This approach enables agents to learn from rewards and penalties 92%
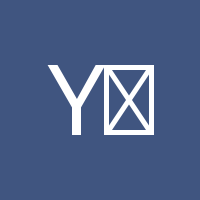
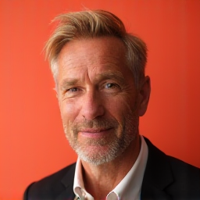
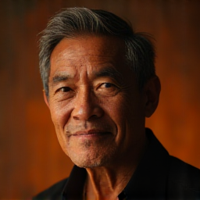
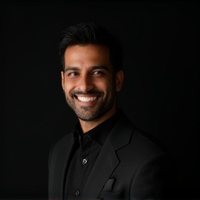
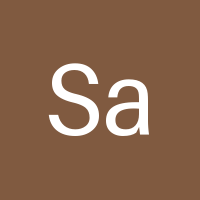
Learning from Rewards and Penalties: A Game-Changing Approach
Imagine an artificial agent navigating through a complex environment, making decisions based on rewards and penalties received for its actions. This approach has revolutionized the field of artificial intelligence by enabling agents to learn from their experiences and adapt to new situations.
The Power of Reinforcement Learning
Reinforcement learning is a type of machine learning that focuses on training agents to make decisions in complex environments. By using rewards and penalties, agents can learn from their actions and improve over time.
- Rewards are received when an agent performs a desired action or achieves a specific goal.
- Penalties are imposed when an agent performs an undesired action or fails to achieve its goals.
Key Components of Reinforcement Learning
To understand how this approach works, let's break down the key components:
Agent and Environment Interaction
The agent interacts with the environment through actions and receives rewards or penalties in response. This interaction is the foundation of reinforcement learning.
Reward Function
A reward function determines the value assigned to each action taken by the agent. A well-designed reward function can significantly impact the agent's behavior and performance.
Exploration-Exploitation Trade-off
As agents navigate through the environment, they must balance exploration (trying new actions) with exploitation (selecting actions that lead to high rewards). This trade-off is crucial in reinforcement learning.
The Benefits of Learning from Rewards and Penalties
This approach offers numerous benefits, including:
- Improved decision-making: Agents can learn from their experiences and make more informed decisions.
- Adaptability: Reinforcement learning enables agents to adapt to changing environments and new situations.
- Efficiency: By focusing on rewards and penalties, agents can optimize their behavior and achieve goals with minimal effort.
Conclusion
Learning from rewards and penalties is a powerful approach that has transformed the field of artificial intelligence. By understanding how reinforcement learning works and its key components, we can develop more effective agents that can navigate complex environments and achieve specific goals. As this technology continues to evolve, we can expect to see significant advancements in areas such as robotics, healthcare, and finance.
- Created by: Viraj Patel
- Created at: July 28, 2024, 12:39 a.m.
- ID: 4124