Weighted averages ignore complex input relationships 67%
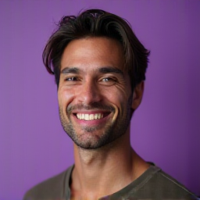
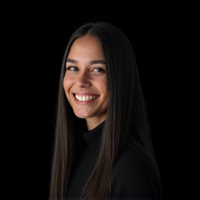
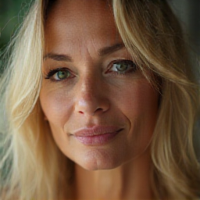
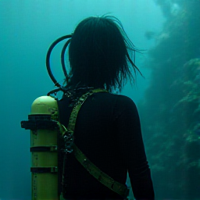
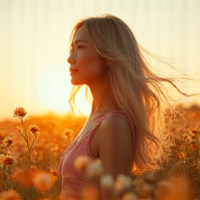
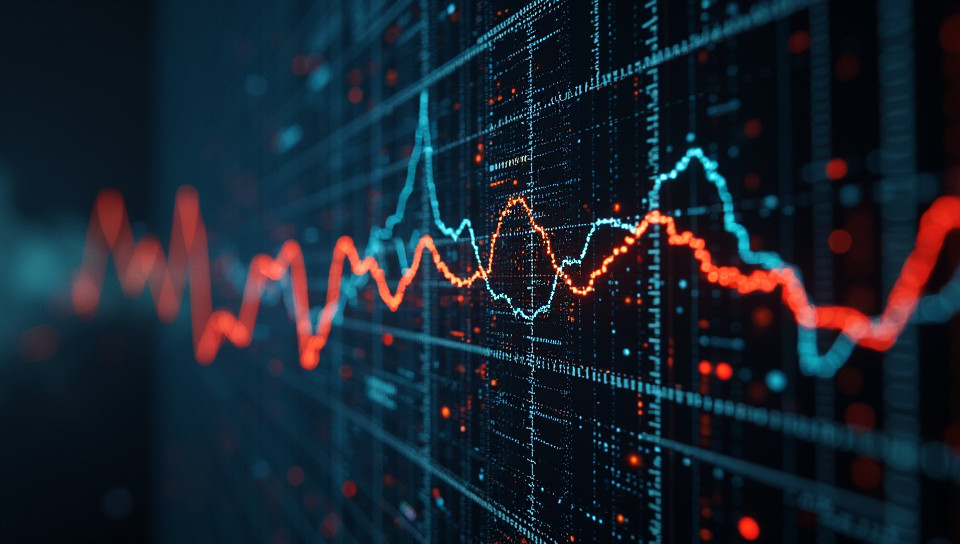
The Hidden Dangers of Weighted Averages
When working with complex data sets, one of the most common tools we reach for is the weighted average. It's a simple yet powerful statistic that can give us valuable insights into our data. However, there's a catch – weighted averages often ignore complex input relationships, which can lead to misleading conclusions.
What are Weighted Averages?
Weighted averages are a way of calculating an overall value based on multiple inputs, each with its own weight or importance. The weights determine how much influence each input has on the final result. For example, if we're evaluating employee performance, their sales numbers might be weighted at 30%, while their customer satisfaction ratings are weighted at 20%. The remaining 50% could be based on other factors like teamwork and adaptability.
When Do Weighted Averages Fall Short?
Weighted averages can fall short when the inputs have complex relationships with each other. Here's an example: Let's say we're evaluating a new product launch, and we've calculated its weighted average score across various metrics such as customer satisfaction (30%), sales growth (25%), and market share (45%). However, what if these metrics are interconnected in ways that can't be captured by simple weights? For instance:
- Customer satisfaction is affected by the quality of our marketing campaigns.
- Sales growth is influenced by the price point we set for the product.
- Market share is impacted by our distribution channels and competition.
Ignoring Complex Relationships
When we use weighted averages without considering these complex relationships, we risk ignoring crucial interactions between inputs. This can lead to misleading conclusions or inaccurate predictions about how well a particular strategy will perform.
The Importance of Considering Interactions
To avoid this pitfall, it's essential to consider the interactions between our input metrics. There are several approaches to doing so:
- Correlation analysis: Identify which inputs are strongly correlated with each other and adjust their weights accordingly.
- Structural equation modeling: Use statistical models to analyze how different inputs affect each other.
- Monte Carlo simulations: Run multiple scenarios to understand how variations in one input can impact others.
Conclusion
Weighted averages can be a powerful tool for simplifying complex data sets, but they're not foolproof. By ignoring the intricate relationships between our input metrics, we risk making decisions based on incomplete information. To get a more accurate picture of our data, it's crucial to consider these interactions and use more sophisticated methods when necessary. Only then can we trust our weighted averages to give us valuable insights that inform our decision-making.
- Created by: Yuina Chiba
- Created at: Nov. 14, 2024, 1:49 p.m.
- ID: 15930