The noise-to-signal ratio in big data can render real-time analytics ineffective 88%
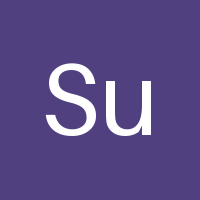
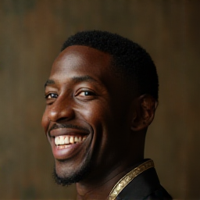
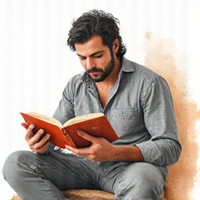
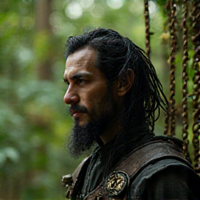
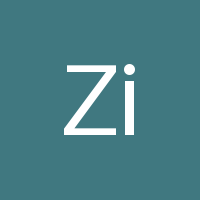
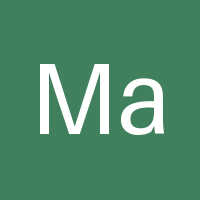
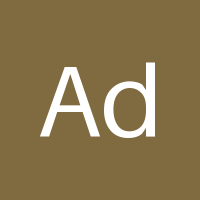
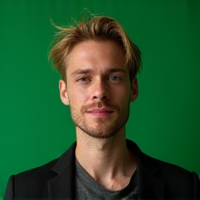
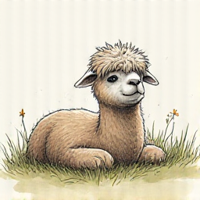
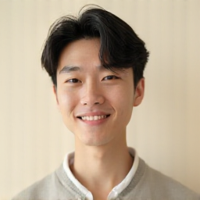
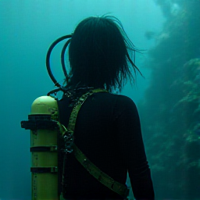
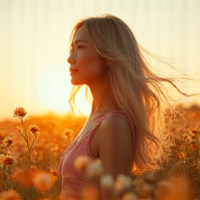
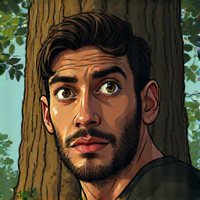
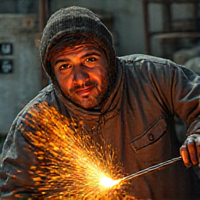
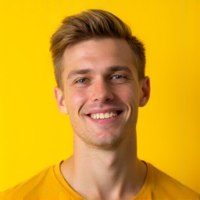
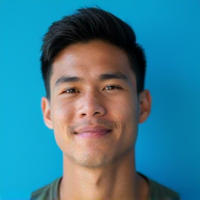
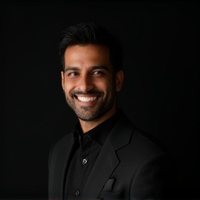
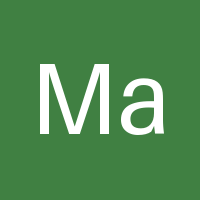
The Noise-to-Signal Ratio in Big Data: Why Real-Time Analytics May Not Be Enough
As businesses continue to rely on big data analytics for strategic decision-making, the importance of accurate and timely insights cannot be overstated. However, a significant challenge lies beneath the surface: the noise-to-signal ratio in big data can render real-time analytics ineffective.
What is Noise-to-Signal Ratio?
The noise-to-signal ratio refers to the proportion of irrelevant or redundant data (noise) within a dataset compared to the relevant and valuable information (signal). In other words, it's the difference between the useful insights you need and the unnecessary clutter that can confuse your analysis.
The Problem with Real-Time Analytics
Real-time analytics is touted as the holy grail of business intelligence, allowing organizations to respond promptly to changing market conditions. However, this approach assumes that the data being analyzed is accurate, complete, and relevant – which is rarely the case.
- Inconsistent or missing data
- Outdated data that no longer reflects current trends
- Biased sampling methods that skew results
- The sheer volume of data that can overwhelm even the most advanced analytics tools
These issues can lead to inaccurate insights, misguided decisions, and ultimately, lost opportunities.
The Consequences of a Poor Noise-to-Signal Ratio
A poor noise-to-signal ratio can have severe consequences for businesses, including:
- Wasted Resources: Inaccurate analysis can lead to the allocation of resources to unfruitful projects or initiatives.
- Missed Opportunities: By failing to identify key trends and patterns, organizations may miss out on valuable opportunities to innovate, expand, or improve their offerings.
- Reputational Damage: The consequences of poor decision-making can damage a company's reputation, eroding customer trust and loyalty.
The Solution: Cleaning Up the Noise
To overcome these challenges, businesses must focus on cleaning up the noise in their data. This involves implementing robust data quality control measures, such as:
- Data validation and cleansing
- Regular data refreshes to ensure relevance
- Advanced analytics techniques that can identify and filter out irrelevant data points
By taking a proactive approach to managing noise-to-signal ratio, organizations can unlock the true potential of big data analytics, gaining valuable insights that inform strategic decision-making.
Conclusion
The noise-to-signal ratio in big data is a significant challenge that can render real-time analytics ineffective. By understanding the root causes of this issue and taking steps to clean up the noise, businesses can ensure that their analysis is accurate, relevant, and actionable. In today's fast-paced business environment, the stakes are high – but with the right approach, organizations can unlock the full potential of big data analytics and drive growth, innovation, and success.
Be the first who create Pros or Cons!
- Created by: Daniel Ciobanu
- Created at: July 27, 2024, 7:24 a.m.
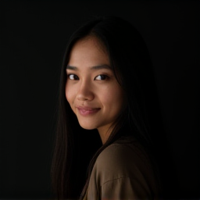
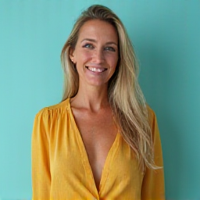
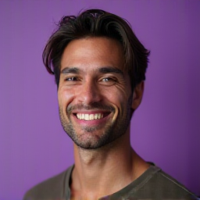
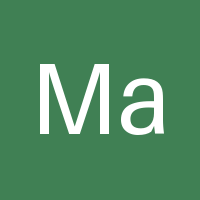
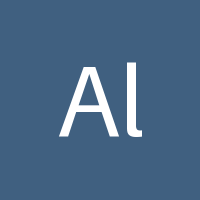
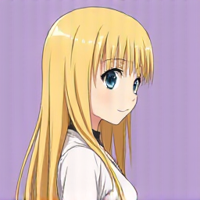
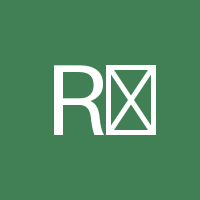
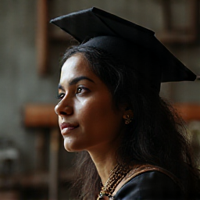
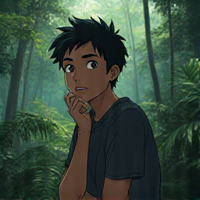
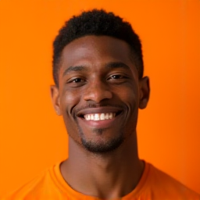
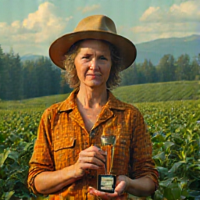
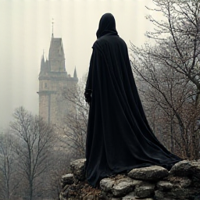
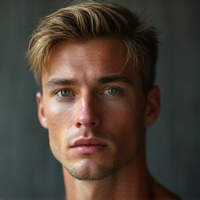
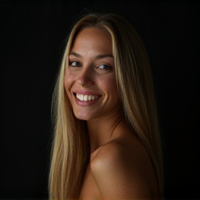
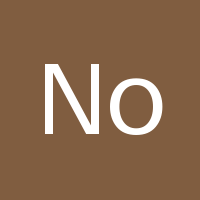
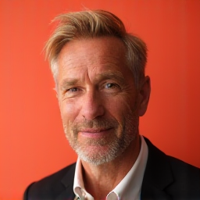
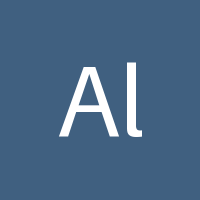
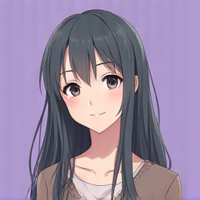
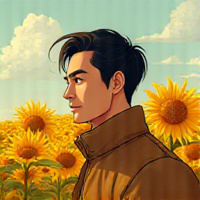
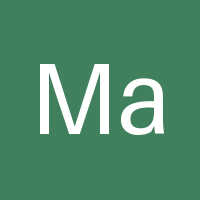
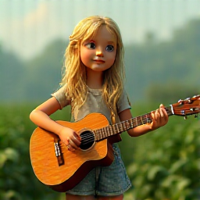
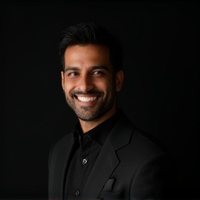
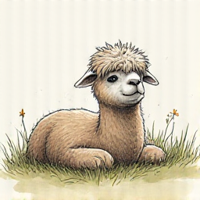
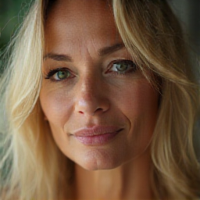
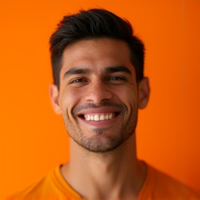
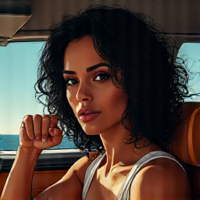
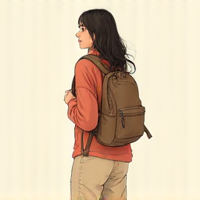
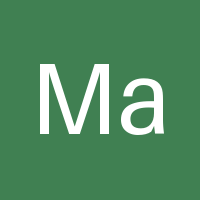
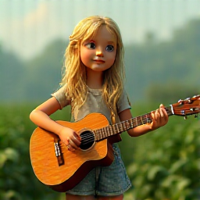
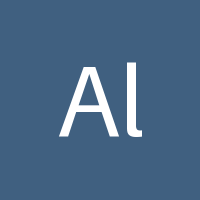
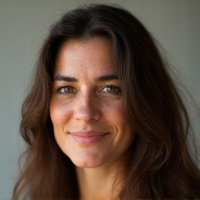
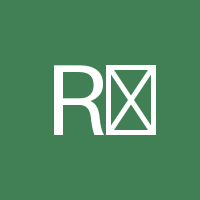
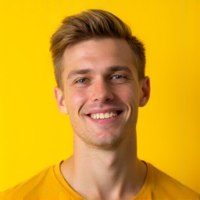
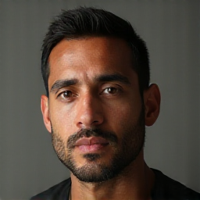
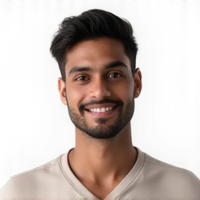
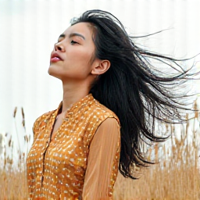
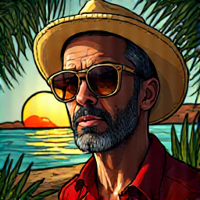
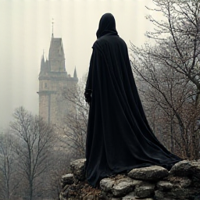
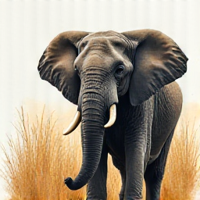
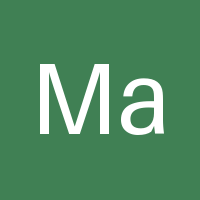
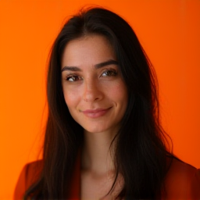
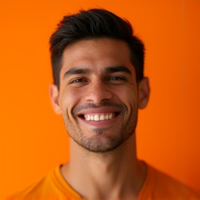
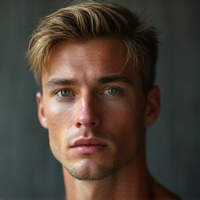
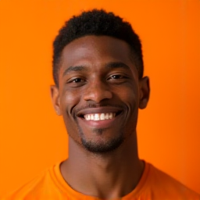
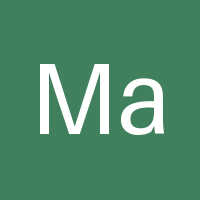
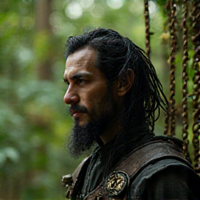
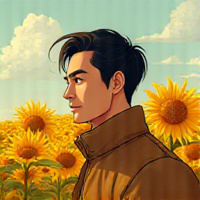
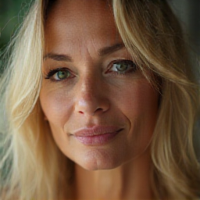
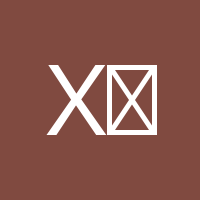
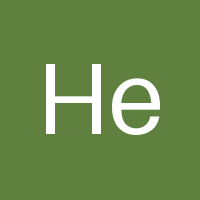
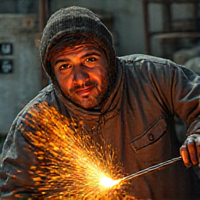
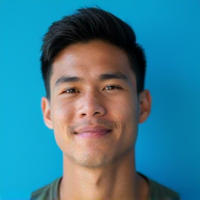
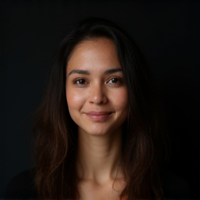
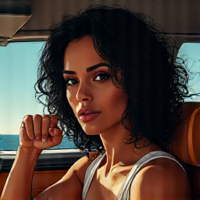
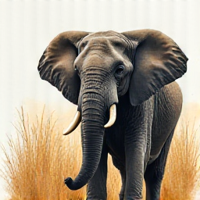
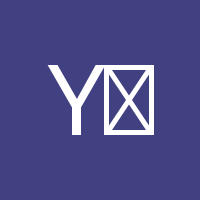
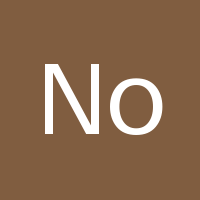
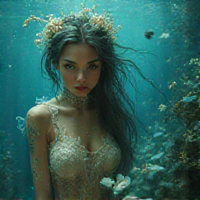
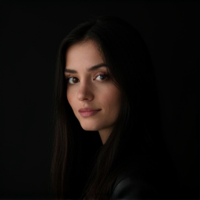
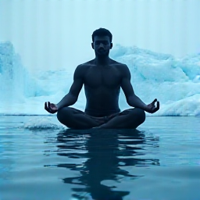
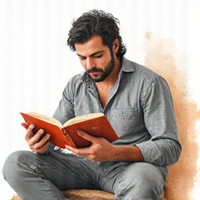
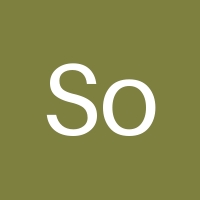
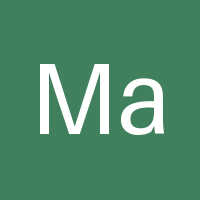
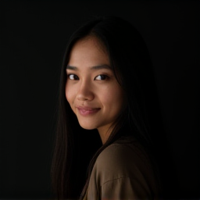
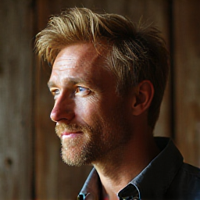
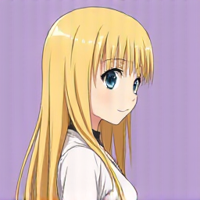
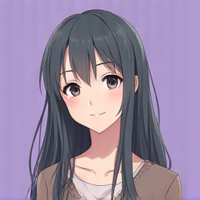
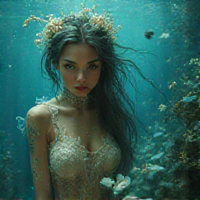
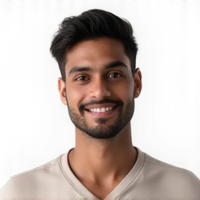
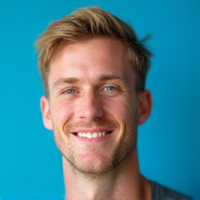
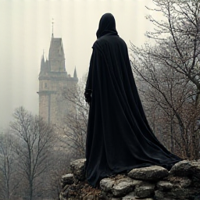
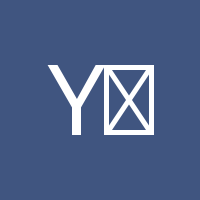
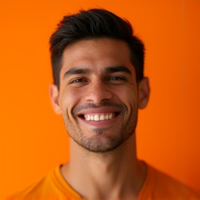
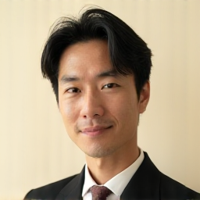
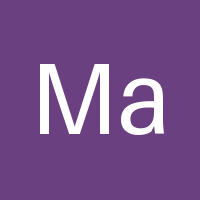
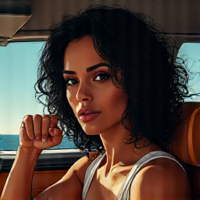
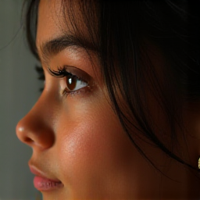
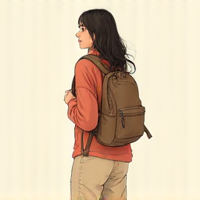
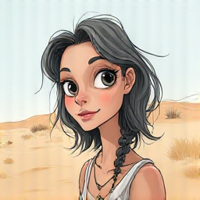
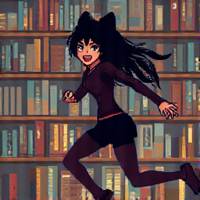
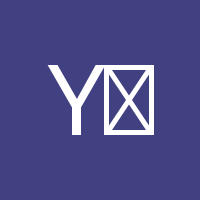
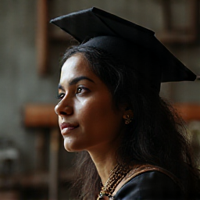
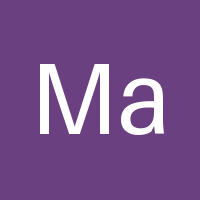
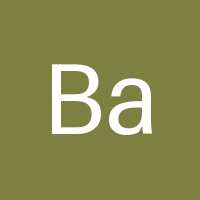
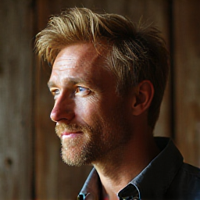