Unsupervised machine learning algorithms detect anomalies in datasets 84%
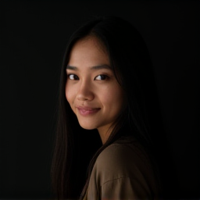
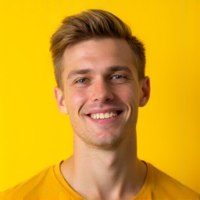
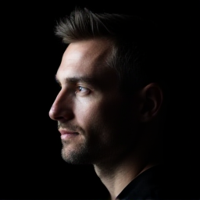
Uncovering Hidden Patterns: How Unsupervised Machine Learning Algorithms Detect Anomalies in Datasets
As data continues to grow at an unprecedented rate, the importance of identifying anomalies and outliers within datasets has become increasingly crucial. In today's big data landscape, being able to detect even the slightest irregularities can make all the difference between making informed decisions and missing out on valuable insights.
The Problem with Traditional Supervised Learning
Traditional supervised learning algorithms rely heavily on labeled training data to learn from. However, this approach comes with its own set of limitations, including:
- High cost of labeling large datasets
- Limited availability of high-quality labeled data
- Difficulty in scaling up to handle complex and diverse datasets
The Power of Unsupervised Machine Learning
Unsupervised machine learning algorithms, on the other hand, don't require any prior knowledge or labeled data. They can learn from patterns and relationships within a dataset, making them particularly effective for anomaly detection.
Types of Unsupervised Anomaly Detection Algorithms
There are several types of unsupervised anomaly detection algorithms, each with its own strengths and weaknesses:
Density-Based Methods
These methods work by identifying clusters within the data based on density. Points that fall outside these clusters are considered anomalies.
- DBSCAN (Density-Based Spatial Clustering of Applications with Noise)
- OPTICS (Ordering Points To Identify the Clustering Structure)
Statistical Methods
These methods rely on statistical models to identify outliers and anomalies. They're often used in conjunction with other methods to improve accuracy.
- Z-score
- Mahalanobis distance
Machine Learning-Based Methods
These methods use machine learning algorithms to learn from patterns within the data. They can be highly effective for anomaly detection, especially when combined with other techniques.
- Isolation Forest
- Local Outlier Factor (LOF)
How Unsupervised Anomaly Detection Algorithms Work
Unsupervised anomaly detection algorithms typically follow a similar process:
- Data preprocessing: Cleaning and transforming the data to prepare it for analysis.
- Feature engineering: Extracting relevant features from the data that can help identify anomalies.
- Algorithm selection: Choosing an unsupervised anomaly detection algorithm based on the characteristics of the dataset and the type of anomalies being targeted.
- Model training: Training the selected algorithm using a subset of the data.
- Anomaly scoring: Applying the trained model to the entire dataset to generate anomaly scores for each point.
Conclusion
Unsupervised machine learning algorithms offer a powerful solution for detecting anomalies in datasets, even when there's no prior knowledge or labeled data available. By leveraging density-based methods, statistical models, and machine learning techniques, we can uncover hidden patterns and insights that might have gone unnoticed otherwise. As the importance of anomaly detection continues to grow, it's essential to understand the capabilities and limitations of these algorithms and how they can be applied in real-world scenarios.
- Created by: MikoĊaj Krawczyk
- Created at: July 28, 2024, 12:01 a.m.
- ID: 4103