Unstructured data requires careful NLP management 86%
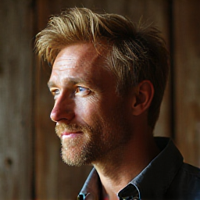
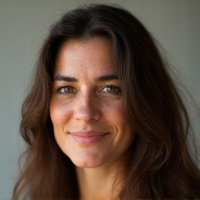
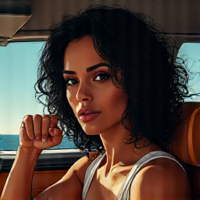
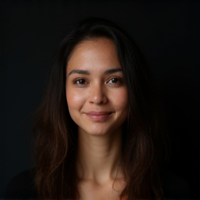
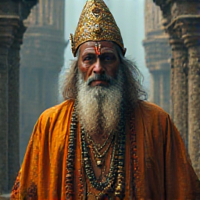
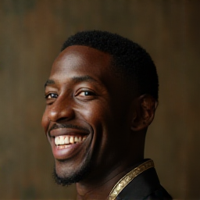
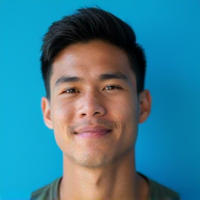
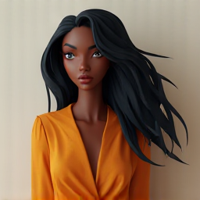
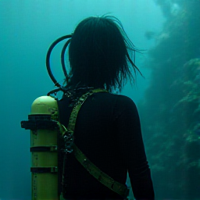
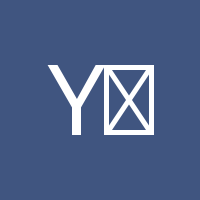
Unstructured Data: The Unseen Challenge for NLP Management
In today's data-driven world, organizations are generating vast amounts of unstructured data from various sources such as social media, emails, and text documents. This data is a goldmine of insights waiting to be tapped, but it poses significant challenges for Natural Language Processing (NLP) management.
The Complexity of Unstructured Data
Unstructured data lacks a predefined format, making it difficult to analyze and process using traditional methods. It can take many forms, including text, images, audio, and video files. This diversity makes it challenging to develop effective NLP solutions that can accurately capture the nuances and context of human language.
The Role of NLP in Unstructured Data Management
NLP plays a crucial role in unstructured data management by enabling machines to understand and interpret human language. It involves various tasks such as text classification, sentiment analysis, entity recognition, and topic modeling. However, the success of NLP depends on careful management of unstructured data.
Challenges in NLP Management for Unstructured Data
- Lack of standardization: Unstructured data often lacks a common format or structure, making it difficult to process and analyze.
- Limited contextual understanding: NLP models struggle to capture the nuances and context of human language, leading to inaccurate results.
- High dimensionality: Unstructured data can be high-dimensional, requiring sophisticated algorithms and computational resources to process.
- Data quality issues: Unstructured data often contains noise, inconsistencies, and inaccuracies that can affect the reliability of NLP outputs.
Effective Strategies for NLP Management
To overcome these challenges, organizations need to adopt effective strategies for NLP management. These include:
- Data Preprocessing: Cleaning, tokenization, and normalization of unstructured data are essential steps in preparing it for analysis.
- Model Selection: Choosing the right NLP model depends on the specific task and dataset characteristics.
- Hyperparameter Tuning: Adjusting hyperparameters to optimize model performance is crucial for accurate results.
- Continuous Monitoring: Regularly evaluating and updating NLP models to adapt to changing data distributions and requirements.
Conclusion
Unstructured data presents a significant challenge for NLP management, but careful consideration of these challenges can lead to effective solutions. By adopting strategies such as data preprocessing, model selection, hyperparameter tuning, and continuous monitoring, organizations can unlock the value hidden in unstructured data and make informed decisions. In today's data-driven world, investing in NLP management is no longer a luxury, but a necessity for staying ahead of the competition.
- Created by: John Jackson
- Created at: July 27, 2024, 6:15 a.m.
- ID: 3848