Transfer learning leverages previously trained models without supervision 73%
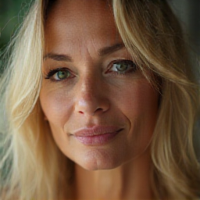
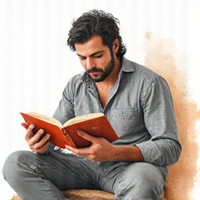
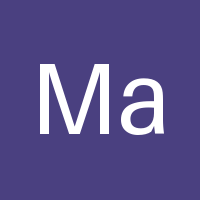
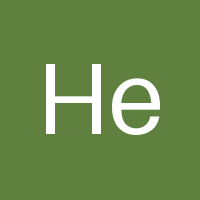
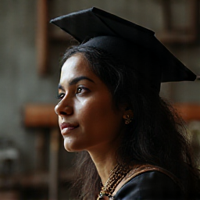
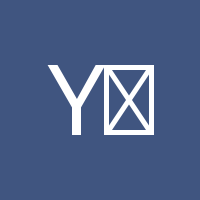
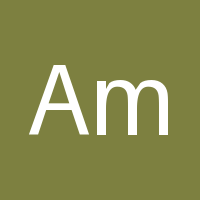
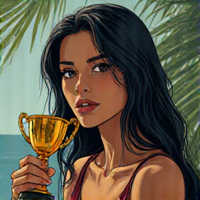
The Power of Transfer Learning: Unlocking Value from Pre-Trained Models
Imagine having access to a treasure trove of pre-trained models, each one honed on a specific task or dataset, and being able to leverage that knowledge without the need for extensive retraining. Sounds too good to be true? Think again! This is precisely what transfer learning offers – the ability to tap into previously trained models and apply their learned features to new tasks with minimal supervision.
What is Transfer Learning?
Transfer learning is a machine learning technique that enables us to use pre-trained models as a starting point for our own task, rather than training from scratch. By leveraging the knowledge gained from previous experiences, we can significantly reduce the amount of time and resources required to train a model on our specific problem.
Benefits of Transfer Learning
Transfer learning offers numerous benefits over traditional machine learning approaches:
- Reduced training time: Since the pre-trained model has already learned features from a related task or dataset, we don't have to spend as much time fine-tuning it.
- Improved accuracy: Pre-trained models often achieve high accuracy on their original tasks and can provide a solid foundation for new tasks.
- Increased efficiency: By leveraging existing knowledge, we can focus on the nuances of our specific problem rather than starting from scratch.
Applications of Transfer Learning
Transfer learning has numerous real-world applications across various industries:
- Image classification: Pre-trained models like VGG16 or ResNet50 are often fine-tuned for image classification tasks such as object detection or facial recognition.
- Natural language processing: Models trained on large text datasets can be adapted for sentiment analysis, named entity recognition, or machine translation.
- Time series forecasting: Knowledge gained from pre-trained models can be applied to predict stock prices, weather patterns, or energy consumption.
Challenges and Limitations
While transfer learning is incredibly powerful, there are potential challenges and limitations:
- Choosing the right pre-trained model: Selecting a model that aligns with our task and dataset is crucial for successful transfer.
- Adapting to new data distributions: Pre-trained models may not always generalize well to new or unseen data.
Conclusion
Transfer learning has revolutionized the field of machine learning by providing an efficient way to leverage previously trained models without extensive supervision. By understanding its benefits, applications, and challenges, we can unlock its full potential and accelerate our progress toward solving complex problems. Whether you're a seasoned researcher or a beginner in AI, transfer learning is definitely worth exploring further – it has the power to transform your projects and catapult your career forward!
- Created by: Pari Singh
- Created at: July 27, 2024, 11:49 p.m.
- ID: 4097