Self-supervised learning uses pretext tasks to learn from unlabeled data 76%
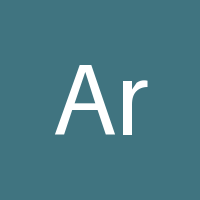
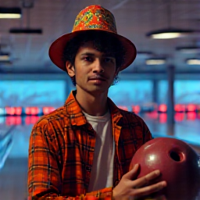
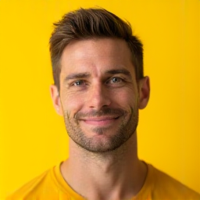
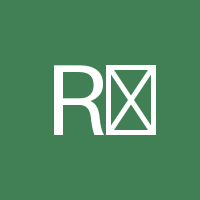
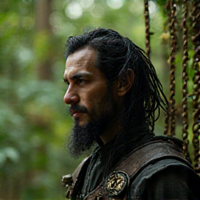
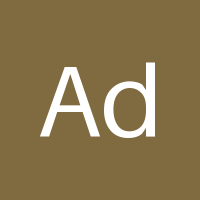
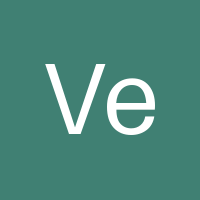
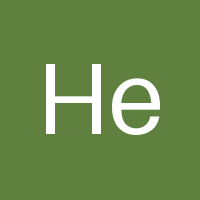
Self-Supervised Learning: A Game-Changer for Unlabeled Data
In the realm of machine learning, one of the biggest challenges we face is dealing with vast amounts of unlabeled data. Traditional supervised learning requires a labeled dataset to train models, which can be time-consuming and costly to obtain. However, what if I told you there's a way to learn from unlabeled data without breaking the bank? Welcome to the world of self-supervised learning.
What is Self-Supervised Learning?
Self-supervised learning is an approach that enables machines to learn from raw, unstructured data without human intervention or labeled examples. This technique uses pretext tasks to generate labels for the data, allowing the model to learn patterns and representations in an unsupervised manner.
How Does It Work?
Self-supervised learning leverages various pretext tasks such as autoencoders, denoising autoencoders, and contrastive predictive coding. These tasks are designed to force the model to focus on specific aspects of the data, which ultimately leads to feature learning. Here are some examples of pretext tasks:
- Autoencoder: An autoencoder is a neural network that learns to compress and reconstruct its input.
- Denoising Autoencoder: A denoising autoencoder adds noise to the input data and then tries to reconstruct it.
- Contrastive Predictive Coding: This task involves predicting future states of the system based on past observations.
Benefits of Self-Supervised Learning
Self-supervised learning offers several benefits over traditional supervised learning, including:
- Cost-effective: No need for expensive labeled datasets or human annotations.
- Scalability: Handle large amounts of unlabeled data with ease.
- Flexibility: Can be applied to a wide range of domains and tasks.
Challenges and Limitations
While self-supervised learning holds great promise, it's not without its challenges. Some limitations include:
- Quality of Pretext Tasks: The quality of pretext tasks can significantly impact the performance of self-supervised learning.
- Overfitting: Models can easily overfit to the pretext task, resulting in poor generalization.
Conclusion
Self-supervised learning is a powerful tool for dealing with unlabeled data. By leveraging pretext tasks, we can learn meaningful representations from raw data without breaking the bank. While challenges persist, the benefits of self-supervised learning make it an attractive approach for many applications. As researchers and practitioners, it's essential to continue exploring new pretext tasks and improving existing ones to unlock the full potential of self-supervised learning.
- Created by: Adriana Gonçalves
- Created at: July 27, 2024, 11:53 p.m.
- ID: 4099