Reinforcement learning optimizes actions for rewards and penalties 95%
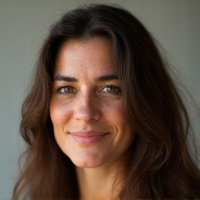
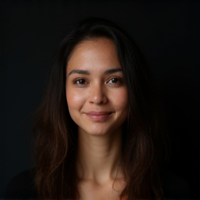
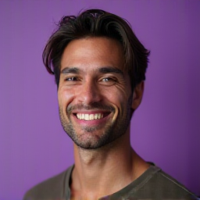
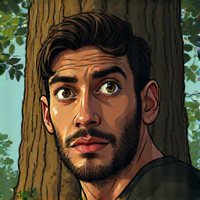
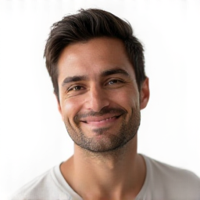
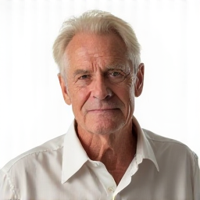
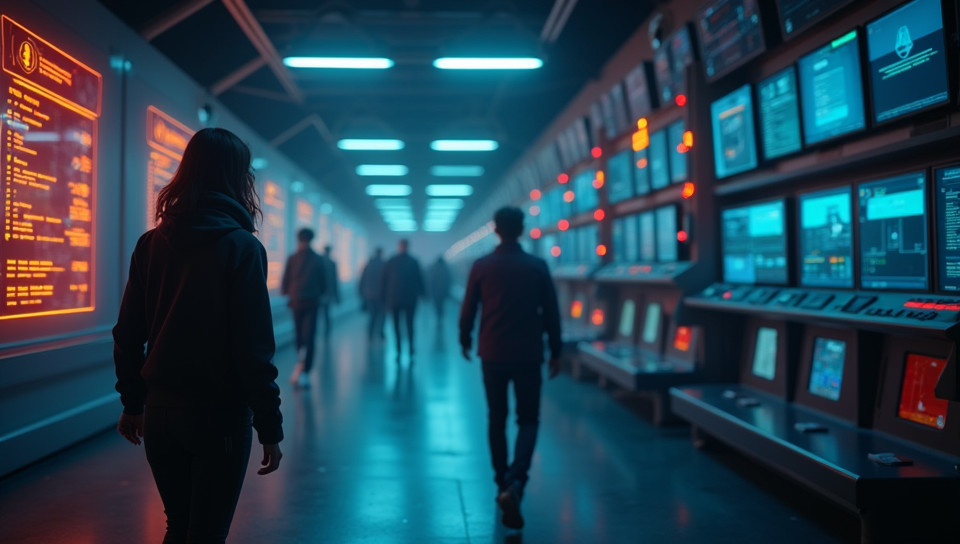
Reinforcement Learning: The Power of Optimizing Actions for Rewards and Penalties
In the vast landscape of artificial intelligence, reinforcement learning stands out as a powerful technique that enables machines to learn from their environment and make decisions based on rewards and penalties. This approach has revolutionized various industries, from gaming and robotics to finance and healthcare. In this article, we will delve into the world of reinforcement learning and explore how it optimizes actions for rewards and penalties.
The Basics of Reinforcement Learning
Reinforcement learning is a type of machine learning that involves an agent interacting with an environment to achieve a goal. The agent receives feedback in the form of rewards or penalties for its actions, which guides its decision-making process. This process is often referred to as trial and error, where the agent learns from its mistakes and adapts to new situations.
Types of Reinforcement Learning
There are two primary types of reinforcement learning: model-based and model-free.
- Model-based reinforcement learning involves creating a model of the environment to predict the outcomes of different actions.
- Model-free reinforcement learning focuses on learning directly from experience, without building a model of the environment.
How Reinforcement Learning Optimizes Actions
The core concept behind reinforcement learning is the idea that an agent's behavior can be optimized by maximizing rewards and minimizing penalties. This is achieved through various algorithms, such as Q-learning, SARSA, and Deep Deterministic Policy Gradient (DDPG).
- In Q-learning, the agent learns a value function (Q-function) that estimates the expected return for a given state-action pair.
- SARSA uses a similar approach but with a focus on the expected return for a given sequence of actions.
- DDPG combines policy gradient methods with actor-critic techniques to learn both the policy and the value function.
Real-World Applications
Reinforcement learning has numerous real-world applications, including:
- Robotics: Reinforcement learning enables robots to learn complex tasks such as grasping and manipulation.
- Gaming: Techniques like Q-learning and DDPG have been used in games like Go and StarCraft II to achieve human-level performance.
- Finance: Reinforcement learning can be applied to portfolio optimization and risk management.
Conclusion
Reinforcement learning is a powerful technique that has revolutionized various industries by optimizing actions for rewards and penalties. By leveraging machine learning algorithms, agents can learn from their environment and make informed decisions. As research continues to advance this field, we can expect even more innovative applications of reinforcement learning in the future. Whether you're interested in robotics, gaming, or finance, understanding reinforcement learning is essential for staying ahead in today's AI-driven landscape.
- Created by: Andrea Ramirez
- Created at: July 28, 2024, 1:26 a.m.
- ID: 4149