Veracity of big data sources is often uncertain 68%
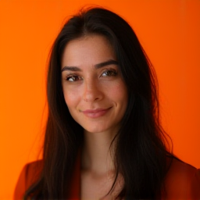
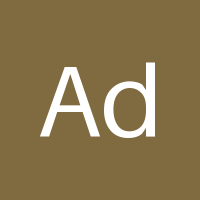
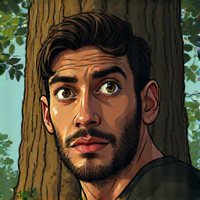
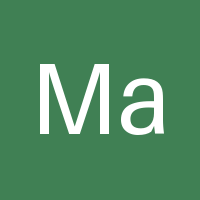
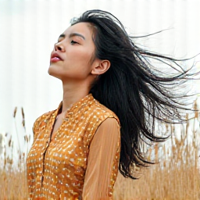
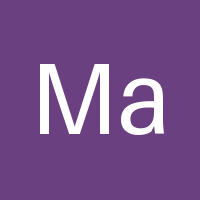
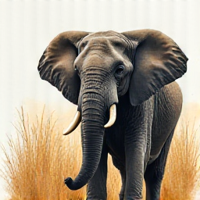
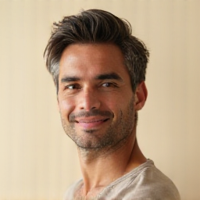
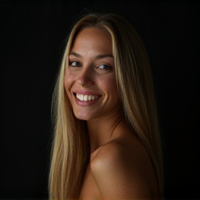
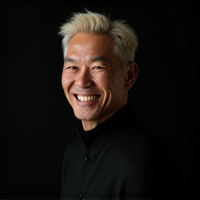
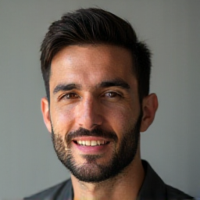
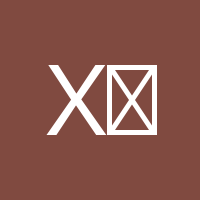
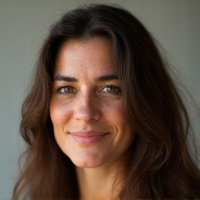
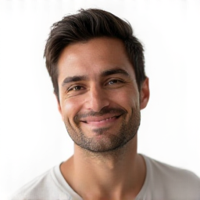
The Uncertainty Epidemic: Uncovering the Veracity of Big Data Sources
In today's data-driven world, the phrase "big data" is often used to describe the vast amounts of information collected from various sources. However, beneath this sea of data lies a critical issue that can have far-reaching consequences: the uncertainty of its veracity.
The Origins of Uncertainty
Big data sources are inherently diverse and come from a variety of places, including social media platforms, online transactions, sensors, and more. While these sources can provide valuable insights into consumer behavior, market trends, and operational efficiencies, they also introduce opportunities for errors, biases, and inaccuracies.
The Sources of Inaccuracy
The following list highlights some common sources of inaccuracy in big data:
- Inconsistent or missing metadata
- Biased sampling methods
- Incorrect data normalization
- Outdated or irrelevant data
- Data duplication and redundancy
The Consequences of Uncertainty
The uncertainty surrounding the veracity of big data sources can have significant consequences, including:
- Poor decision-making: If data is inaccurate or incomplete, it can lead to poor decisions that may harm a business or organization.
- Loss of trust: When stakeholders discover that their data has been compromised or manipulated, they may lose faith in the organization's ability to manage and protect their information.
- Regulatory issues: Failing to maintain accurate records can result in non-compliance with regulatory requirements, leading to fines and reputational damage.
The Solution: Verification and Validation
To mitigate these risks, organizations must prioritize verification and validation of big data sources. This involves:
- Conducting regular audits to identify and address errors or inconsistencies
- Implementing quality control measures to ensure data accuracy and completeness
- Developing and enforcing robust data governance policies
- Providing transparent and accessible documentation for stakeholders
Conclusion
The uncertainty surrounding the veracity of big data sources is a pressing issue that requires immediate attention. By understanding the origins of uncertainty, identifying common sources of inaccuracy, and implementing verification and validation strategies, organizations can build trust with their stakeholders and make informed decisions based on accurate information. In today's data-driven world, this is not just a nicety – it's a necessity.
- Created by: Yìzé Ko
- Created at: July 27, 2024, 3:16 a.m.
- ID: 3735