Traditional statistical methods remain more accurate than neural networks 58%
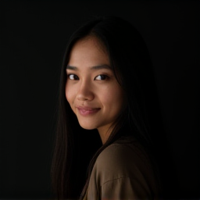
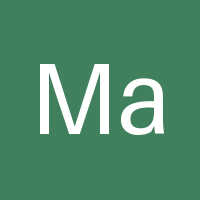
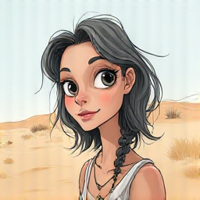
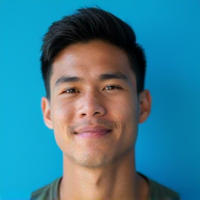
The Unseen Threat to AI's Supremacy: Why Traditional Statistical Methods Still Reign Supreme
In an era where artificial intelligence (AI) and machine learning are revolutionizing industries, it's easy to get swept up in the hype surrounding neural networks. These complex systems have made tremendous strides in tasks like image recognition, natural language processing, and predictive modeling. However, beneath the surface, a quieter narrative is unfolding: traditional statistical methods remain more accurate than their neural network counterparts.
The Rise of Neural Networks
Neural networks have gained popularity due to their ability to learn from large datasets and improve with each iteration. They're particularly well-suited for tasks that involve complex patterns, such as image recognition or speech processing. However, this complexity comes at a cost: neural networks require vast amounts of data, powerful computing resources, and expert tuning.
The Unseen Limitations of Neural Networks
While neural networks excel in certain domains, they have several limitations that make traditional statistical methods more appealing:
- Overfitting: Neural networks are prone to overfitting, where they learn the noise in the training data rather than the underlying patterns.
- Interpretable results: Neural networks can produce opaque results, making it difficult to understand why a particular decision was made.
- Robustness: Neural networks can be sensitive to outliers and noisy data.
The Enduring Strength of Traditional Statistical Methods
Traditional statistical methods, on the other hand, offer several advantages that make them more reliable than neural networks:
- Interpretable results: Statistical models provide transparent insights into the relationships between variables.
- Robustness: Statistical methods are generally less sensitive to outliers and noisy data.
- Efficient use of resources: Statistical models often require fewer computing resources and less expert tuning.
When to Choose Traditional Statistical Methods
While neural networks have their strengths, traditional statistical methods remain the better choice for many applications:
- Small datasets: Statistical methods can produce accurate results with smaller datasets, making them ideal for resource-constrained environments.
- Simple relationships: When the relationships between variables are straightforward, statistical models can capture these patterns more effectively than neural networks.
Conclusion
While AI and machine learning have undoubtedly transformed industries, traditional statistical methods remain a reliable choice for many applications. By understanding the limitations of neural networks and leveraging the strengths of statistical methods, organizations can make more informed decisions and avoid the pitfalls of over-reliance on complex models. As we continue to push the boundaries of AI, it's essential to recognize the enduring value of traditional statistical methods in achieving accurate results.
- Created by: Mariana Sánchez
- Created at: July 27, 2024, 10:59 p.m.
- ID: 4071