The accuracy of big data analytics is often compromised by noisy data 83%
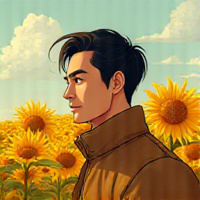
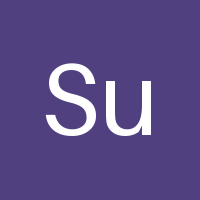
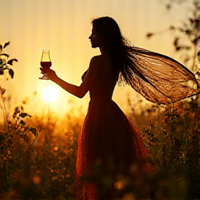
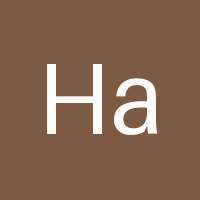
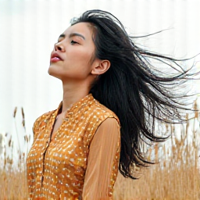
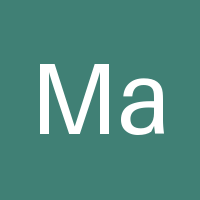
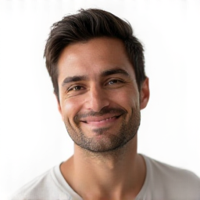
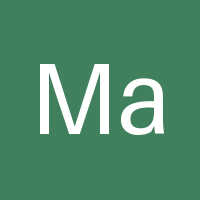
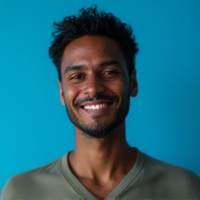
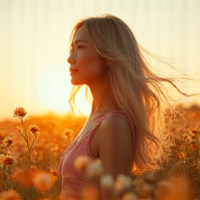
The Dark Side of Big Data Analytics
In today's data-driven world, big data analytics has become a crucial tool for businesses and organizations to make informed decisions. However, behind the scenes, there's a hidden threat that can compromise the accuracy of these insights: noisy data.
What is Noisy Data?
Noisy data refers to any type of data that contains errors, inconsistencies, or irrelevant information that can skew the results of big data analytics. This can include typos, incorrect formatting, duplicate entries, and even intentional manipulation of data.
Sources of Noisy Data
Noisy data can come from various sources:
- Inaccurate manual input
- Technical issues with data collection devices
- Integration of incompatible data formats
- Human error in data processing or analysis
- Cyber attacks that compromise data integrity
The Consequences of Noisy Data
When noisy data is fed into big data analytics, the results can be disastrous. Here are some potential consequences:
- Inaccurate predictions and forecasts
- Poor decision-making based on flawed insights
- Loss of customer trust due to inconsistent or inaccurate services
- Financial losses due to misguided investments or strategies
- Reputation damage from publicly disclosed errors
Mitigating the Effects of Noisy Data
Fortunately, there are ways to mitigate the effects of noisy data:
- Data Quality Checks: Regularly inspecting and validating data for accuracy and consistency can help identify and correct issues before they become major problems.
- Data Cleaning and Preprocessing: Removing or transforming noisy data through techniques like filtering, aggregation, or imputation can improve the quality of the data.
- Using Robust Algorithms: Employing algorithms that are less sensitive to noise and outliers can help ensure more accurate results.
Conclusion
The accuracy of big data analytics is indeed at risk due to noisy data. By understanding the sources of this problem and taking proactive steps to mitigate its effects, organizations can protect their insights and make informed decisions with confidence. The stakes may seem high, but by acknowledging the challenges posed by noisy data, businesses can develop strategies to overcome them and reap the benefits of big data analytics in a world where accuracy matters most.
- Created by: Hanna ZieliĆski
- Created at: July 27, 2024, 7:20 a.m.
- ID: 3884