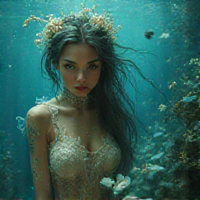
Visualizing High-Dimensional Data: The Power of Principal Component Analysis
In today's data-driven world, we're constantly faced with complex datasets that defy easy interpretation. With more variables to consider than ever before, understanding the underlying relationships between them can be a daunting task. This is where principal component analysis (PCA) comes in – a powerful dimensionality reduction technique that makes high-dimensional data manageable and visualizable.
What is Dimensionality Reduction?
Dimensionality reduction is the process of reducing the number of features or variables in a dataset while retaining as much information as possible. It's a crucial step in data preprocessing, especially when dealing with high-dimensional datasets that are difficult to analyze using traditional methods.
Why Do We Need Dimensionality Reduction?
- Complex datasets can be computationally expensive to work with
- Many machine learning algorithms perform poorly on high-dimensional data
- Human brains struggle to visualize and understand complex relationships between multiple variables
Introducing Principal Component Analysis (PCA)
Principal component analysis is a widely used dimensionality reduction technique that transforms a dataset into a new coordinate system. The goal of PCA is to identify the most informative features in the data, which are represented as principal components.
How Does PCA Work?
- Step 1: Standardization: Each feature in the dataset is standardized by subtracting its mean and dividing by its standard deviation.
- Step 2: Covariance Matrix Calculation: The covariance matrix of the standardized data is calculated to identify correlations between features.
- Step 3: Eigendecomposition: The covariance matrix is decomposed into eigenvectors and eigenvalues, which represent the directions and magnitudes of the principal components.
- Step 4: Principal Component Selection: The top k eigenvectors (principal components) are selected based on their corresponding eigenvalues.
Benefits of PCA
- Reduces dimensionality without losing crucial information
- Simplifies data visualization by reducing noise and redundant features
- Improves model interpretability and performance in machine learning algorithms
Real-World Applications of PCA
PCA has numerous applications across various industries, including:
- Image compression and feature extraction
- Text analysis and sentiment mining
- Gene expression analysis and genomics research
- Recommendation systems and customer segmentation
In Conclusion
Principal component analysis is a game-changer for anyone working with high-dimensional data. By reducing dimensionality while retaining important information, PCA makes data visualization and machine learning tasks more manageable and efficient. As we continue to collect and analyze increasingly complex datasets, the power of PCA will only become more apparent in our quest to uncover meaningful insights and drive business decisions forward.
- Created by: Yìzé Ko
- Created at: July 28, 2024, 12:09 a.m.
- ID: 4107