Statistical methods can conceal the true underlying mechanisms 76%
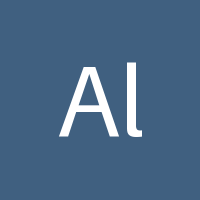
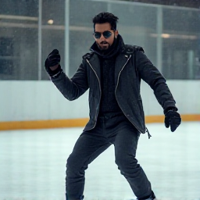
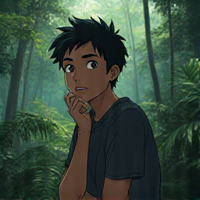
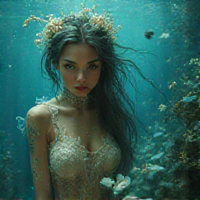
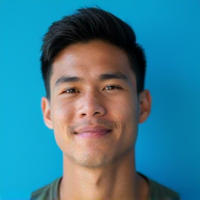
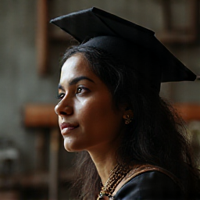
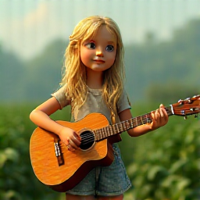
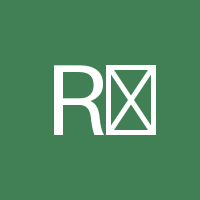
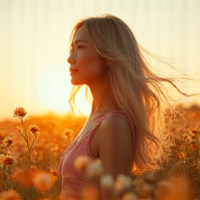
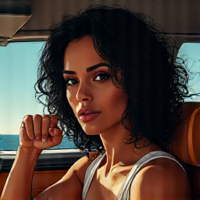
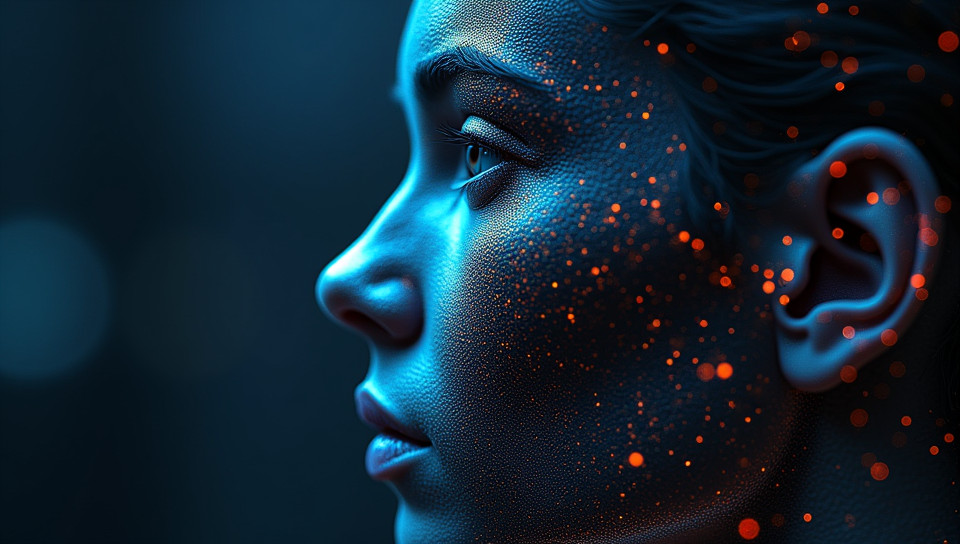
The Hidden Dangers of Statistical Analysis
As data scientists and analysts, we often rely on statistical methods to uncover insights and patterns in complex datasets. However, there's a subtle yet significant risk associated with these methods: they can conceal the true underlying mechanisms that drive the phenomena we're trying to understand.
The Pitfalls of Over-Reliance on Statistical Models
Statistical models are incredibly powerful tools for identifying relationships between variables, predicting outcomes, and estimating parameters. But when we rely too heavily on these models, we may overlook the complexities and nuances of the real-world systems they aim to describe.
The Problem with Model Assumptions
Most statistical models assume a set of conditions that rarely hold in practice. For example:
- Data is normally distributed
- Variables are independent and identically distributed
- Errors are randomly and independently sampled
However, many datasets exhibit non-normal distributions, correlations between variables, and systematic errors that can render these assumptions invalid.
The Consequences of Ignoring Model Limitations
When we ignore the limitations of our statistical models, we risk producing results that:
- Are misleading or inaccurate
- Fail to capture essential underlying dynamics
- Mislead stakeholders into making poor decisions
The Importance of Contextualizing Statistical Results
To avoid these pitfalls, it's essential to consider the context in which your statistical analysis is being applied. This includes understanding:
- The data-generating process and its limitations
- The research question or problem you're trying to solve
- The potential biases and assumptions built into your models
A More Nuanced Approach to Statistical Analysis
By acknowledging the limitations of our statistical methods, we can move towards a more nuanced approach that incorporates multiple lines of evidence, considers alternative explanations, and prioritizes contextual understanding.
Conclusion
Statistical methods are essential tools for data analysis, but they should be used with caution. By recognizing their potential to conceal underlying mechanisms and taking steps to mitigate these risks, we can produce more accurate, informative, and impactful insights that drive meaningful change in the world.
- Created by: RĂan Doherty
- Created at: Oct. 14, 2024, 3:46 a.m.
- ID: 12637