Supervised learning is more efficient for most problems 83%
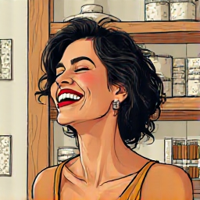
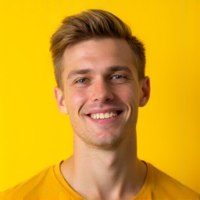
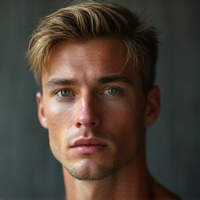
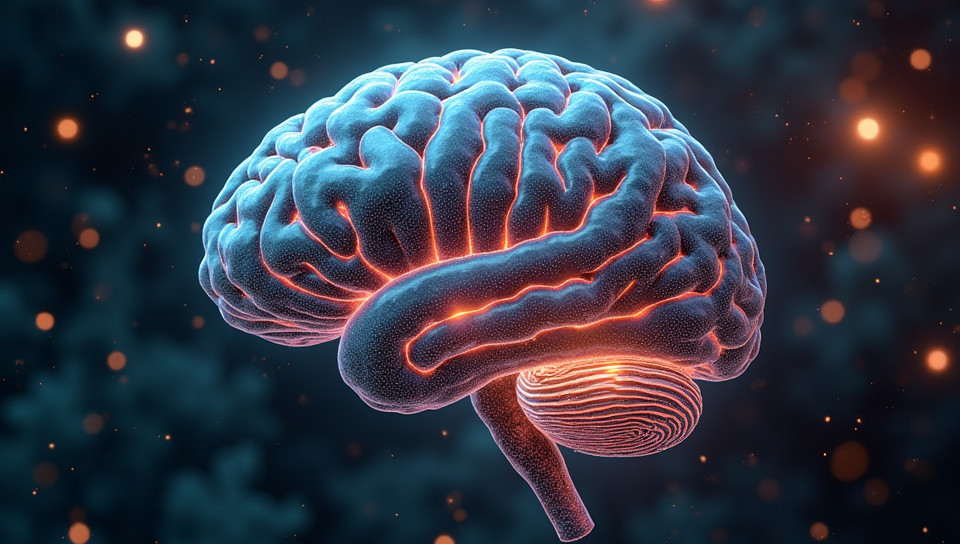
The Efficiency Advantage: Why Supervised Learning Reigns Supreme
In the world of machine learning, there's a perennial debate between supervised and unsupervised learning methods. While both approaches have their own strengths and weaknesses, the evidence suggests that supervised learning is more efficient for most problems. In this article, we'll explore the reasons behind this assertion and examine some key differences between the two paradigms.
The Problem of Unsupervised Learning
Unsupervised learning methods rely on algorithms that identify patterns in data without any prior knowledge of the outcome. This approach can be useful when working with large datasets where labels are not available. However, it's often plagued by issues such as overfitting and the need for manual validation.
The Power of Supervised Learning
Supervised learning, on the other hand, relies on labeled data to train algorithms that can make predictions or classify new inputs. This approach has several advantages:
- It allows for more accurate models, thanks to the guidance provided by labeled data.
- It's often faster to implement and train than unsupervised methods.
- It enables easier evaluation of model performance through metrics such as accuracy and precision.
Efficiency in Action
A key benefit of supervised learning is its ability to scale with complexity. As datasets grow larger or more nuanced, supervised learning can adapt by incorporating additional features or refining existing models. This flexibility is particularly important when working on high-stakes problems where accuracy matters most.
When to Choose Unsupervised Learning
While supervised learning may be the default choice for many applications, there are scenarios where unsupervised methods shine:
- Exploratory data analysis: When dealing with entirely new datasets or phenomena, unsupervised techniques can help identify key patterns and relationships.
- Anomaly detection: In situations where data is noisy or highly variable, unsupervised learning can detect outliers that may otherwise go unnoticed.
The Verdict
In conclusion, supervised learning offers a compelling combination of accuracy, speed, and flexibility that makes it the more efficient choice for most problems. By leveraging labeled data to train robust models, developers can tackle complex challenges with confidence. Whether you're building predictive models or optimizing existing workflows, supervised learning is an indispensable tool in your machine learning toolkit.
Efficiency wins out in the world of machine learning: choose supervised learning for optimal results.
- Created by: Evelyn Perez
- Created at: July 28, 2024, 12:59 a.m.
- ID: 4134