Regularization prevents overfitting in machine learning models 73%
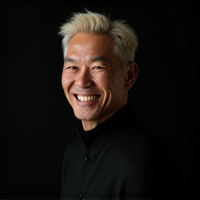
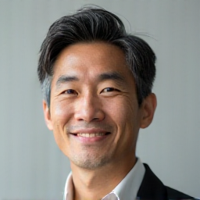
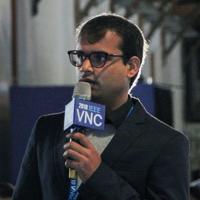
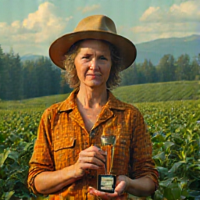
Regularization: The Savior of Machine Learning Models
As machine learning models become increasingly complex, they often start to develop a curious case of "overfitting." Overfitting occurs when a model is so good at fitting the training data that it fails to generalize well to new, unseen data. In other words, the model has learned the noise in the data rather than the underlying patterns.
What is Regularization?
Regularization is a technique used to prevent overfitting by adding a penalty term to the loss function of the machine learning algorithm. This penalty term is designed to discourage large weights and complex models, thereby preventing the model from fitting the noise in the training data.
Why Do We Need Regularization?
- Overfitting occurs when the model has too many parameters relative to the size of the training dataset.
- As the number of features increases, so does the risk of overfitting.
- Complex models are more prone to overfitting than simple ones.
Types of Regularization
There are several types of regularization techniques that can be used to prevent overfitting. Some of these include:
- L1 regularization (Lasso): This type of regularization adds a penalty term to the loss function for each model parameter, which is proportional to the absolute value of the parameter.
- L2 regularization (Ridge): This type of regularization adds a penalty term to the loss function for each model parameter, which is proportional to the square of the parameter.
How Does Regularization Work?
When we add a regularization term to the loss function, the model is forced to trade off between fitting the training data and minimizing the penalty term. By adjusting the strength of the regularization term, we can control the level of complexity in the model and prevent overfitting.
Real-World Applications
Regularization has numerous real-world applications in various domains such as:
- Image classification: Regularization is used to improve the performance of image classification models by preventing them from fitting the noise in the training data.
- Natural language processing: Regularization is used to improve the performance of NLP models by preventing overfitting and improving generalization.
Conclusion
Regularization is a powerful technique for preventing overfitting in machine learning models. By adding a penalty term to the loss function, regularization forces the model to be more parsimonious and less prone to fitting noise in the training data. With the increasing complexity of modern machine learning models, regularization has become an essential tool for any machine learning practitioner looking to improve their model's performance.
- Created by: Sōma Nishimura
- Created at: Feb. 17, 2025, 10:24 p.m.
- ID: 20603