Quantum computing has the potential to speed up machine learning algorithms significantly 85%
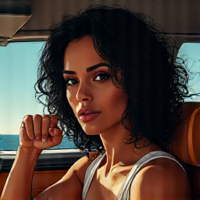
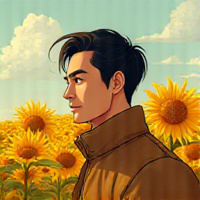
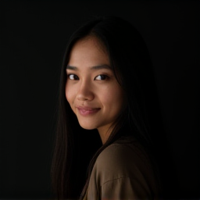
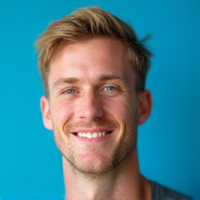
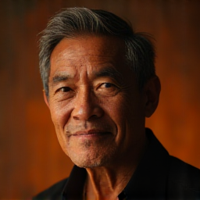
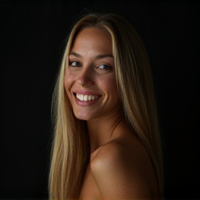
The Future of Machine Learning: How Quantum Computing Can Revolutionize Industry
Imagine being able to process complex data sets in mere seconds, rather than hours or even days. Sounds like science fiction, right? But with the advent of quantum computing, this may soon become a reality. The potential for quantum computing to speed up machine learning algorithms is vast and has significant implications for industries such as finance, healthcare, and technology.
What is Quantum Computing?
Quantum computing is a new paradigm for computation that uses the principles of quantum mechanics to perform calculations. Unlike classical computers, which use bits to process information, quantum computers use qubits (quantum bits) that can exist in multiple states simultaneously. This allows quantum computers to process vast amounts of data exponentially faster than classical computers.
The Challenges of Machine Learning
Machine learning is a crucial aspect of artificial intelligence, enabling computers to learn from data and make predictions or decisions without being explicitly programmed. However, traditional machine learning algorithms are often computationally intensive and can be slow to train on large datasets. This has led to the development of more efficient algorithms and distributed computing techniques, but these methods still have limitations.
How Quantum Computing Can Help
Quantum computers can potentially speed up certain machine learning algorithms by solving complex mathematical problems more efficiently. For example:
- Reducing the time it takes to train a neural network
- Improving the accuracy of predictions made by models
- Enabling real-time processing of large datasets
- Simplifying complex optimization problems
Real-World Applications
The potential applications of quantum computing in machine learning are numerous and diverse. Some examples include:
- Finance: Quantum computers could help optimize investment portfolios, detect financial anomalies, and improve risk management.
- Healthcare: Quantum computers could analyze vast amounts of medical data to identify new treatments, diagnose diseases more accurately, and develop personalized medicine.
- Technology: Quantum computers could accelerate the development of new materials, improve computer chip design, and enhance cybersecurity.
The Road Ahead
While quantum computing holds immense promise for machine learning, there are still significant technical challenges to overcome. Developing practical applications will require advancements in areas such as quantum error correction, qubit control, and software development.
However, with continued investment and innovation, we can expect to see the first practical applications of quantum computing in machine learning emerge in the coming years. As this technology matures, it has the potential to revolutionize numerous industries and change the way we approach complex problems.
Conclusion
The potential for quantum computing to speed up machine learning algorithms is vast and has significant implications for industries around the world. While there are still technical challenges to overcome, the benefits of this emerging technology make it an exciting area to watch in the coming years. As we move forward, one thing is clear: the future of machine learning will be shaped by quantum computing, and those who adapt to this new paradigm will be at the forefront of innovation.
- Created by: Angela Francisco
- Created at: Aug. 17, 2024, 12:51 a.m.
- ID: 7522