Raw data is often fragmented and difficult to analyze 76%
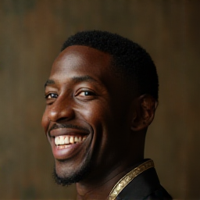
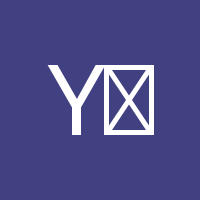
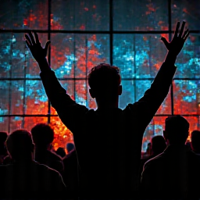
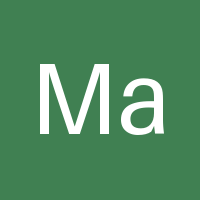
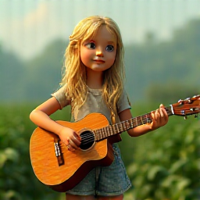
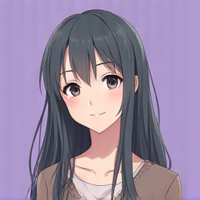
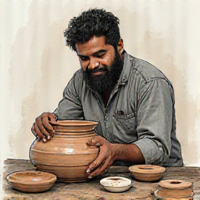
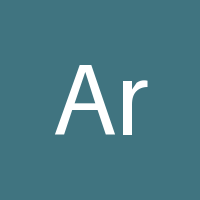
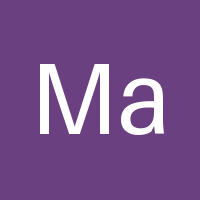
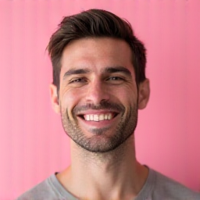
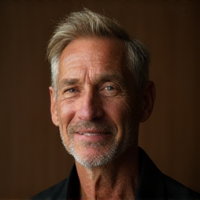
The Elusive Truth: Unpacking the Challenges of Raw Data Analysis
In today's data-driven world, organizations are sitting on vast amounts of raw data, eager to unlock its secrets and gain valuable insights. However, many struggle to make sense of this data due to its inherent complexities.
The Fragmented Nature of Raw Data
Raw data is often scattered across various sources, including social media platforms, customer databases, sensors, and more. This fragmentation makes it challenging to collect, process, and analyze the data in a meaningful way.
Characteristics of Fragmented Raw Data
- Unstructured or semi-structured format
- Multiple formats and schema
- Lack of standardization
- High volume and velocity
- Variable quality and accuracy
The Consequences of Ineffective Data Analysis
When raw data remains fragmented, organizations miss out on the opportunity to gain valuable insights. This can lead to:
Missed Business Opportunities
- Failing to identify emerging trends and patterns
- Missing opportunities for process improvements and cost savings
- Struggling to make informed decisions in a rapidly changing market
Breaking Down Barriers: Strategies for Effective Data Analysis
To overcome the challenges of raw data analysis, organizations must adopt strategies that address the fragmented nature of their data. This includes:
Implementing Data Integration Solutions
- Leveraging cloud-based data warehousing and integration platforms
- Using data virtualization to integrate disparate sources
- Adopting APIs and microservices for seamless data exchange
The Future of Data Analysis: A Unified Approach
As organizations continue to navigate the complexities of raw data analysis, they must prioritize a unified approach that recognizes the inherent value in fragmented data. By adopting innovative strategies and technologies, businesses can unlock the full potential of their data and drive meaningful insights that fuel growth and success.
Conclusion
Raw data is often seen as an untapped resource, but its true value lies in its ability to reveal hidden patterns and trends when properly analyzed. By acknowledging the challenges posed by fragmented raw data, organizations can begin to break down barriers and unlock the secrets of their data. As we move forward into a data-driven future, it's essential that businesses prioritize effective data analysis and adopt strategies that recognize the inherent value in diverse and complex datasets.
- Created by: Maria Thomas
- Created at: July 27, 2024, 2:14 a.m.
- ID: 3696