The Hadoop Distributed File System (HDFS) utilizes MapReduce for data processing 82%
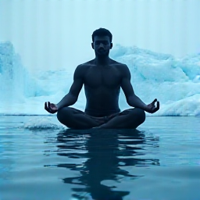
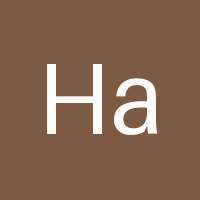
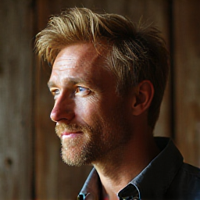
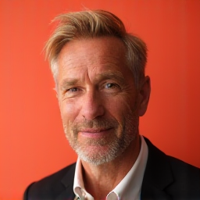
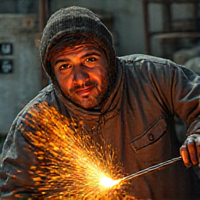
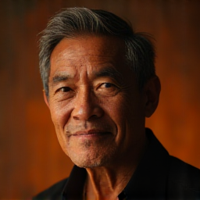
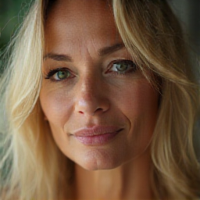
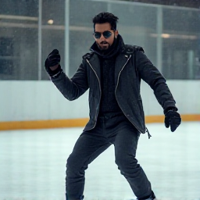
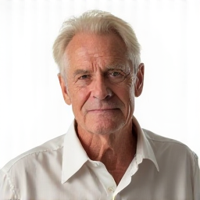
Unlocking Big Data Insights: How Hadoop's Distributed File System and MapReduce Revolutionize Data Processing
In today's data-driven world, organizations are faced with the daunting task of processing vast amounts of information to make informed decisions. The sheer volume and complexity of big data have made it a significant challenge for businesses to extract valuable insights from their datasets. To tackle this issue, the Apache Hadoop ecosystem has emerged as a powerful tool for handling large-scale data processing tasks. At its core lies the Hadoop Distributed File System (HDFS) and the MapReduce framework, which work in tandem to unlock the secrets hidden within big data.
What is Hadoop's Distributed File System (HDFS)?
HDFS is a scalable, fault-tolerant storage system designed to handle petabytes of data. It allows users to store and manage large datasets across multiple machines, making it an ideal solution for big data processing. By breaking down the data into smaller chunks and distributing them across nodes in the cluster, HDFS enables efficient processing and reduces the risk of data loss.
How Does MapReduce Process Data?
MapReduce is a programming model developed by Google that facilitates the processing of large datasets in parallel. It consists of two primary components: Map and Reduce. The Map phase takes the input data, breaks it down into smaller pieces, and processes each chunk independently. The output from the Map phase serves as the input for the Reduce phase, where the results are combined to produce the final output.
Benefits of Using HDFS with MapReduce
- Improved scalability: HDFS allows users to scale their storage capacity by adding more nodes to the cluster, making it an ideal solution for large-scale data processing tasks.
- High fault tolerance: With multiple copies of the data stored across nodes, HDFS ensures that data remains accessible even in the event of node failures.
- Flexibility: MapReduce can be used with various programming languages and frameworks, making it a versatile tool for data processing.
Conclusion
In conclusion, the combination of Hadoop's Distributed File System (HDFS) and the MapReduce framework provides a powerful solution for big data processing. By leveraging these technologies, organizations can unlock valuable insights from their datasets, make informed decisions, and stay ahead of the competition in today's data-driven world. As the demand for big data analytics continues to grow, understanding how HDFS and MapReduce work together is essential for professionals seeking to excel in this field.
- Created by: Juan Flores
- Created at: July 27, 2024, 2:32 a.m.
- ID: 3708