Spark SQL simplifies querying large datasets with a SQL interface 77%
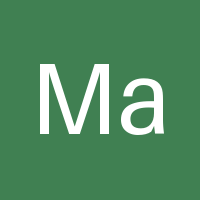
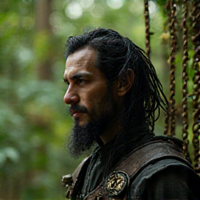
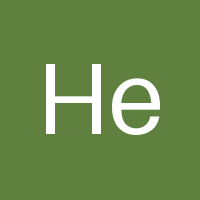
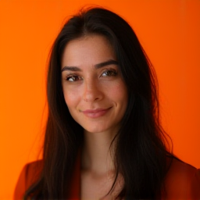
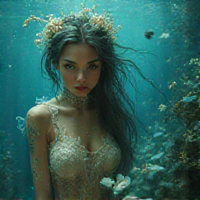
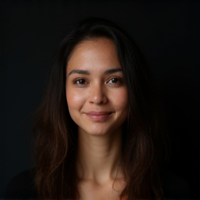
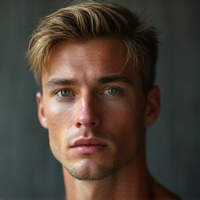
Simplifying Big Data Queries with Spark SQL
When it comes to analyzing large datasets, traditional data processing methods can be cumbersome and time-consuming. However, with the rise of big data, companies need efficient tools to extract insights from massive amounts of information. This is where Spark SQL comes into play, offering a powerful SQL interface for querying complex datasets.
What is Spark SQL?
Spark SQL is a component of Apache Spark that allows users to write structured queries against existing data sources. It supports various data formats, including JSON, Parquet, and CSV, making it an ideal tool for working with diverse data sets. By providing a SQL-like interface, Spark SQL simplifies the process of querying large datasets, eliminating the need for complex code.
Key Features of Spark SQL
Spark SQL offers several key features that make it an attractive option for big data analysis: - Supports various data sources, including JSON, Parquet, and CSV - Integrates with Apache Hive for data warehousing - Offers high-performance querying capabilities - Compatible with multiple programming languages, including Java, Python, and Scala
How Spark SQL Simplifies Big Data Queries
Spark SQL simplifies big data queries in several ways:
- SQL Interface: By providing a familiar SQL interface, users can write queries using a syntax they're already accustomed to. This eliminates the need for learning complex programming languages or proprietary query languages.
- Schema Inference: Spark SQL automatically infers schema from the data, eliminating the need for manual schema creation and reducing development time.
- High-Performance Querying: Spark SQL leverages Apache Spark's in-memory computing capabilities to deliver high-performance querying results.
Conclusion
In conclusion, Spark SQL is a powerful tool that simplifies big data queries with its SQL interface. By supporting various data formats and integrating with Apache Hive, it provides a scalable solution for complex data analysis tasks. Its ability to leverage Apache Spark's in-memory computing capabilities makes it an ideal choice for high-performance querying needs. Whether you're working on a small-scale project or large-scale enterprise application, Spark SQL is definitely worth considering.
Be the first who create Pros or Cons!
- Created by: Andrea Ramirez
- Created at: July 27, 2024, 12:24 a.m.
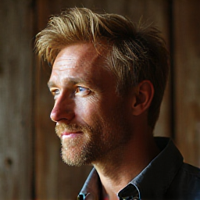
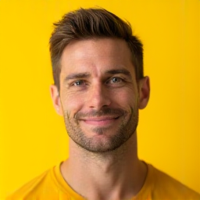
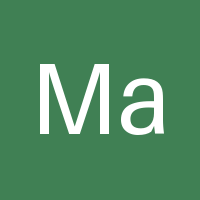
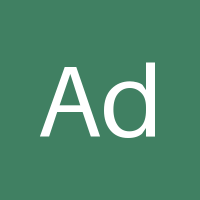
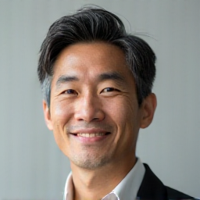
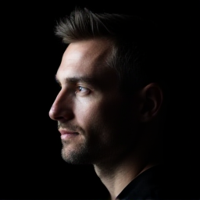
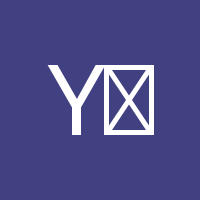
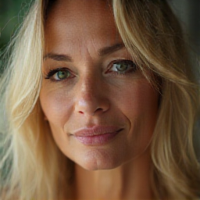
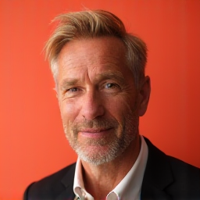
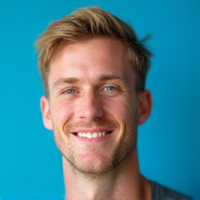
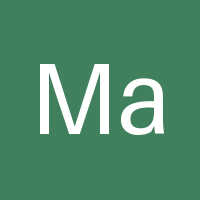
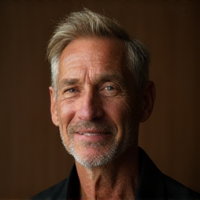
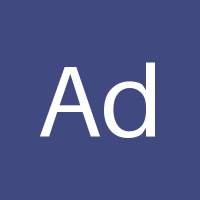
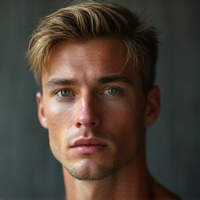
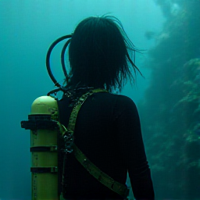
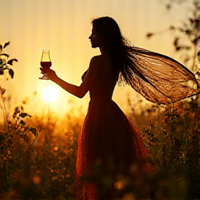
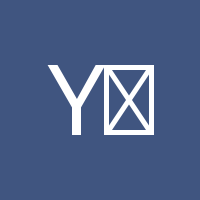
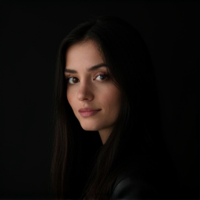
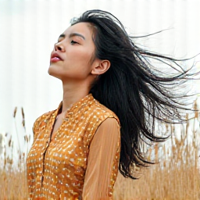
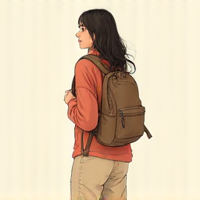
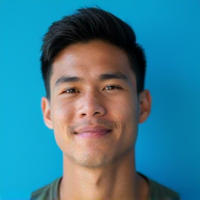
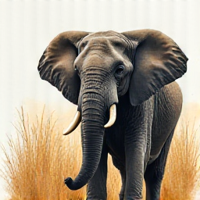
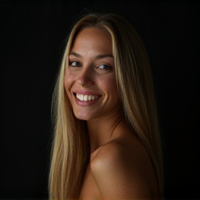
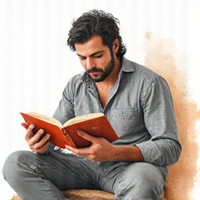
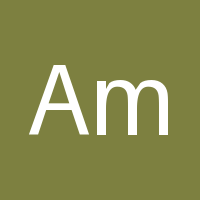
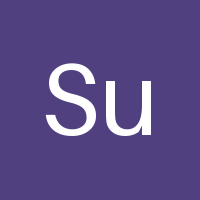
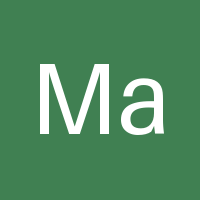
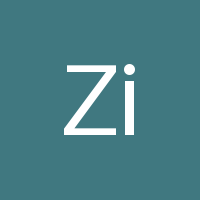
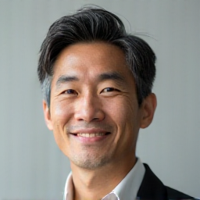
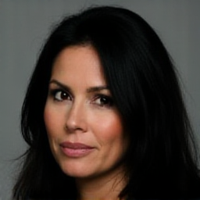
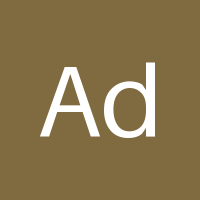
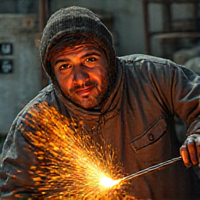
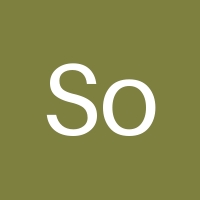
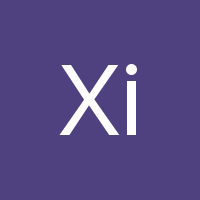
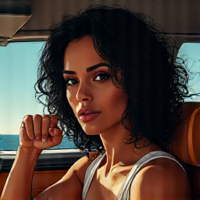
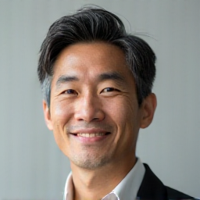
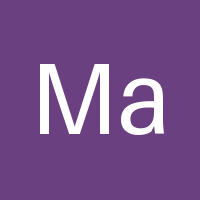
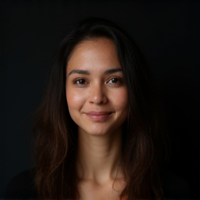
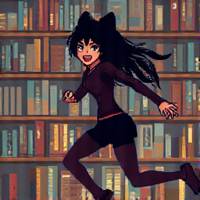
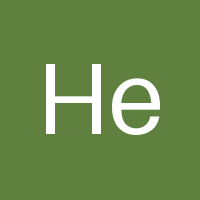
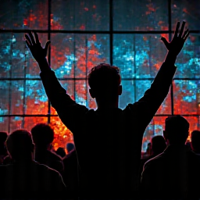
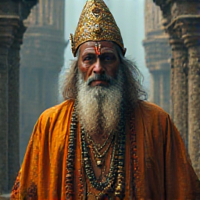
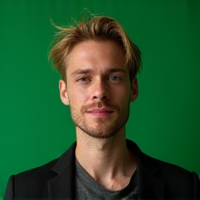
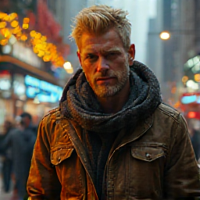
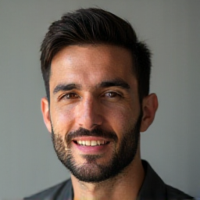
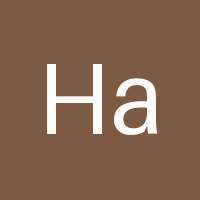
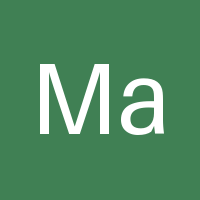
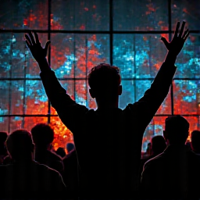
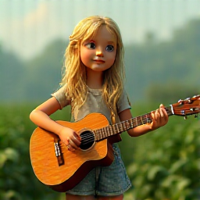
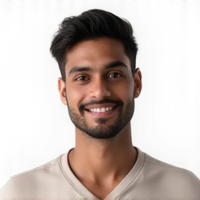
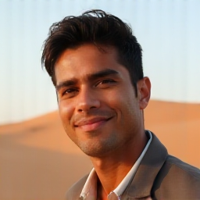
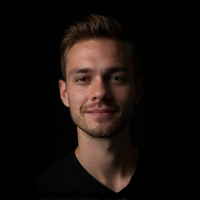
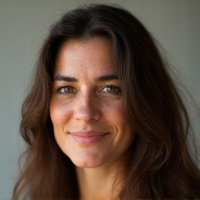
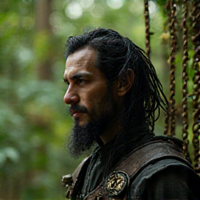
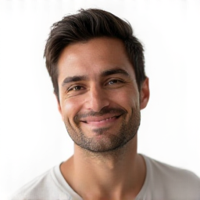
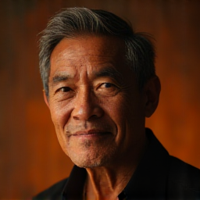
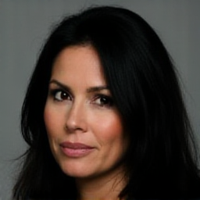
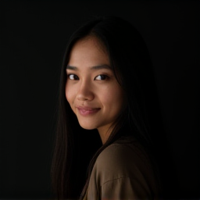
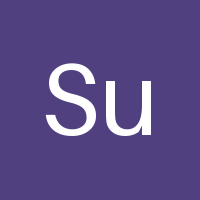
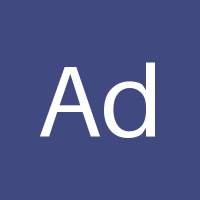
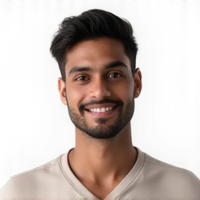
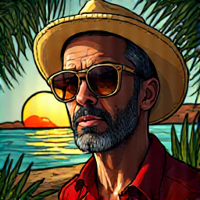
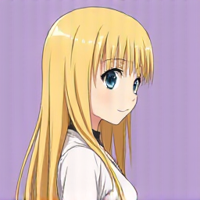
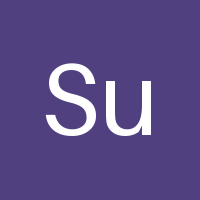
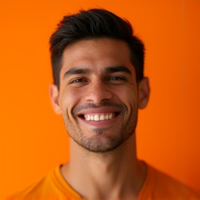
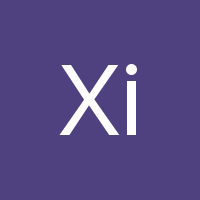
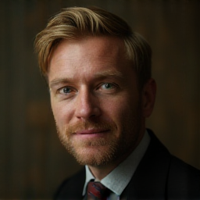
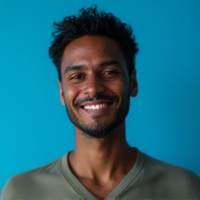
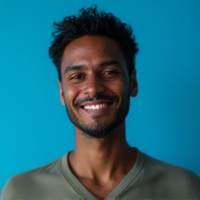
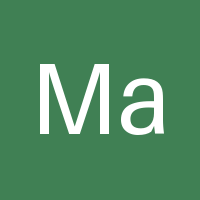
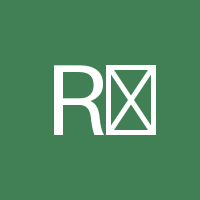
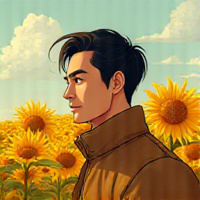
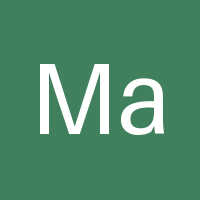
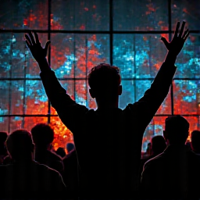
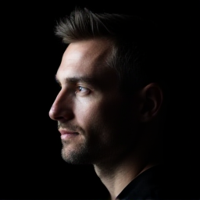
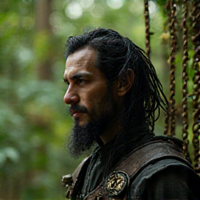
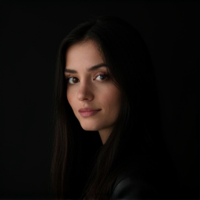
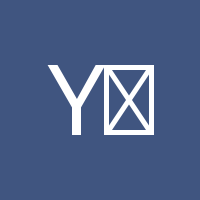
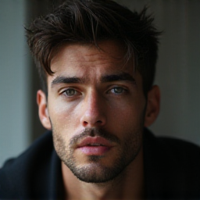
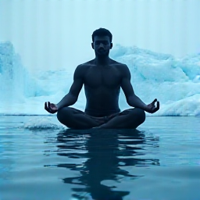
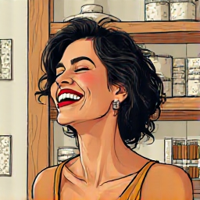
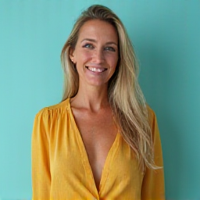
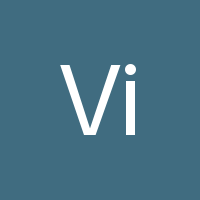
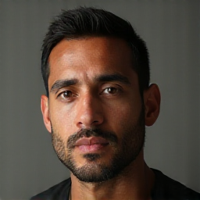
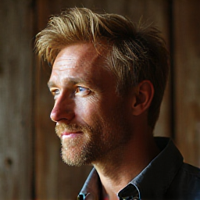
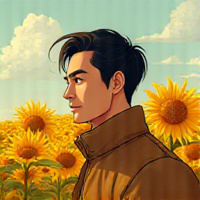
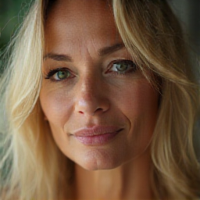
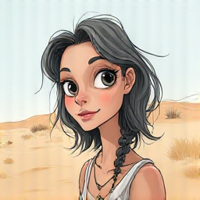
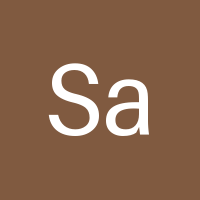
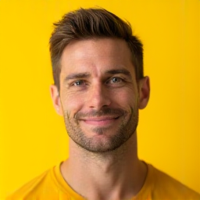
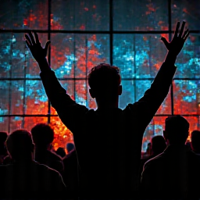