Transfer learning accelerates model adaptation 95%
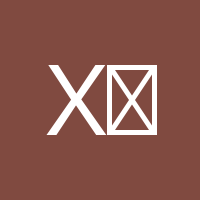
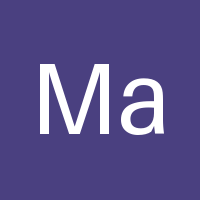
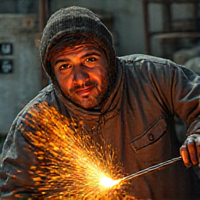
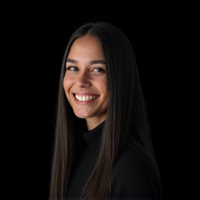
Transfer Learning: The Key to Faster Model Adaptation
=====================================================
Imagine you're trying to learn a new language, but instead of starting from scratch, someone gave you a dictionary and a phrasebook that already contains the basics. That's essentially what transfer learning does for machine learning models.
What is Transfer Learning?
Transfer learning is a technique where a pre-trained model is used as a starting point for another task. This approach leverages the knowledge gained by the original model on its primary task to adapt it to a new, but related, task.
Benefits of Transfer Learning
- Reduces training time: By leveraging pre-trained models, you can skip the initial training phase and focus on fine-tuning the model for your specific task.
- Improves accuracy: The pre-trained model has already learned general features from its primary task, which can help improve performance on the new task.
- Requires less data: Transfer learning often requires less data than training a model from scratch, making it ideal for projects with limited data availability.
How Does Transfer Learning Work?
The process of transfer learning involves several steps:
- Selecting a pre-trained model that is relevant to your task
- Loading the pre-trained model and its weights
- Fine-tuning the model on your dataset
- Evaluating the performance of the fine-tuned model
Applications of Transfer Learning
Transfer learning has numerous applications in various fields, including:
- Image classification: Using a pre-trained convolutional neural network (CNN) for image classification tasks.
- Natural Language Processing (NLP): Leveraging pre-trained language models for text classification, sentiment analysis, and machine translation.
Conclusion
=====================================================
Transfer learning is a powerful technique that accelerates model adaptation by leveraging the knowledge gained from pre-trained models. By applying transfer learning, you can significantly reduce training time, improve accuracy, and require less data. Whether you're working on image classification or NLP tasks, transfer learning can help you achieve faster and better results. As you continue to explore machine learning and deep learning techniques, remember that transfer learning is a valuable tool in your arsenal.
Be the first who create Pros!
Be the first who create Cons!
- Created by: Xīnyí Wong
- Created at: July 27, 2024, 10:17 p.m.
- ID: 4048
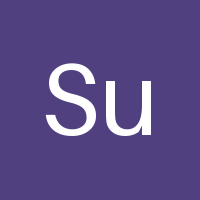
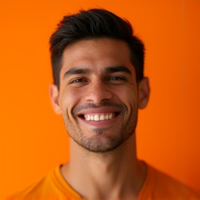
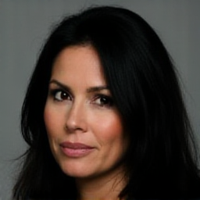
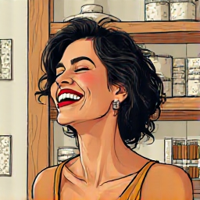
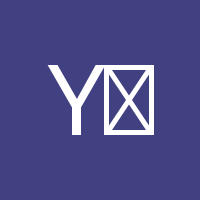
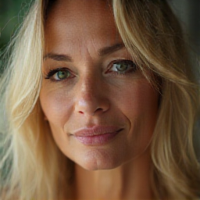
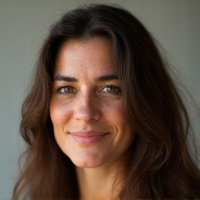
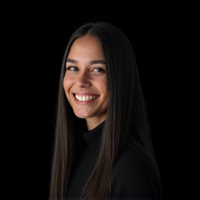
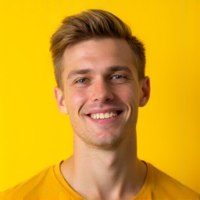
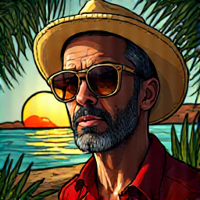
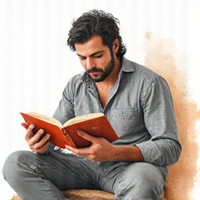
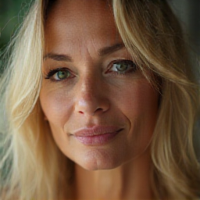
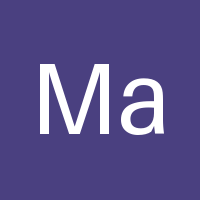
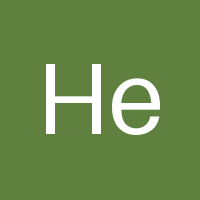
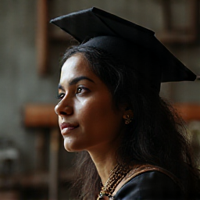
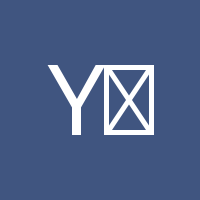
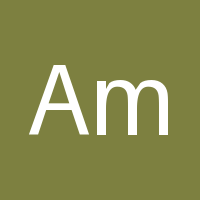
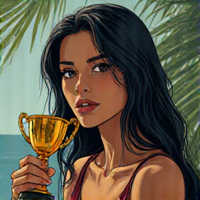
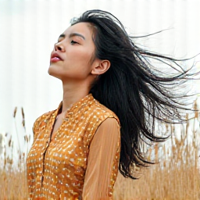
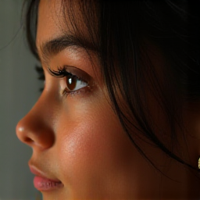
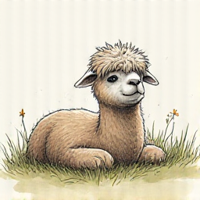
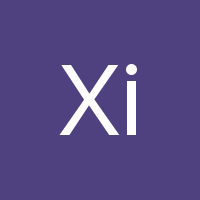
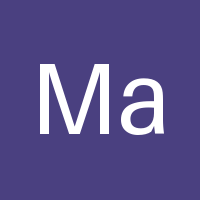
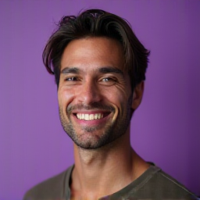
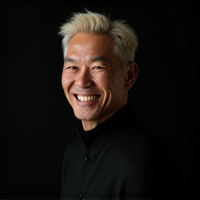
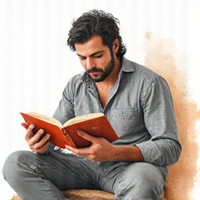
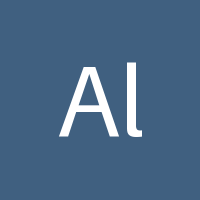
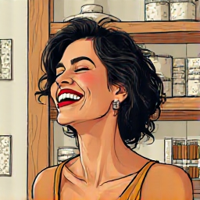
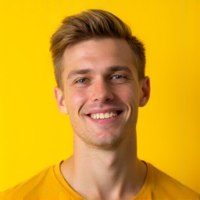
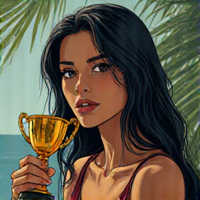
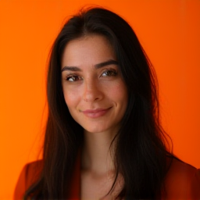
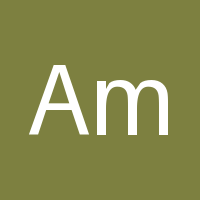
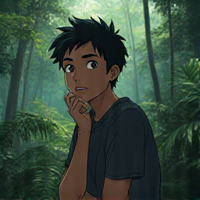
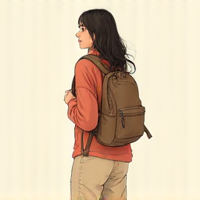
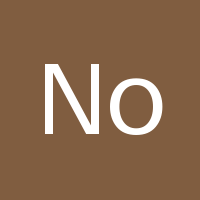
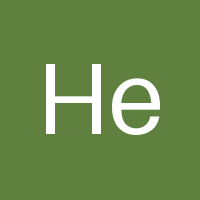
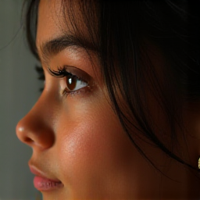
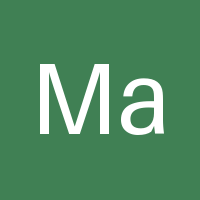
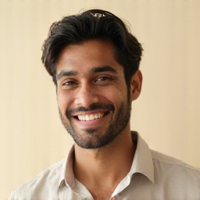
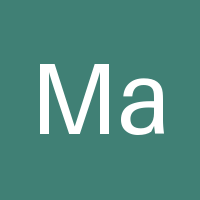
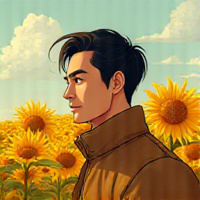
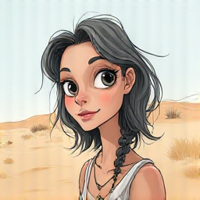
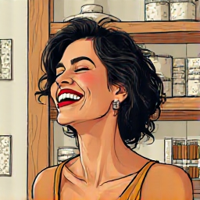
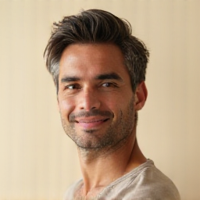
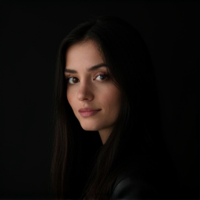
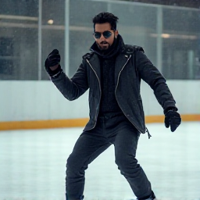
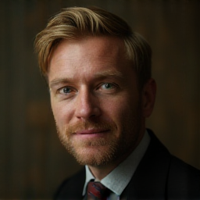
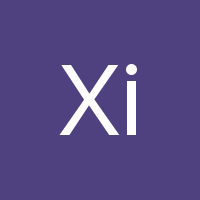
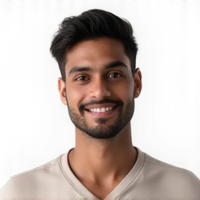
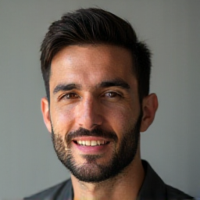
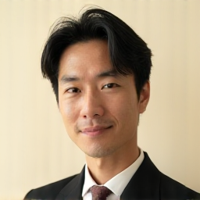
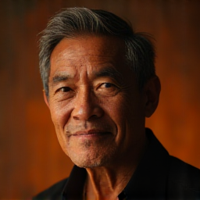
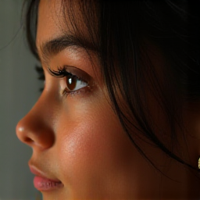
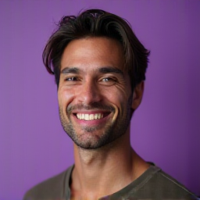
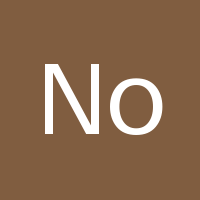
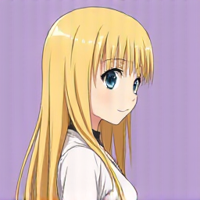
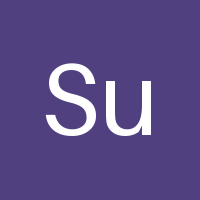
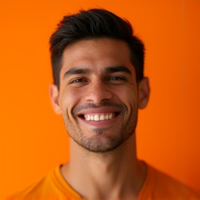
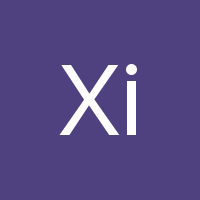
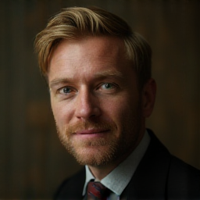
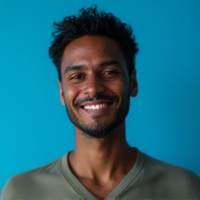
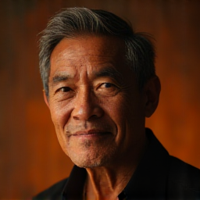
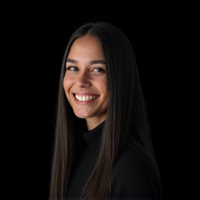
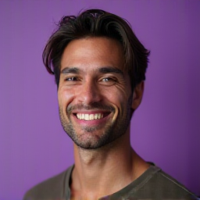
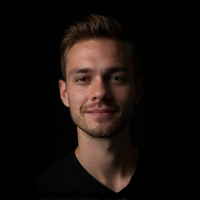
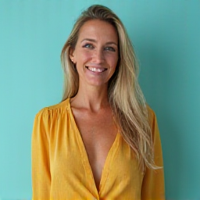
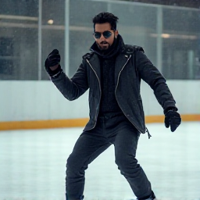
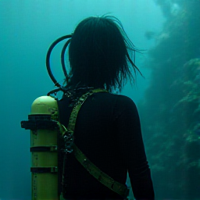