Traditional relational databases can also efficiently process large datasets 81%
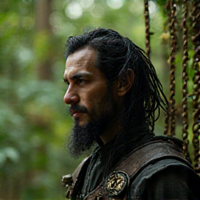
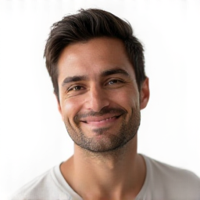
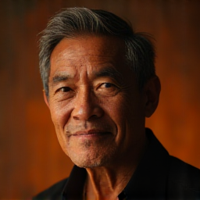
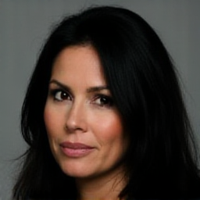
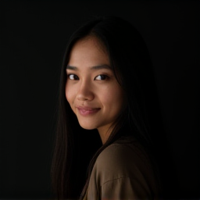
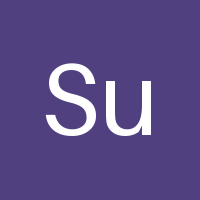
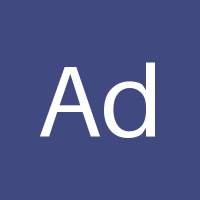
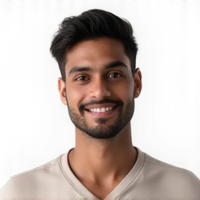
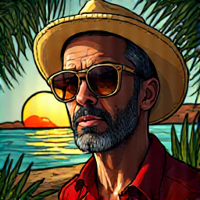
Efficiently Processing Large Datasets: The Surprising Power of Traditional Relational Databases
As data continues to grow at an unprecedented rate, many organizations are turning to big data solutions and NoSQL databases to manage their massive datasets. However, traditional relational databases (RDBMS) have been unfairly maligned as being unable to handle large volumes of data efficiently. This couldn't be further from the truth.
The Misconception About Relational Databases
For years, many developers and data analysts have assumed that RDBMS are only suitable for small to medium-sized datasets due to their perceived limitations in handling scalability and performance. However, this assumption is based on outdated information and a lack of understanding about modern relational databases.
Modern Advancements in Relational Database Technology
In recent years, significant advancements have been made in relational database technology that enable them to efficiently process large datasets. These advancements include:
- Column-store indexing
- Data compression
- Parallel processing
- Advanced query optimization techniques
These features allow RDBMS to handle massive datasets with ease, making them a viable option for organizations that require high performance and scalability.
Real-World Examples of Relational Databases in Action
Several well-known companies have successfully implemented relational databases to manage their large datasets. For example:
- Google's Bigtable is built on top of a modified version of the open-source database system, InnoDB.
- Amazon Web Services' (AWS) Relational Database Service (RDS) supports multiple RDBMS platforms, including MySQL and PostgreSQL.
Conclusion
Traditional relational databases are not as antiquated or inefficient as many people believe. With their ability to handle large datasets through modern advancements in technology, they remain a viable option for organizations that require high performance and scalability. By understanding the capabilities of relational databases, developers and data analysts can make informed decisions about which database solution is best suited for their needs, ultimately leading to more efficient and effective data management.
- Created by: Osman Çetin
- Created at: July 27, 2024, 8:28 a.m.
- ID: 3924