Recurrent neural networks analyze sequential data effectively 83%
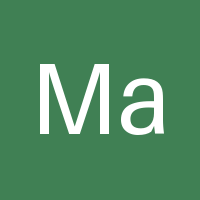
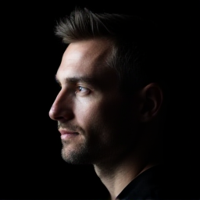
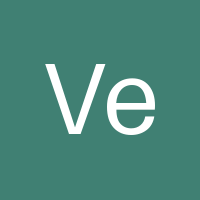
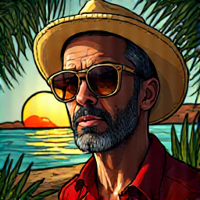
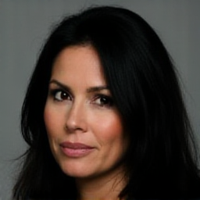
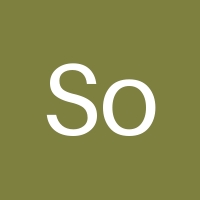
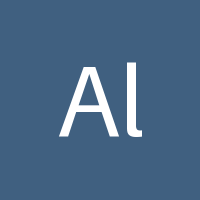
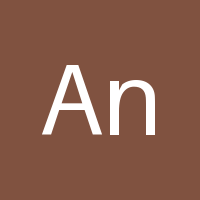
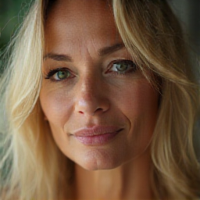
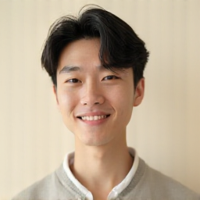
Recurrent Neural Networks: Unlocking the Power of Sequential Data
In today's data-driven world, analyzing sequential data has become increasingly important across various industries. From predicting stock prices to understanding customer behavior, the ability to extract insights from complex sequences of events is crucial for making informed decisions. This is where recurrent neural networks (RNNs) come in – a type of artificial intelligence that has revolutionized the way we approach sequential data analysis.
What are Recurrent Neural Networks?
Recurrent neural networks are a class of neural networks that are specifically designed to handle sequential data. Unlike traditional feedforward neural networks, RNNs have feedback connections between hidden units, allowing them to maintain an internal state and capture temporal dependencies in the input data.
How do Recurrent Neural Networks Work?
- They process sequences one step at a time.
- The output from each step is fed back into the network as input for the next step.
- This allows RNNs to learn complex patterns and relationships within sequential data.
Applications of Recurrent Neural Networks
RNNs have been successfully applied in various fields, including:
- Natural Language Processing (NLP): RNNs are used for tasks such as language modeling, sentiment analysis, and machine translation.
- Time Series Prediction: RNNs can predict future values in a time series based on past observations.
- Speech Recognition: RNNs are used to recognize spoken words and phrases.
The Benefits of Recurrent Neural Networks
- Handling Sequential Data: RNNs can effectively handle sequential data, making them ideal for tasks that involve time or order dependencies.
- Temporal Dependencies: RNNs can capture temporal relationships between data points, allowing for more accurate predictions and insights.
- Improved Accuracy: By learning from patterns in sequential data, RNNs can achieve higher accuracy rates compared to traditional machine learning models.
Challenges with Recurrent Neural Networks
While RNNs have revolutionized the way we analyze sequential data, they also come with some challenges:
- Vanishing Gradients: The gradients of the loss function can become very small during backpropagation, making it difficult for the network to learn.
- Exploding Gradients: Conversely, the gradients can become too large, causing the network to diverge.
Conclusion
Recurrent neural networks have undoubtedly become a crucial tool in the field of machine learning. Their ability to analyze sequential data effectively has opened up new avenues for insights and understanding across various industries. While challenges exist, ongoing research and advancements in RNN architectures are continually improving their performance and reliability. As we continue to explore the vast potential of RNNs, one thing is clear: they will remain a cornerstone in our pursuit of unlocking the secrets hidden within sequential data.
Be the first who create Pros!
Be the first who create Cons!
- Created by: Mikołaj Krawczyk
- Created at: July 27, 2024, 10:49 p.m.
- ID: 4066
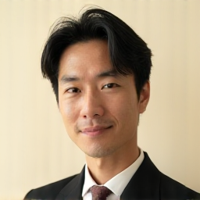
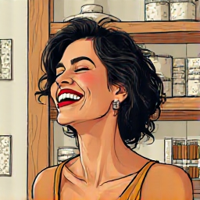
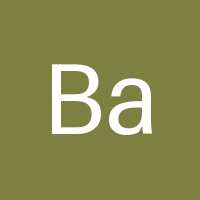
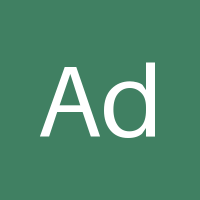
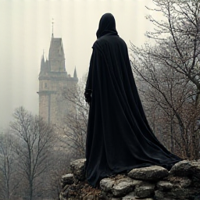
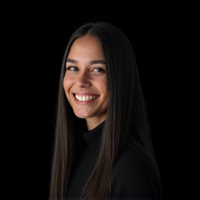
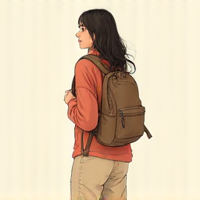
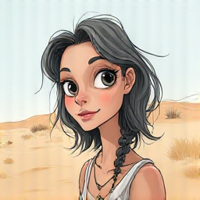
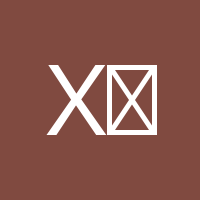
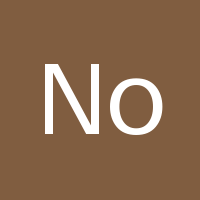
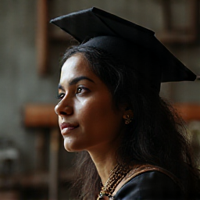
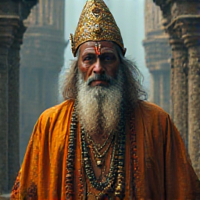
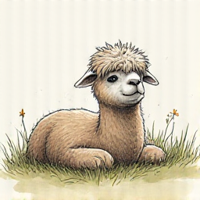
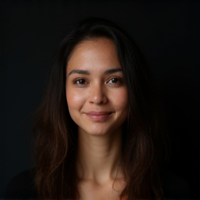
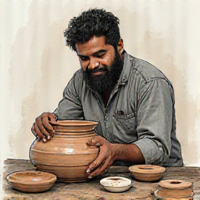
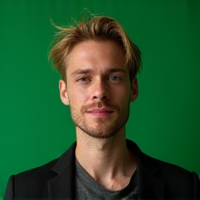
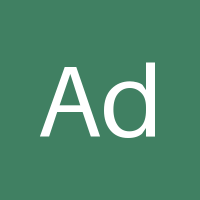
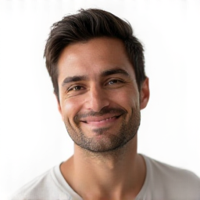
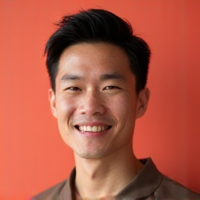
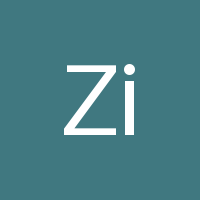
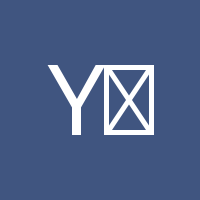
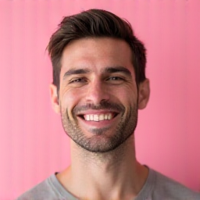
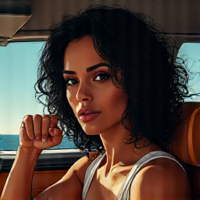
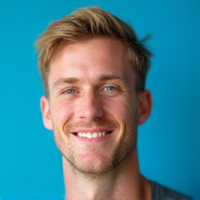
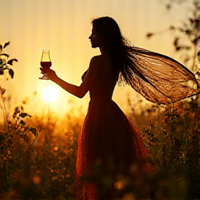
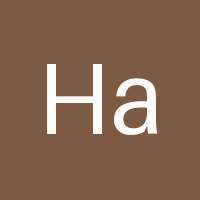
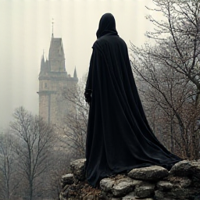
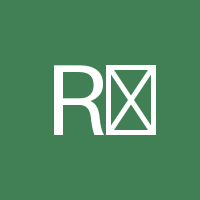
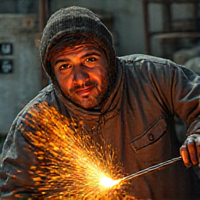
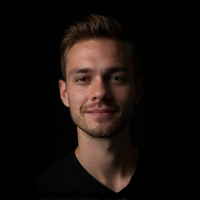
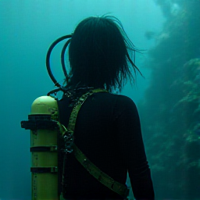
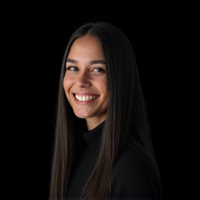
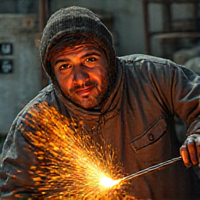
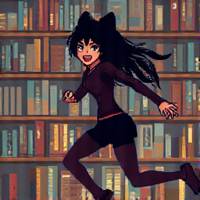
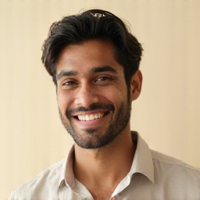
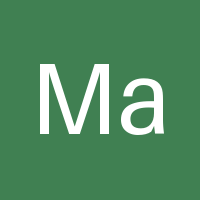
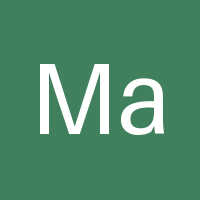
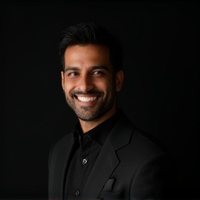
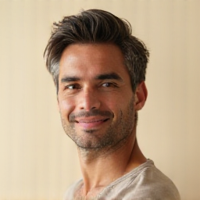
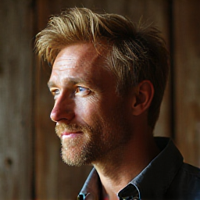
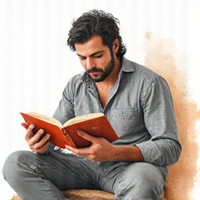
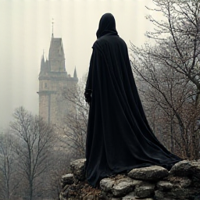
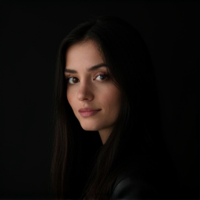
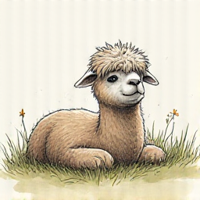
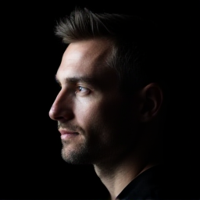
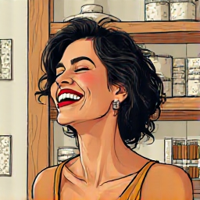
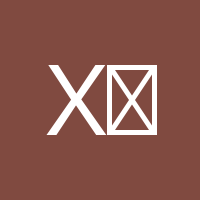
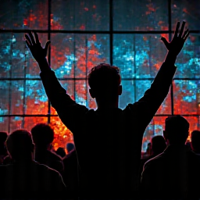
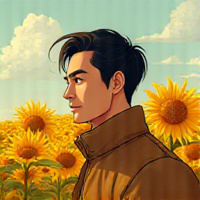
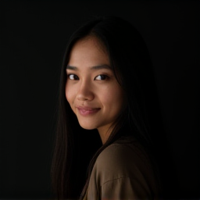
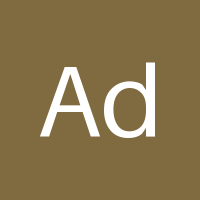
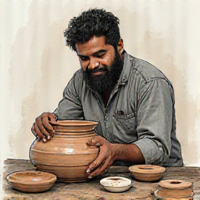
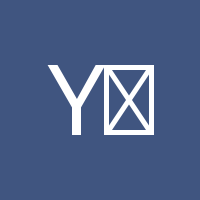
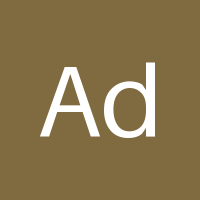
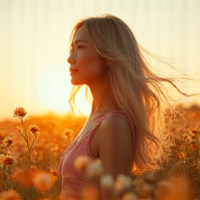
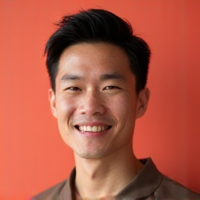
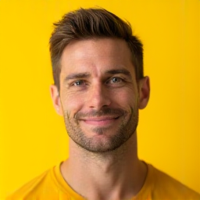
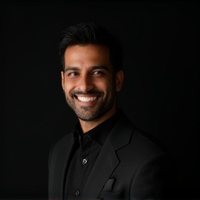
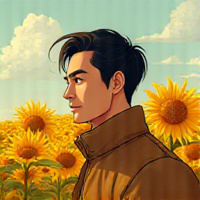
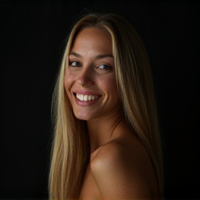
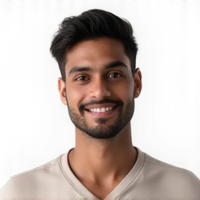
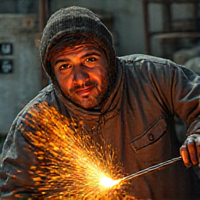
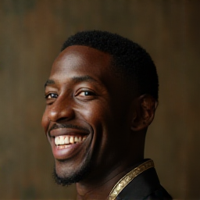
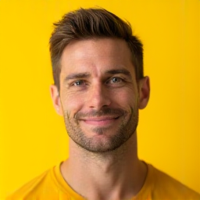
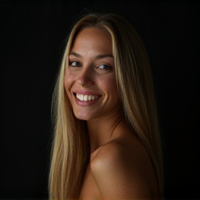
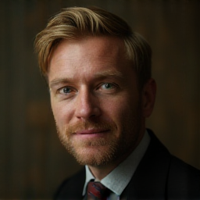
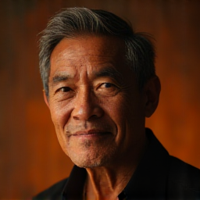
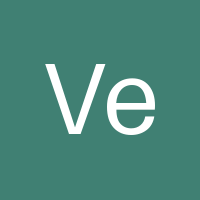
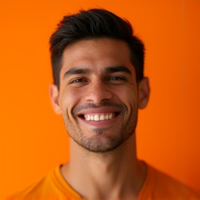
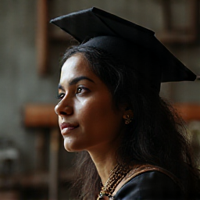
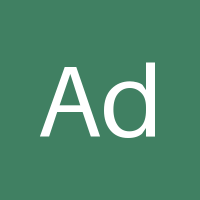
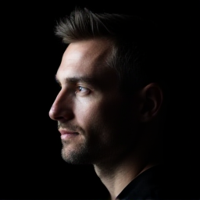
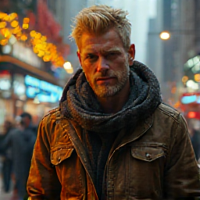
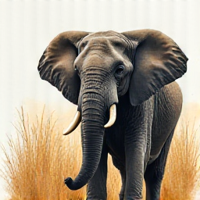
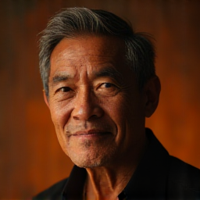
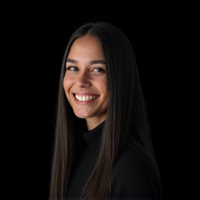
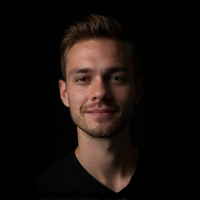
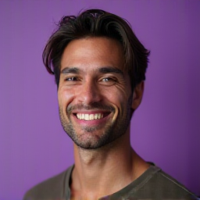
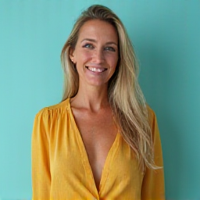
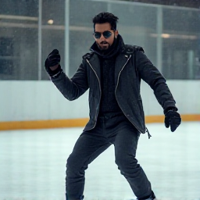
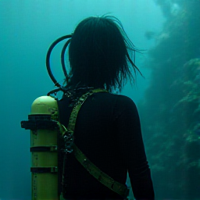
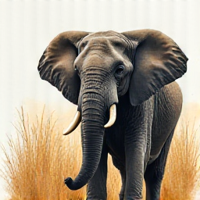
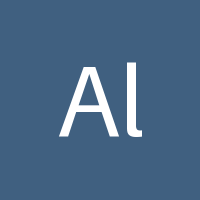
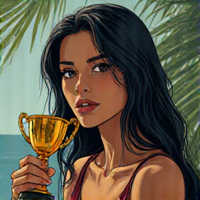
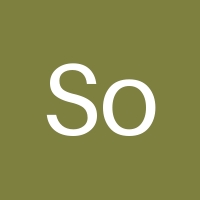
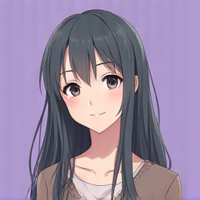
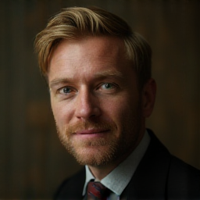
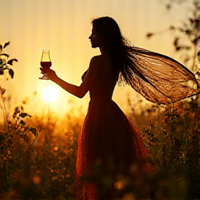
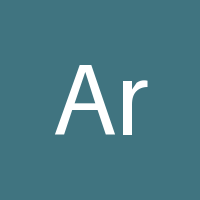
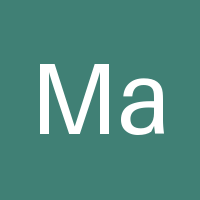
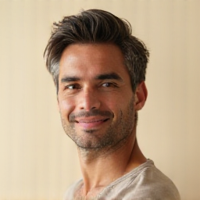
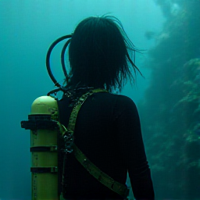
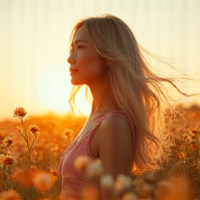