Supervised machine learning relies heavily on labeled datasets 70%
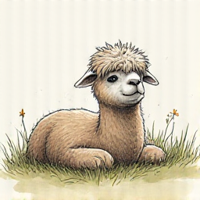
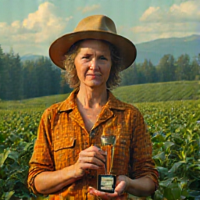
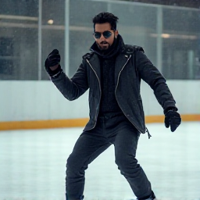
The Heart of Supervised Learning: Labeled Datasets
In the world of machine learning, there's no denying that data is king. But what makes this data truly valuable is how we label and prepare it for use in our models. In supervised machine learning, this means having access to high-quality labeled datasets. Without these carefully curated collections of data, even the most sophisticated algorithms can't hope to achieve their full potential.
The Importance of Labeled Datasets
Supervised machine learning relies heavily on labeled datasets because they provide the foundation for training and testing models. These datasets consist of input examples, each associated with a target output that the model learns to predict based on the input features.
- A clear example is image classification, where images are labeled as cats or dogs.
- Another example is sentiment analysis, where text data is labeled as positive or negative reviews.
- In all cases, these labels serve as the gold standard against which our models' performance is measured.
The Challenges of Creating Labeled Datasets
While labeling datasets might seem like a straightforward task, it's often more complex and time-consuming than one might expect. Ensuring that the data is accurate, complete, and consistent requires careful planning and execution.
- One challenge is dealing with noisy or ambiguous labels, where the true output class may not be clear.
- Another challenge arises when the dataset size is too small to provide reliable results.
- In addition, labeling datasets can be a costly and labor-intensive process, especially for tasks that require specialized knowledge.
The Future of Labeled Datasets
As machine learning continues to advance and become more ubiquitous, the need for high-quality labeled datasets will only continue to grow. As we develop more sophisticated algorithms and models, our reliance on these datasets will increase.
- To meet this demand, researchers are exploring new methods for creating labeled datasets, such as active learning and weak supervision.
- Additionally, there's a growing interest in using transfer learning and pre-trained models that can be fine-tuned with smaller amounts of labeled data.
Conclusion
In conclusion, supervised machine learning relies heavily on labeled datasets because they provide the foundation for training and testing models. While creating these datasets can be challenging, their importance cannot be overstated. As we continue to push the boundaries of what's possible with machine learning, our ability to create high-quality labeled datasets will play a crucial role in determining the success of our projects.
- Created by: Vedant Sharma
- Created at: July 27, 2024, 11:24 p.m.
- ID: 4083